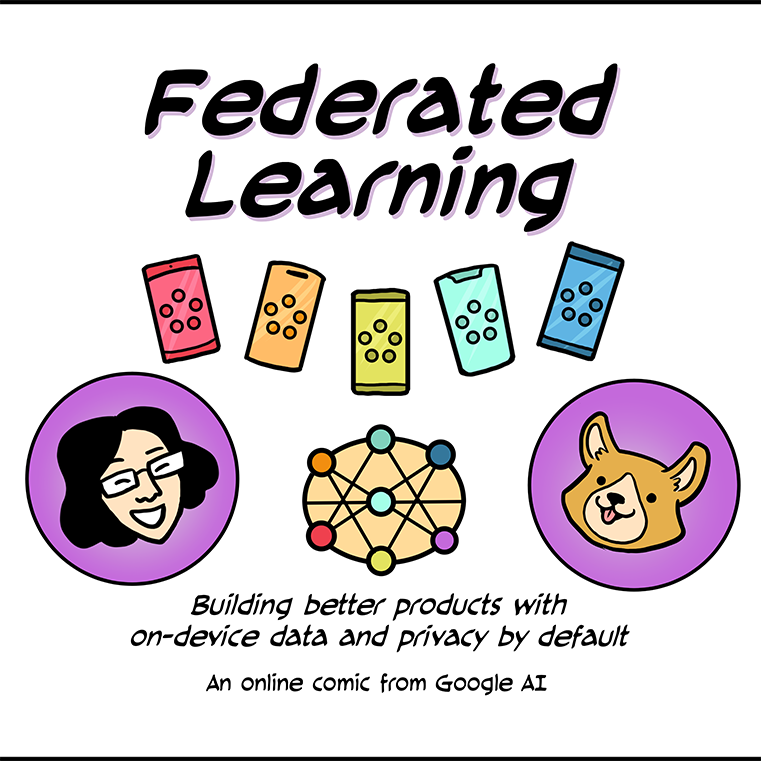
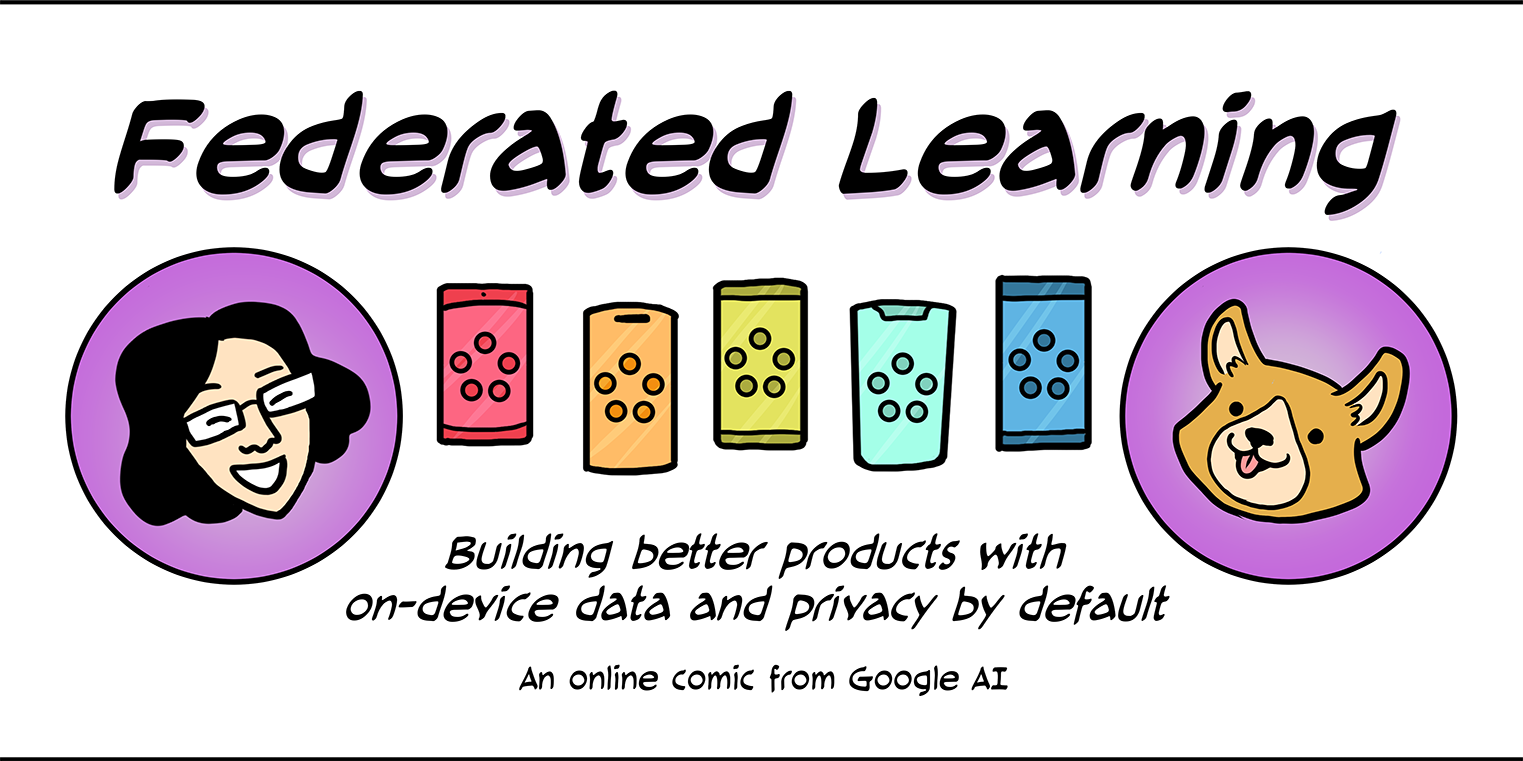
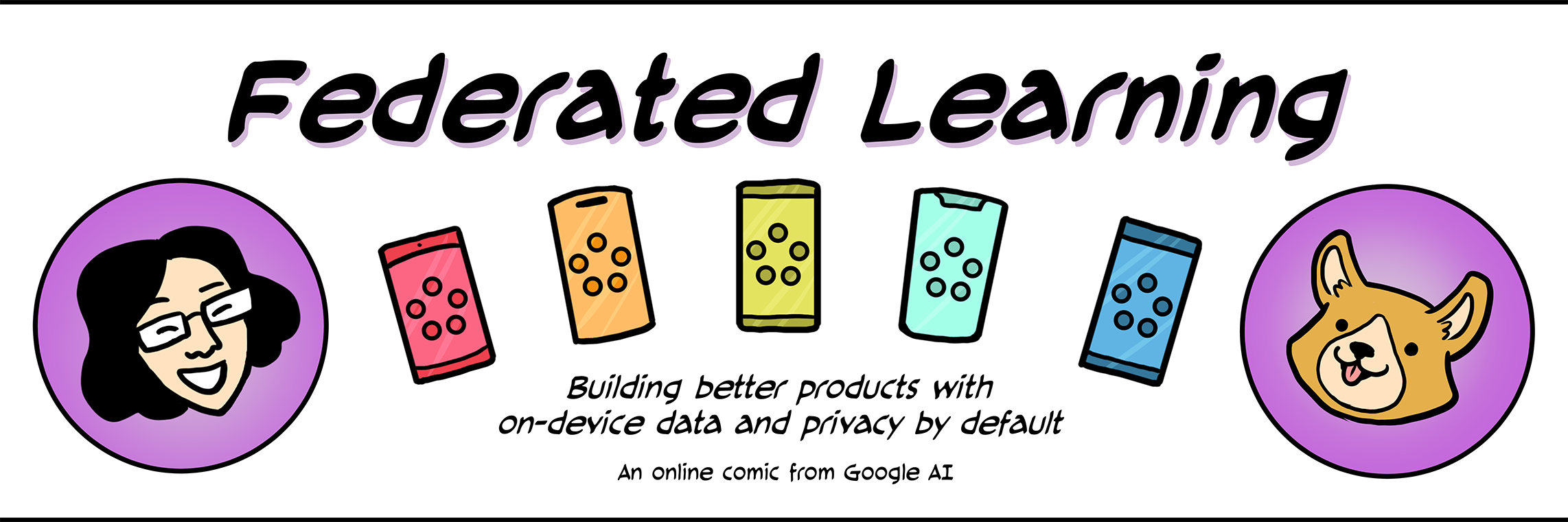
Building better products with on-device data and privacy by default. An online comic from Google AI.
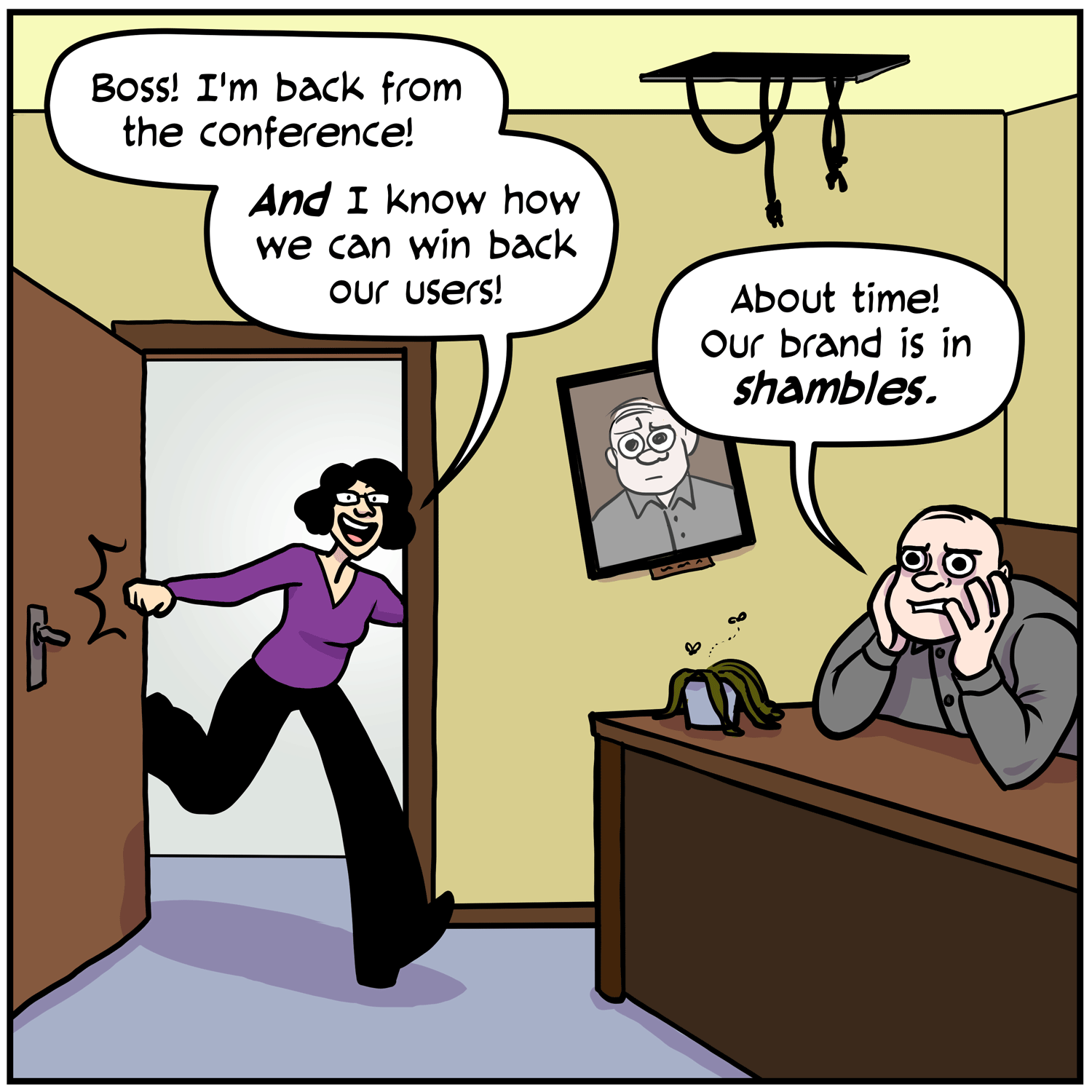
Martha, a caucasian woman in her mid-thirties, bursts into a run-down office. Her Boss, a balding caucasian man in his fifties, sits behind his desk in despair. There’s a dead cactus by his elbow, an anxious-looking photo of him on the wall, and exposed wires hanging from the ceiling. Martha shouts “Boss! I’m back from the conference! And I know how we can win back our users!” “About time!” The Boss says. “Our brand is in shambles.”
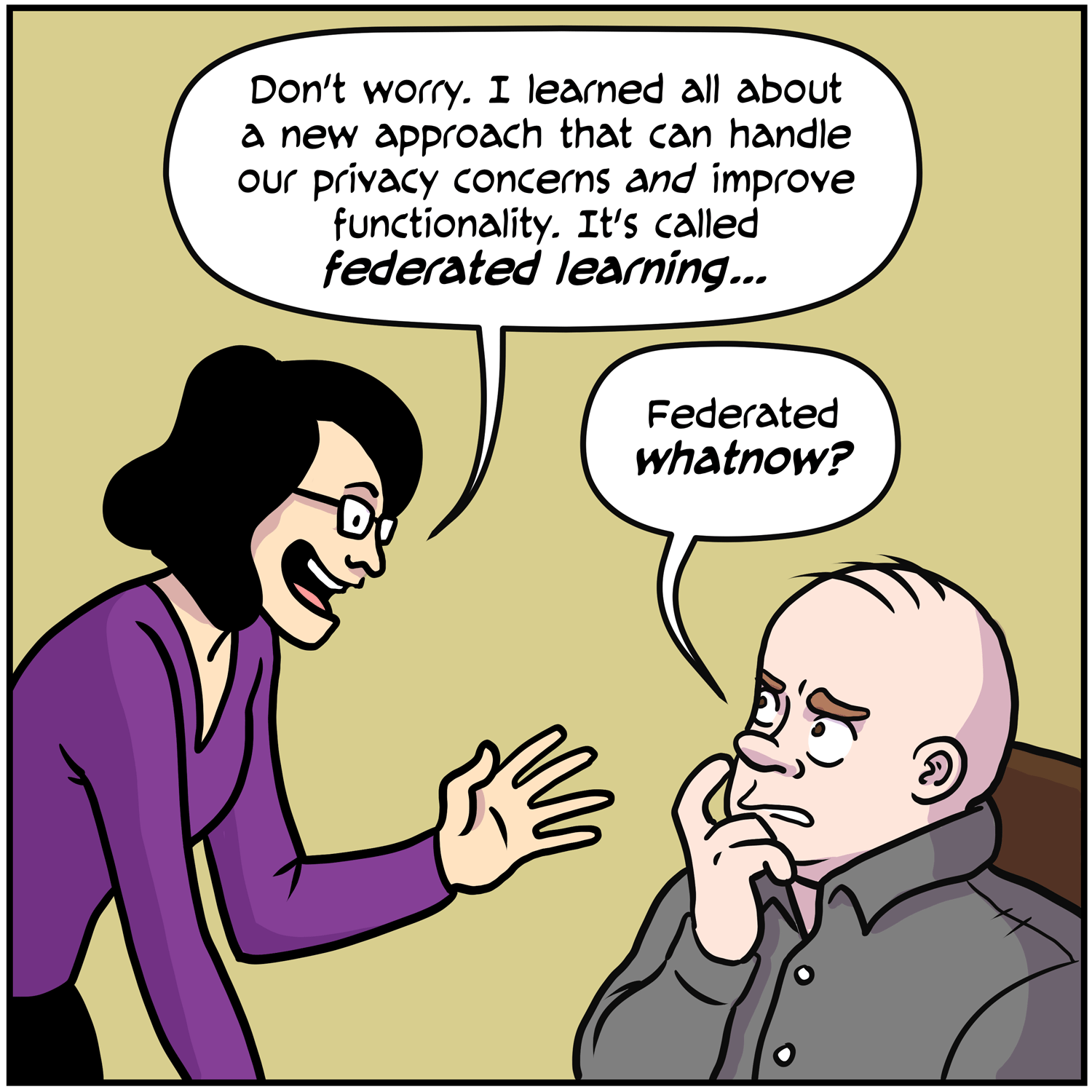
Martha leans eagerly across the desk. “Don’t worry,” she says. “I learned all about a new approach that can handle our privacy concerns and improve functionality. It’s called federated learning…” Her Boss interrupts. “Federated what now?”
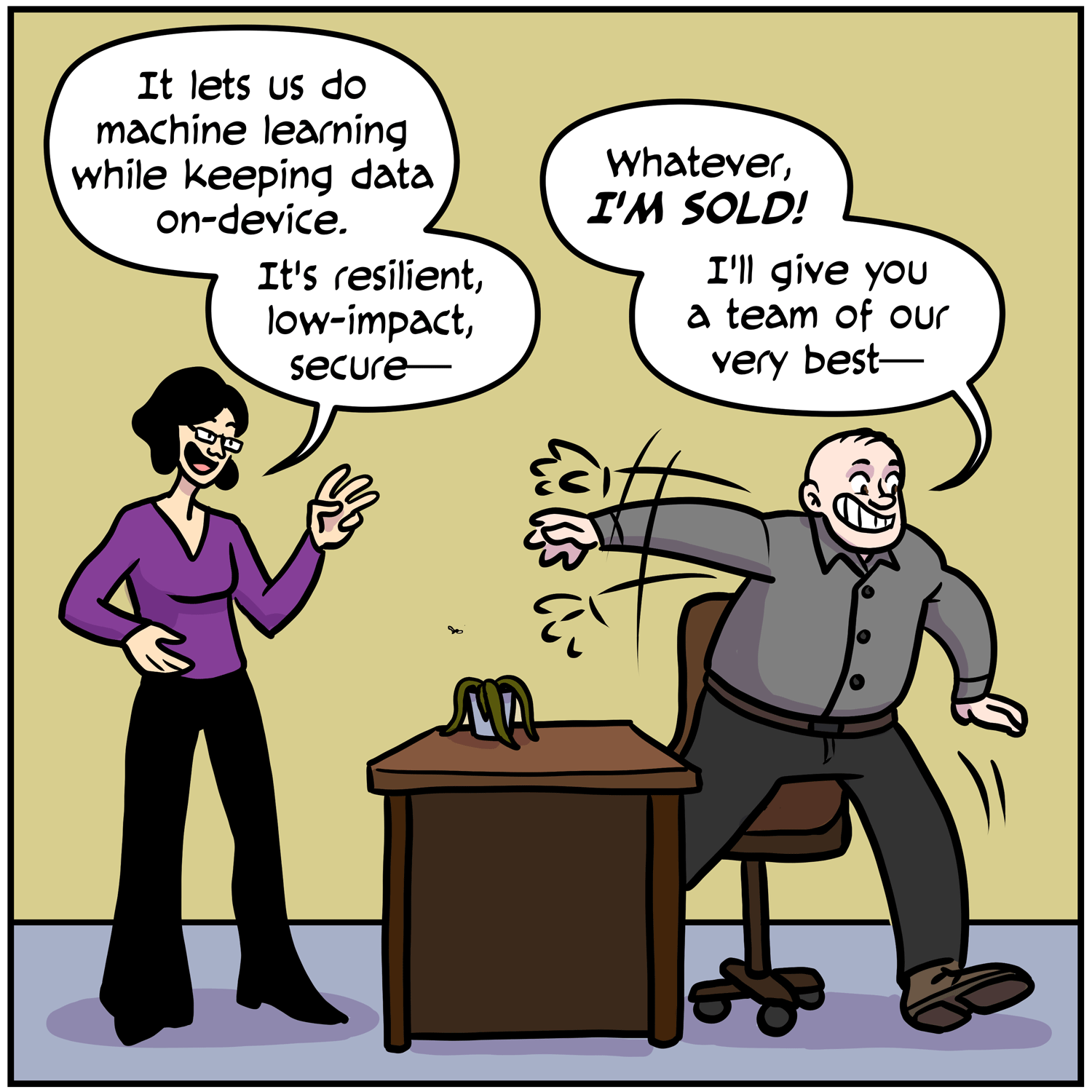
Martha waves her hands in excitement while she talks. “It lets us do machine learning while keeping data on-device. It’s resilient, low-impact, secure–” The Boss leaps out of his chair and hurries off-panel. “Whatever, I’m sold! I’ll give you a team of our very best–”
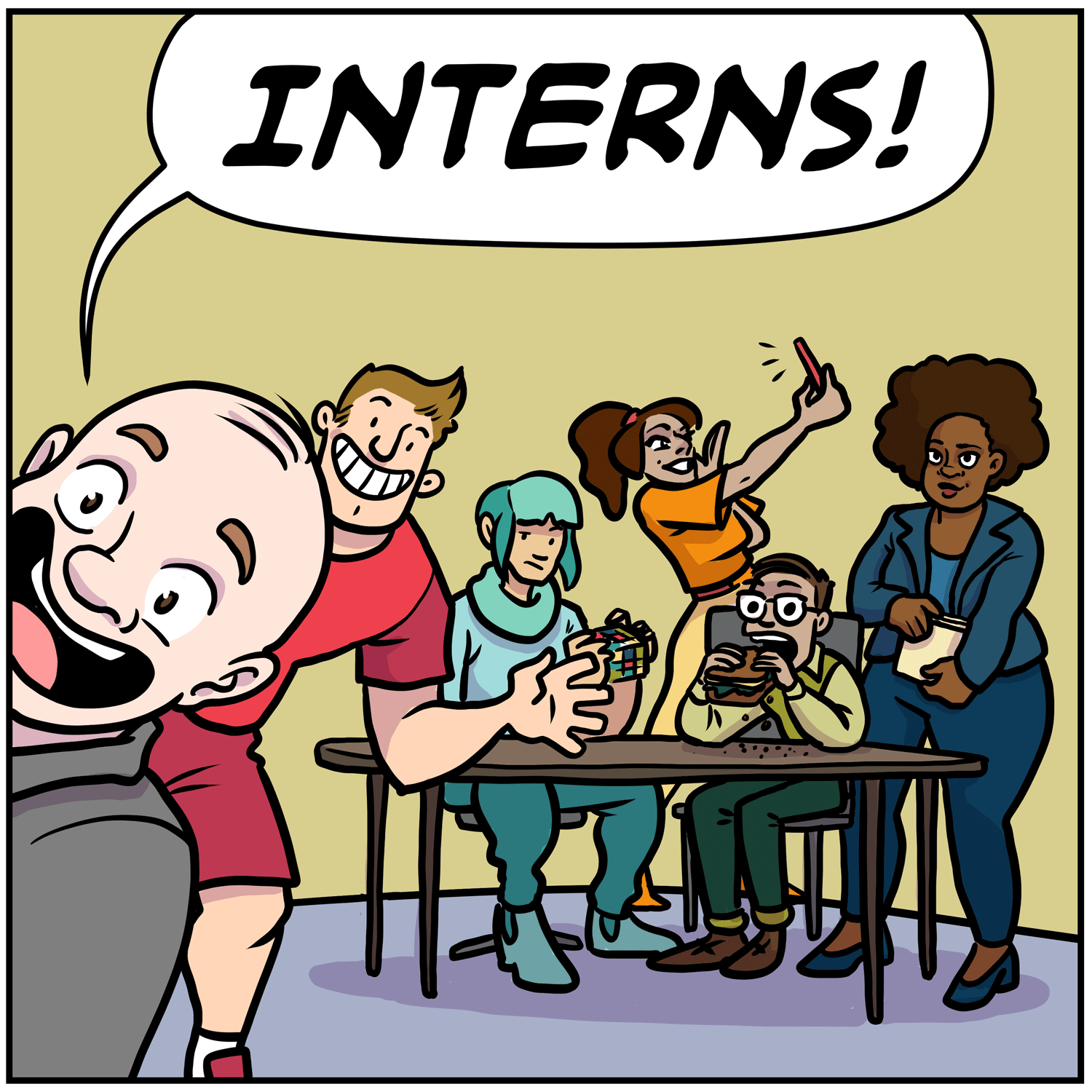
The Boss gestures grandly to a rag-tag group of twenty-somethings while shouting “Interns!” The five interns all look up. Brad, a burly caucasian jock, waves hello overenthusiastically. Kai, a nonbinary Japanese-American hacker, plays with a Rubix cube. Devi, a bubbly Indian-American networker, snaps a selfie. Mateo, a scrawny Hispanic bookworm, pauses in the middle of eating a sandwich. Aliyah, a sharply-dressed African-American security enthusiast, looks unimpressed.
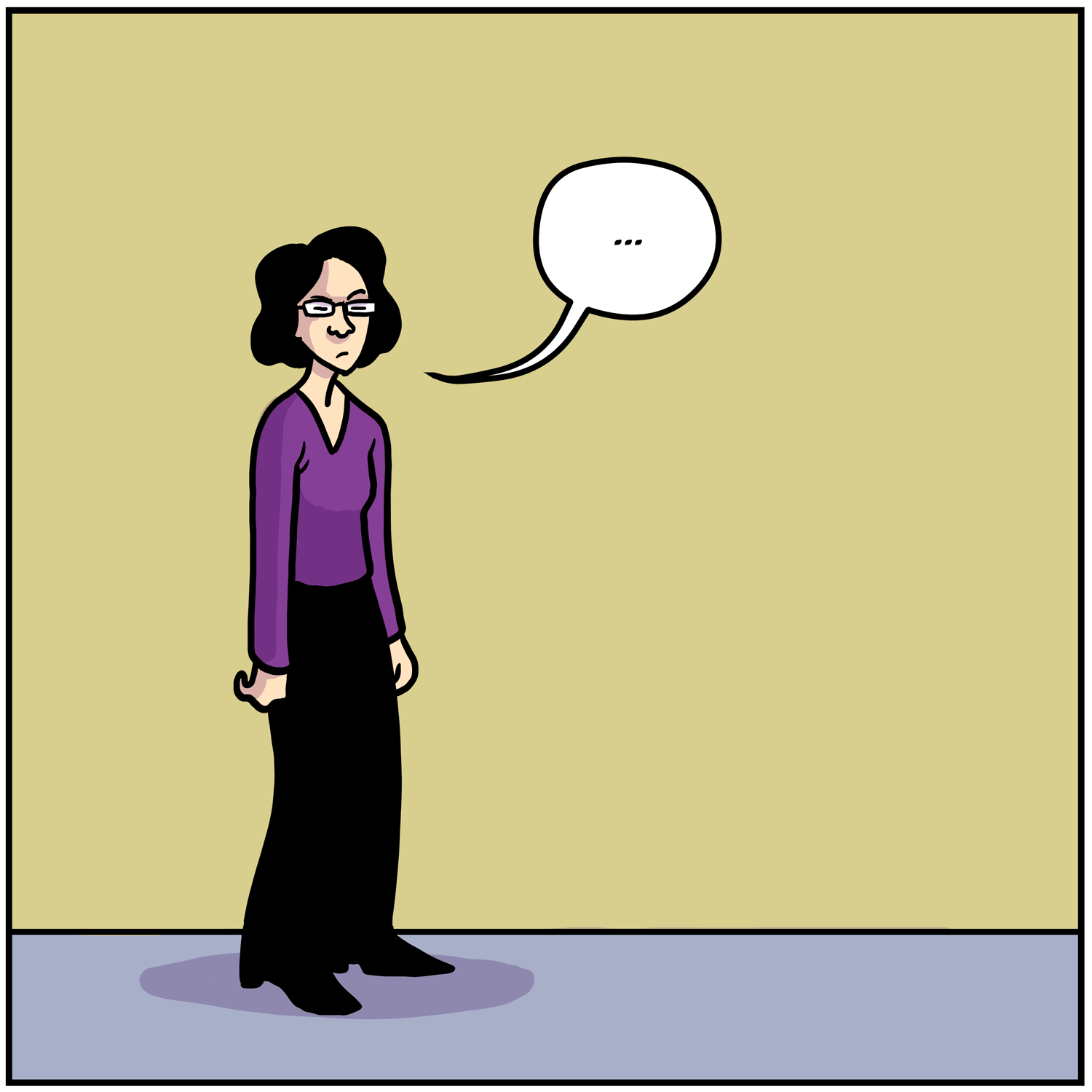
Martha glares at the interns.
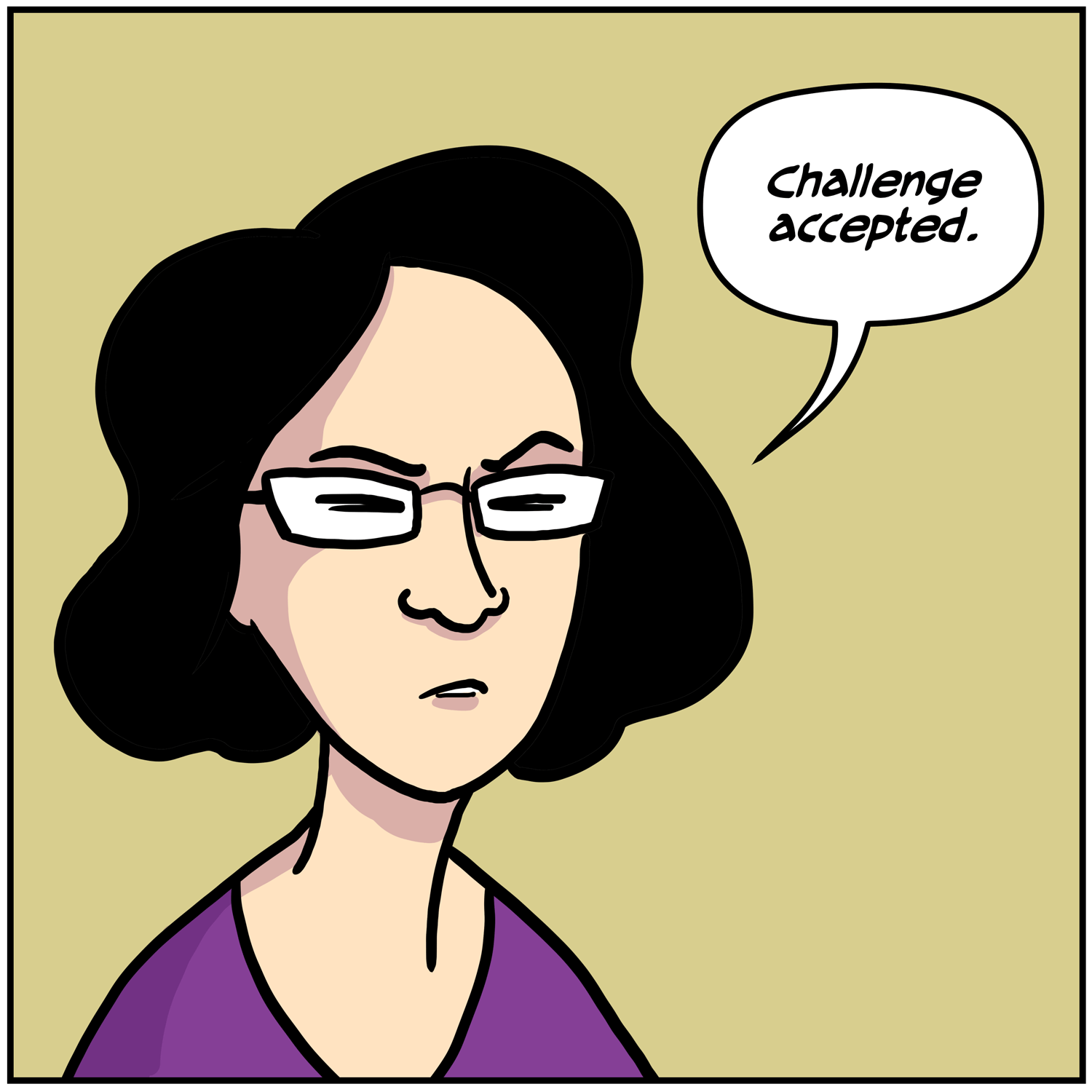
Martha narrows her eyes and says “Challenge accepted.”
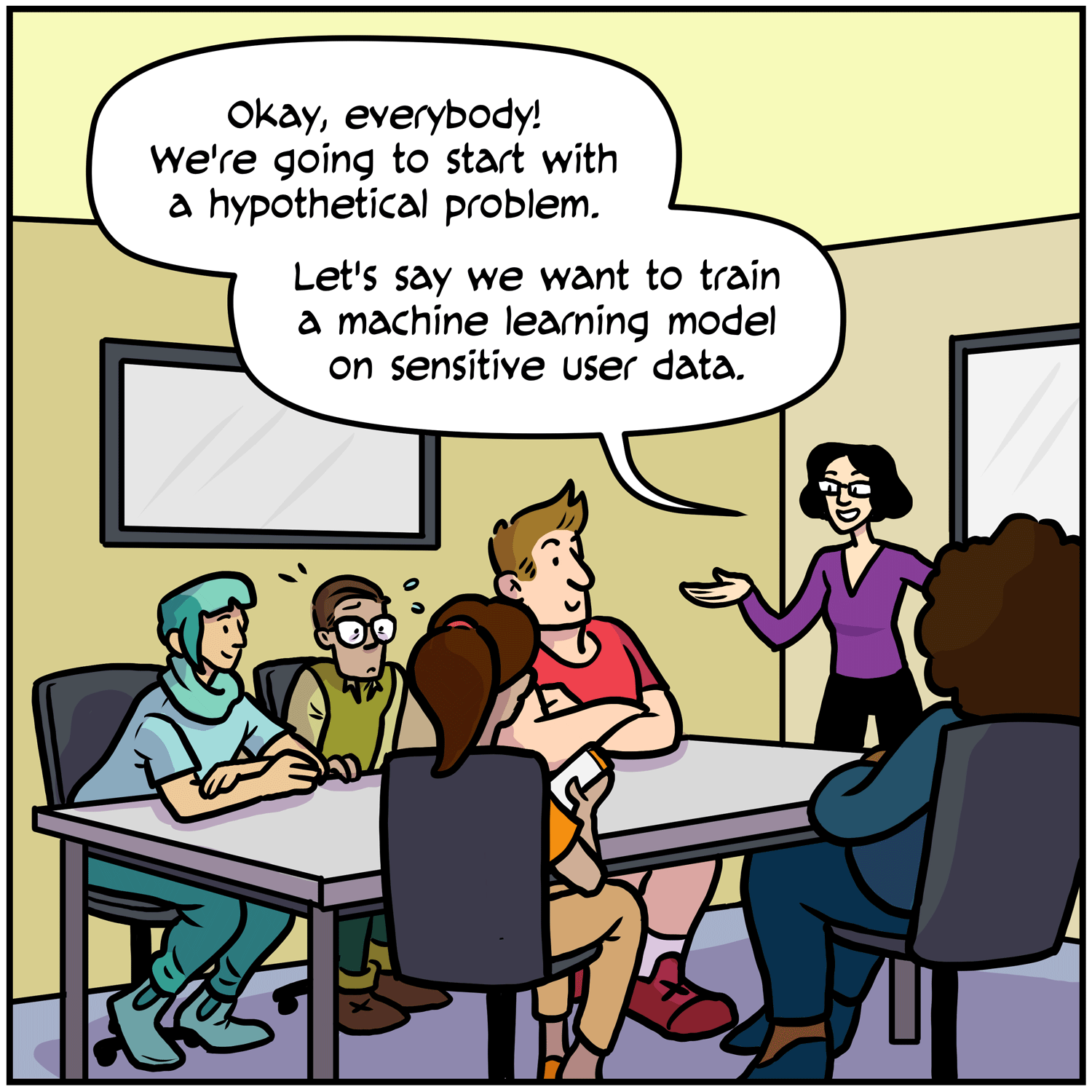
The interns sit around a conference table while Martha presents in front of a white-board. “Okay, everybody!” Martha says. “We’re going to start with a hypothetical problem. Let’s say we want to train a machine learning model on sensitive user data.”
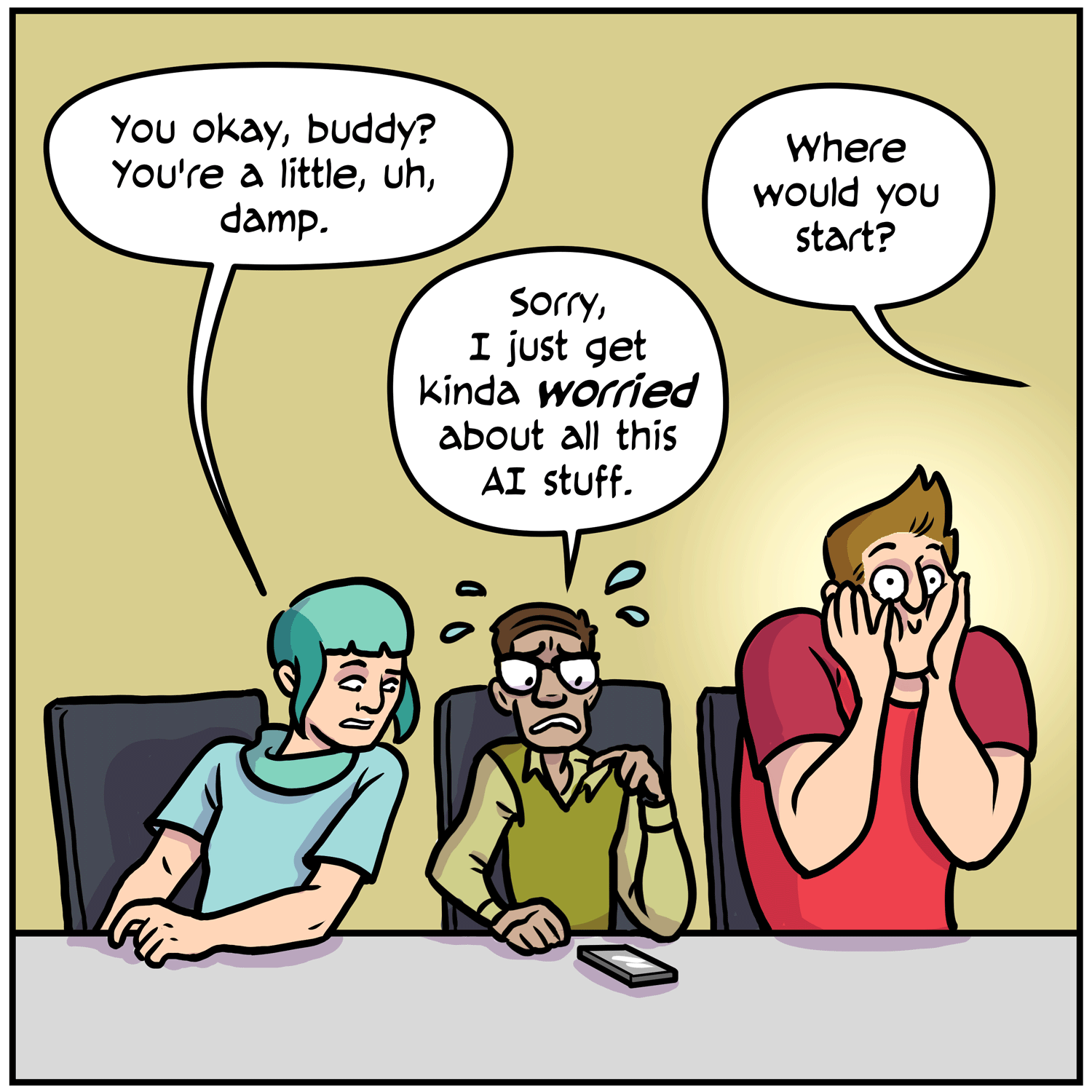
Kai leans over to Mateo, who suddenly looks very sweaty. Kai says “You okay, buddy? You’re a little, uh, damp.” Mateo tugs at his collar and says “Sorry, I just get kinda worried about all this AI stuff.” Brad sits up in his seat eagerly. Martha, speaking from off-panel, says “Where would you start?”
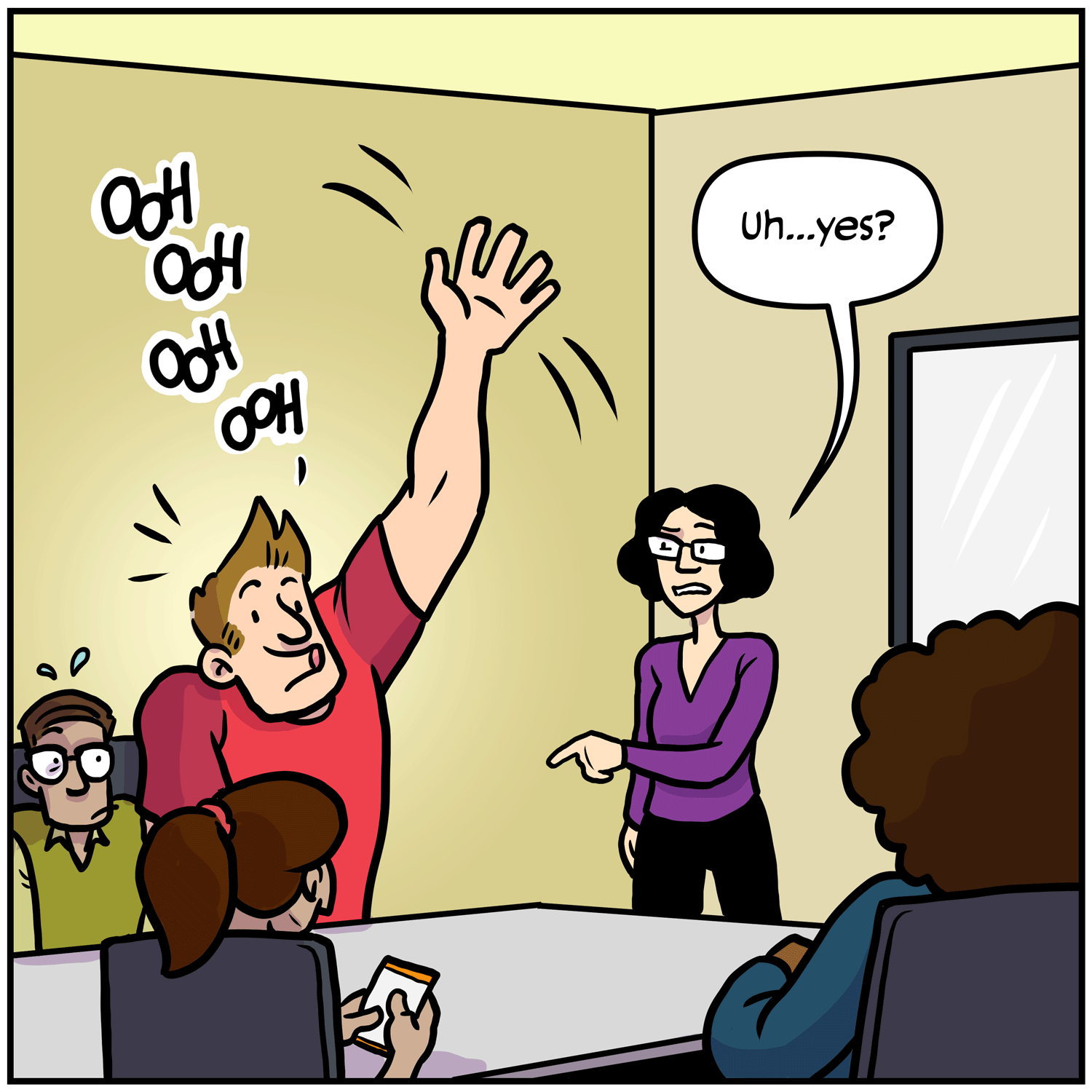
Brad waves his hand while shouting “Ooh! Ooh! Ooh! Ooh!” Matha calls on him while saying “Uh…yes?”
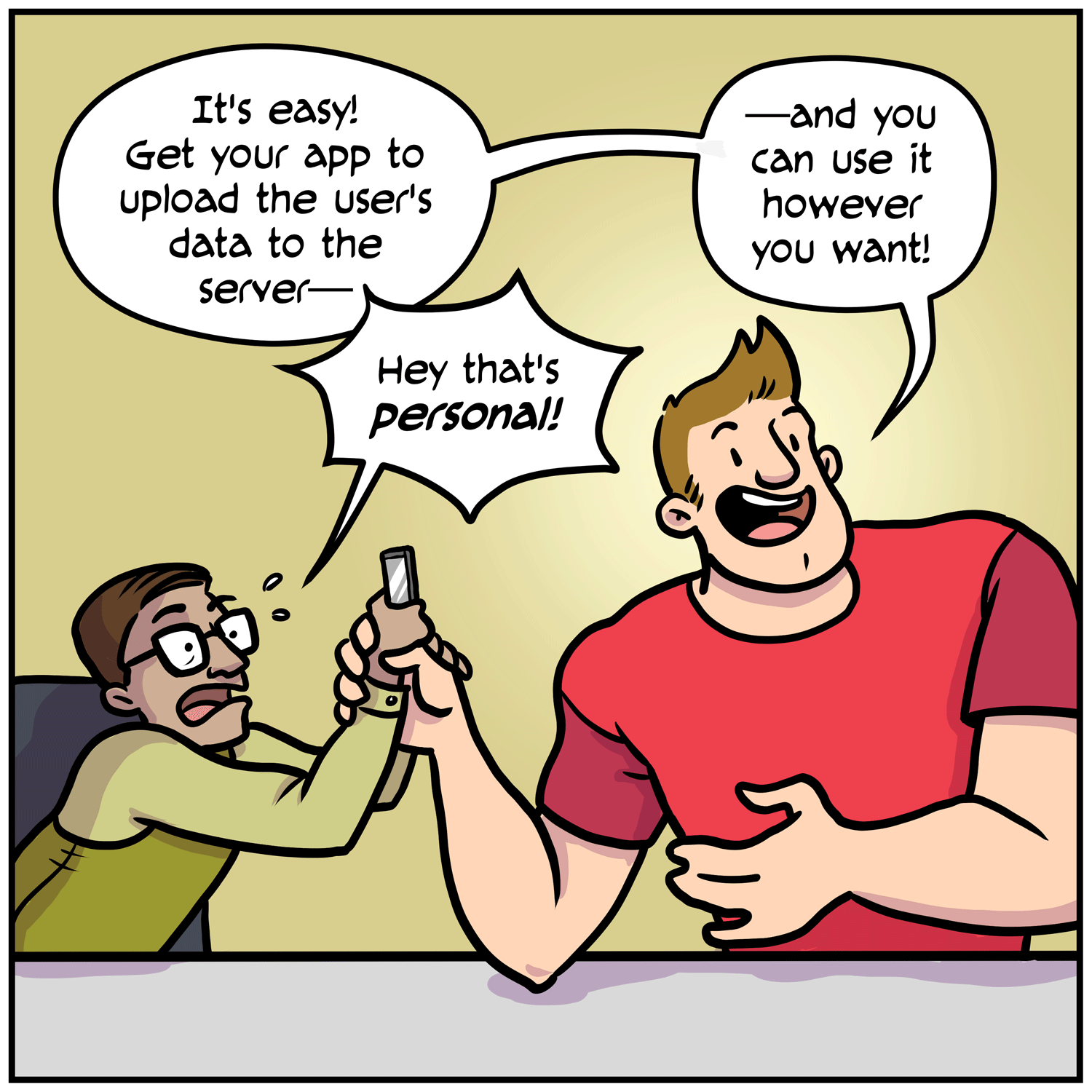
Brad grabs Mateo’s phone, beaming. “It’s easy!” Brad says. “Get your app to upload the user’s data to the server, and you can use it however you want!” Mateo looks panicked and says “Hey, that’s personal!”
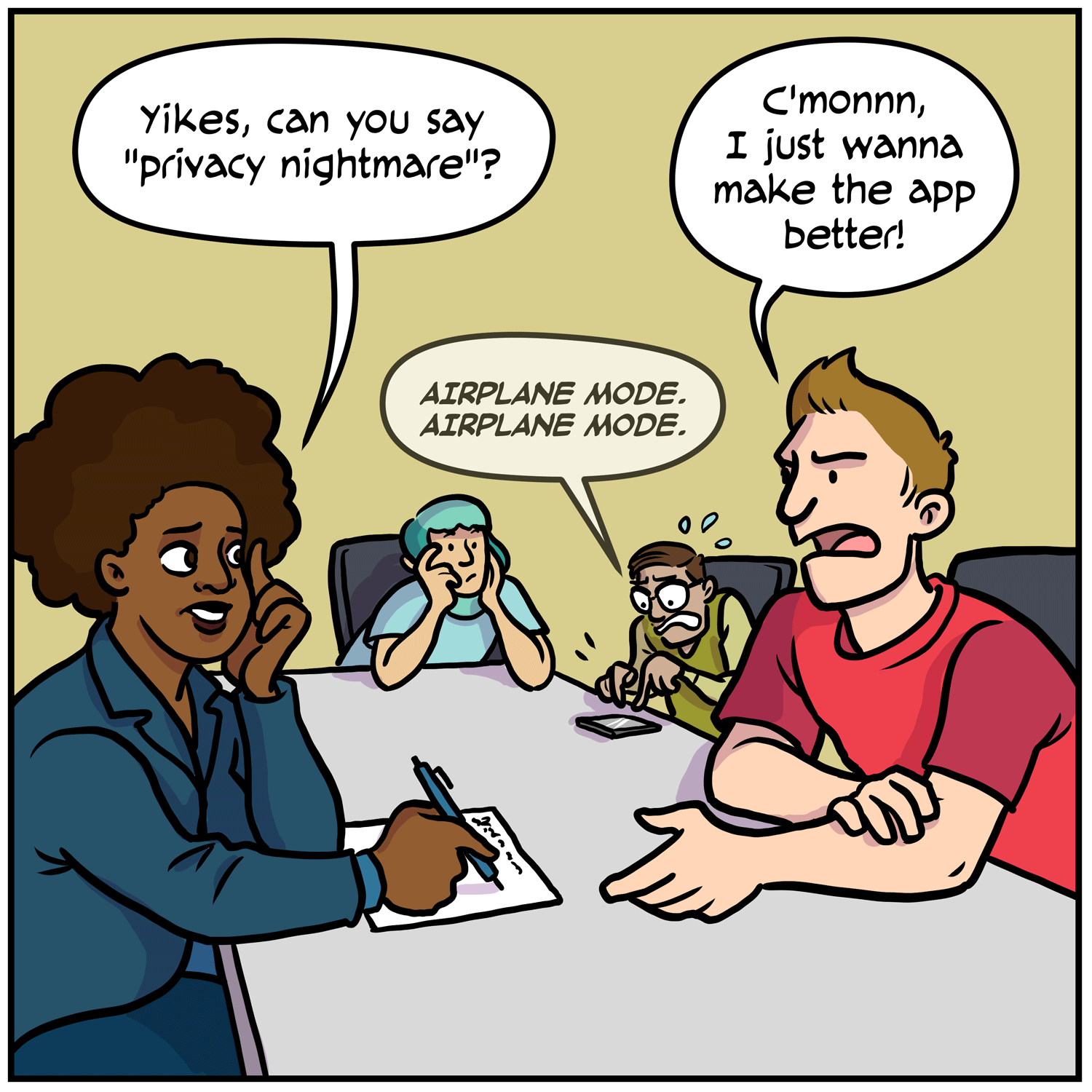
Aliyah looks up from her notepad and says “Wow. Can you say, ‘privacy nightmare’?” In the background, Mateo anxiously tries to switch his phone off while muttering “Airplane mode. Airplane mode.” Brad spreads his hands and whines. “Come on, I just wanna make the app better.”
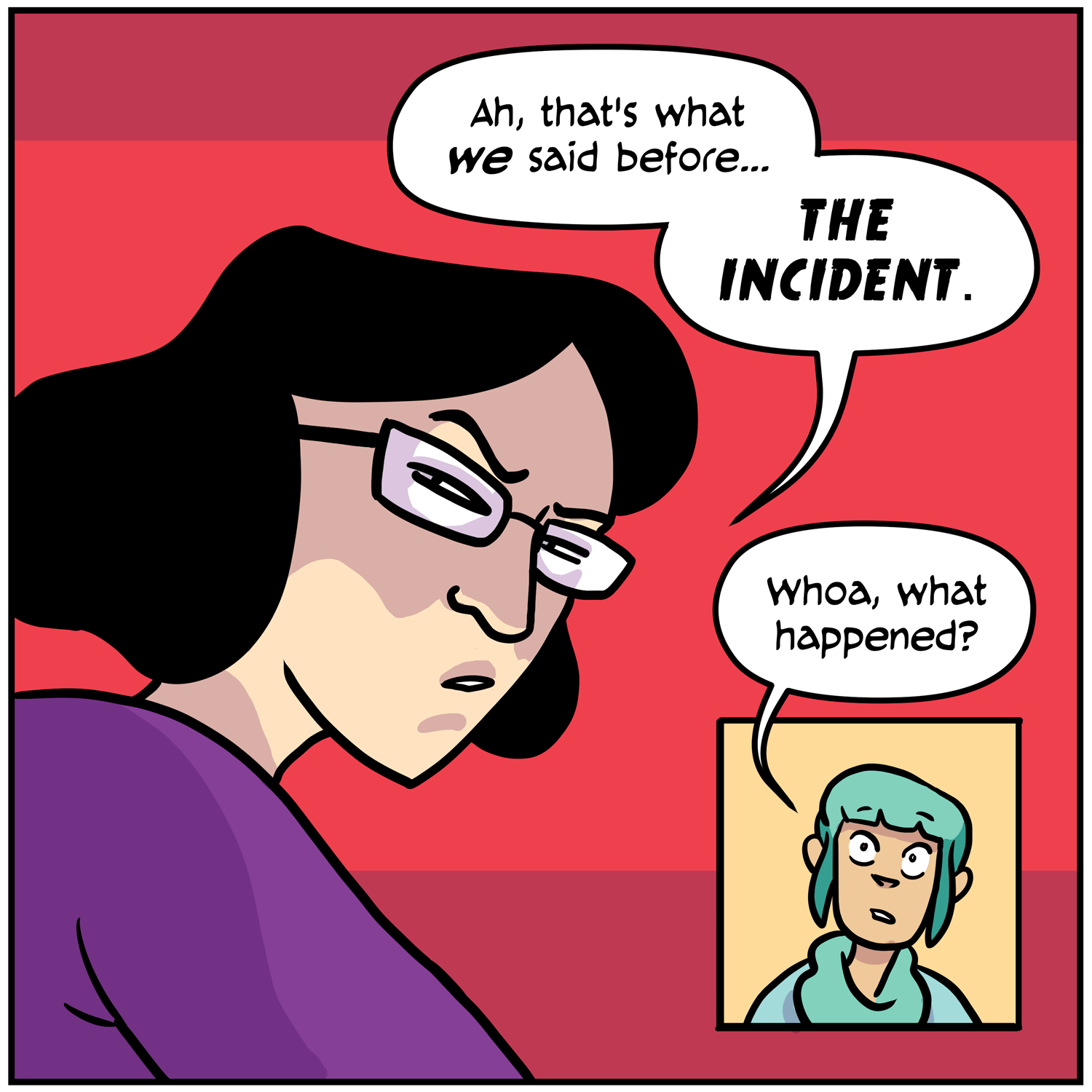
A close-up of Martha’s face, lit dramatically from below. She turns and looks over her shoulder. “Ah,” she says. “That’s what we said before...The Incident.” Kai looks up at her and asks “Whoa, what happened?”
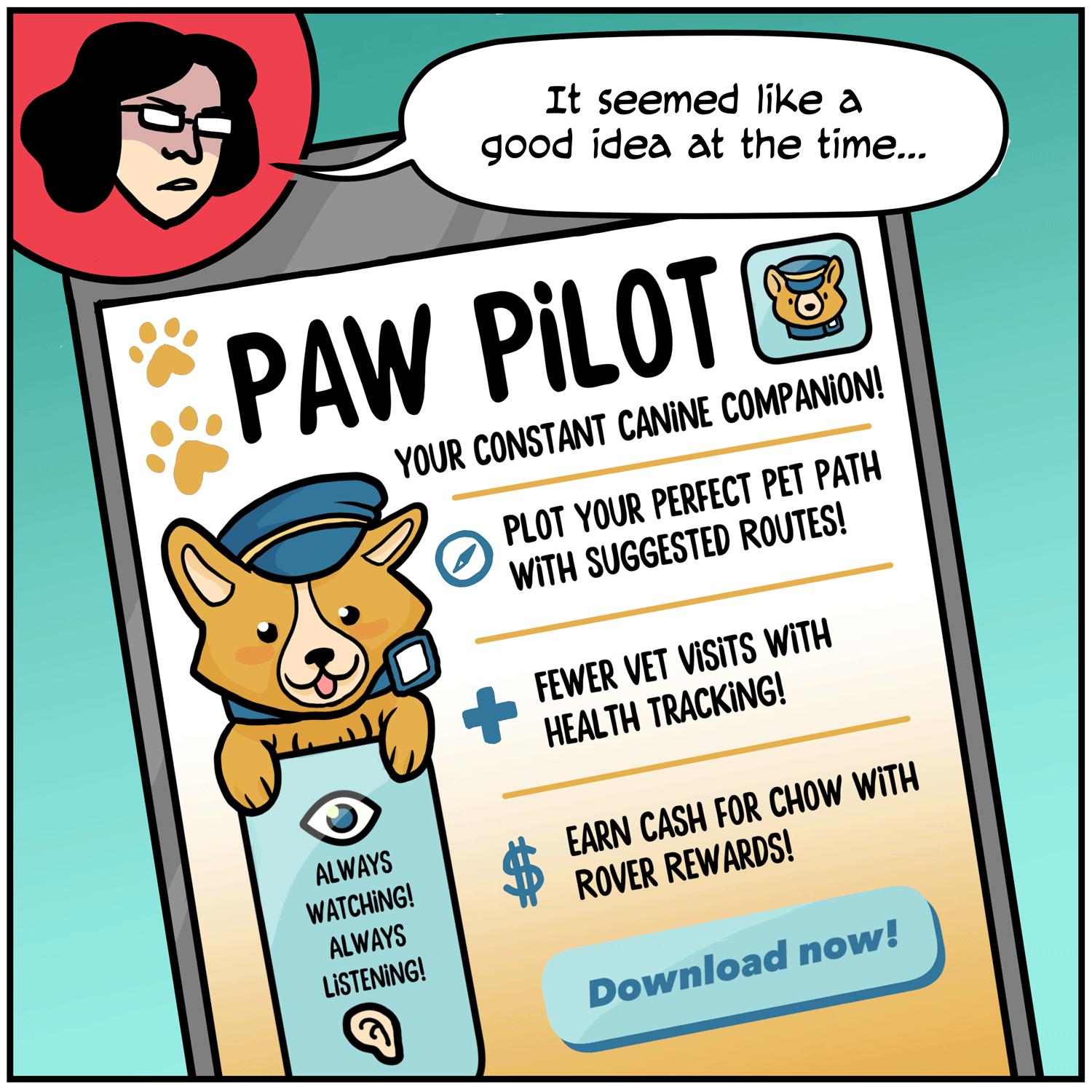
A little icon of Martha’s head in the corner says “It seemed like a good idea at the time…” A smartphone screen showing an app-store advertisement for “Paw Pilot: Your Constant Canine Companion!” The advertised features include: Plot your perfect pet path with suggested routes Fewer vet visits with health tracking Earn cash for chow with Rover Rewards The app has a logo of a cute corgi in a hat sitting above the words “Always watching! Always listening!”
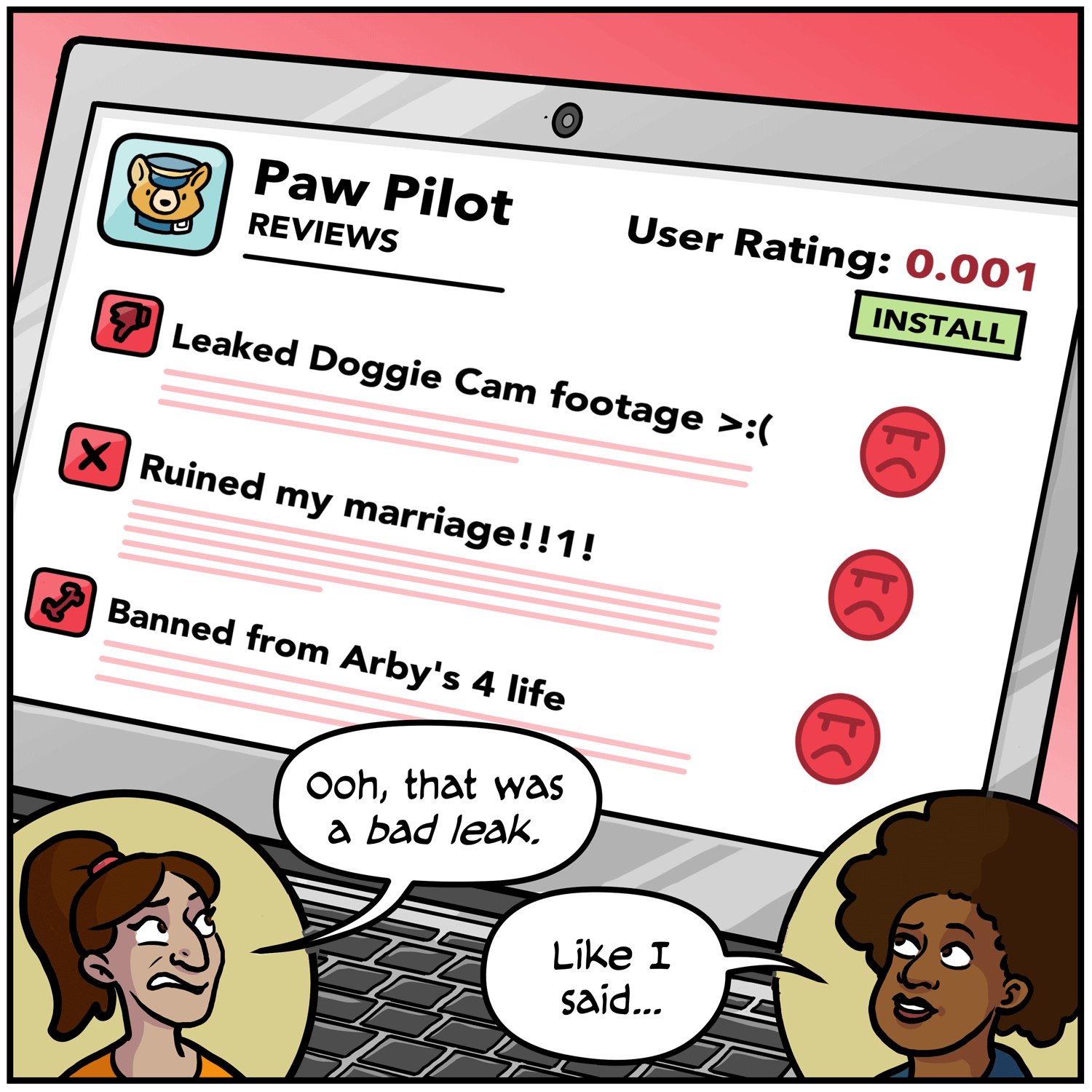
A laptop showing app store reviews of Paw Pilot. It has a dismal rating of 0.001 Angry reviews say “Leaked doggy cam footage!” “Ruined my marriage!” and “Banned from Arby’s for life!” Grouchy red emojis abound. Devi and Aliyah observe from the bottom corner of the panel. “Ooh,” says Devi, wincing. “That was a bad leak.” Aliyah says “Like I said…”
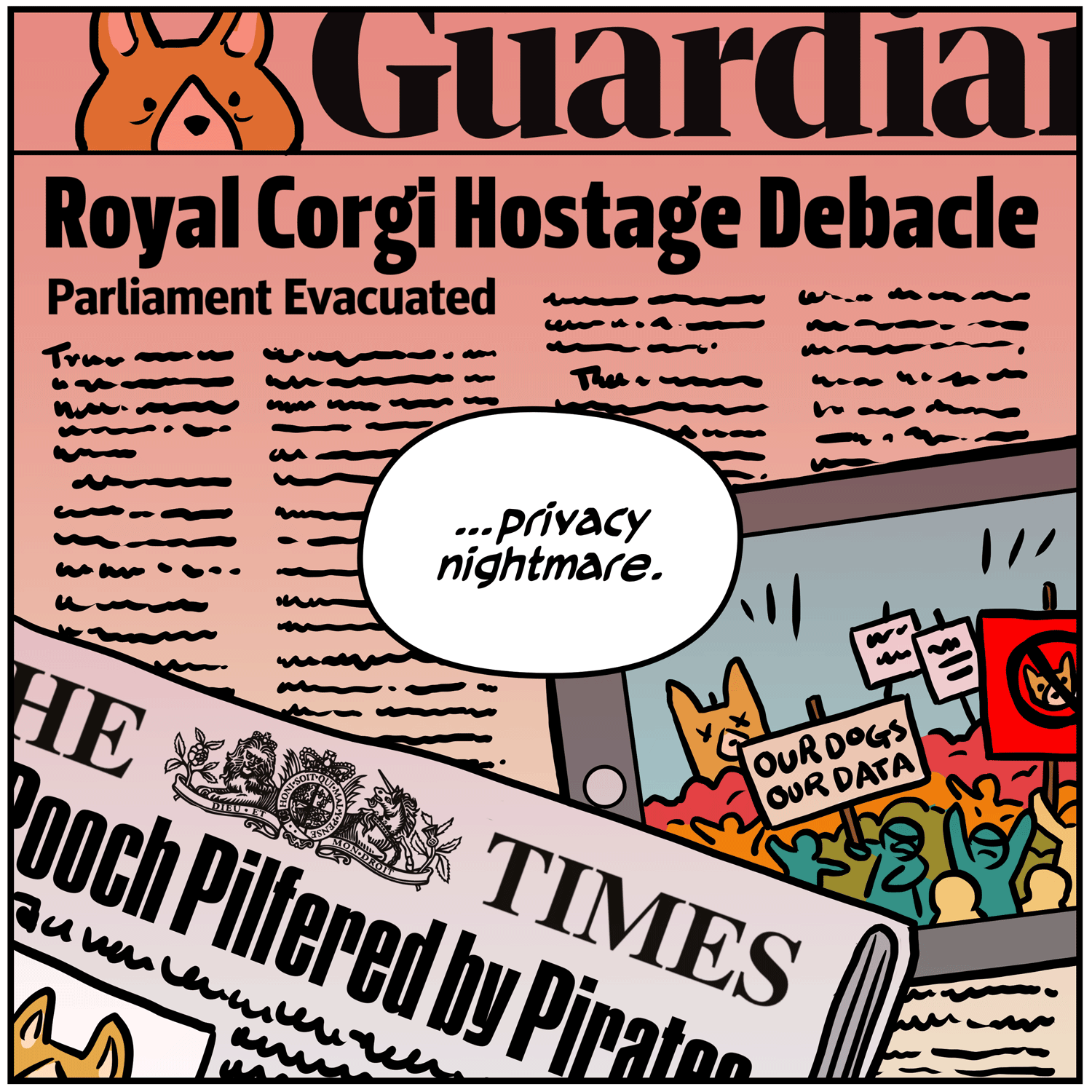
Aliyah finishes speaking from off-panel and says “...privacy nightmare.” An assortment of dramatic headlines from The Guardian (“Royal Corgi Hostage Debacle”) and The Times (“Pooch Pilfered by Pirates). A tablet devices plays footage of protesters carrying signs that say “Our dogs, our data.”
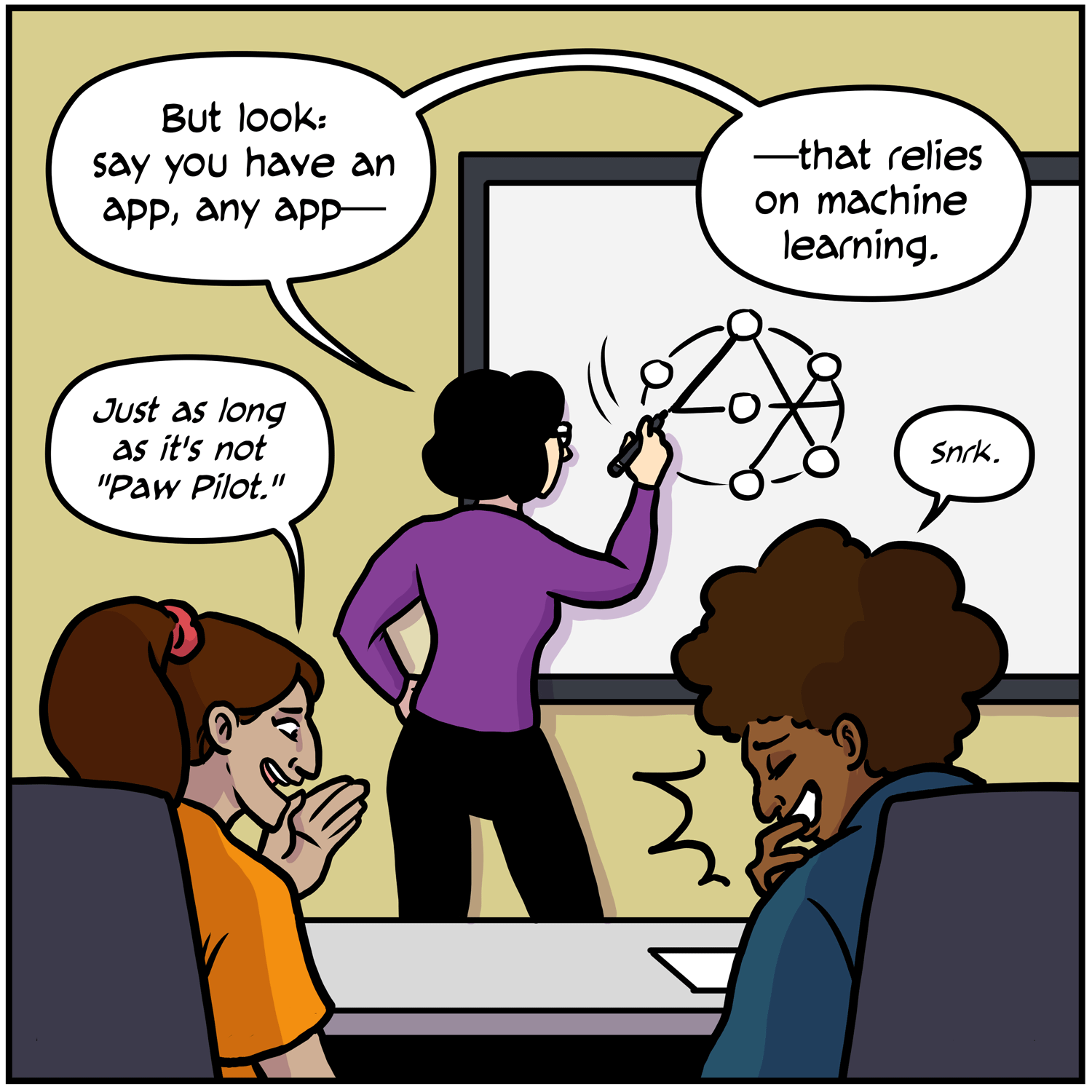
Back in the conference room, Martha sketches a neural network model on a whiteboard. Martha says: “But look…say you have an app, any app–that relies on machine learning.” Devi and Aliyah sit in the foreground and snicker to each other. “Just as long as it’s not Paw Pilot,” says Devi.
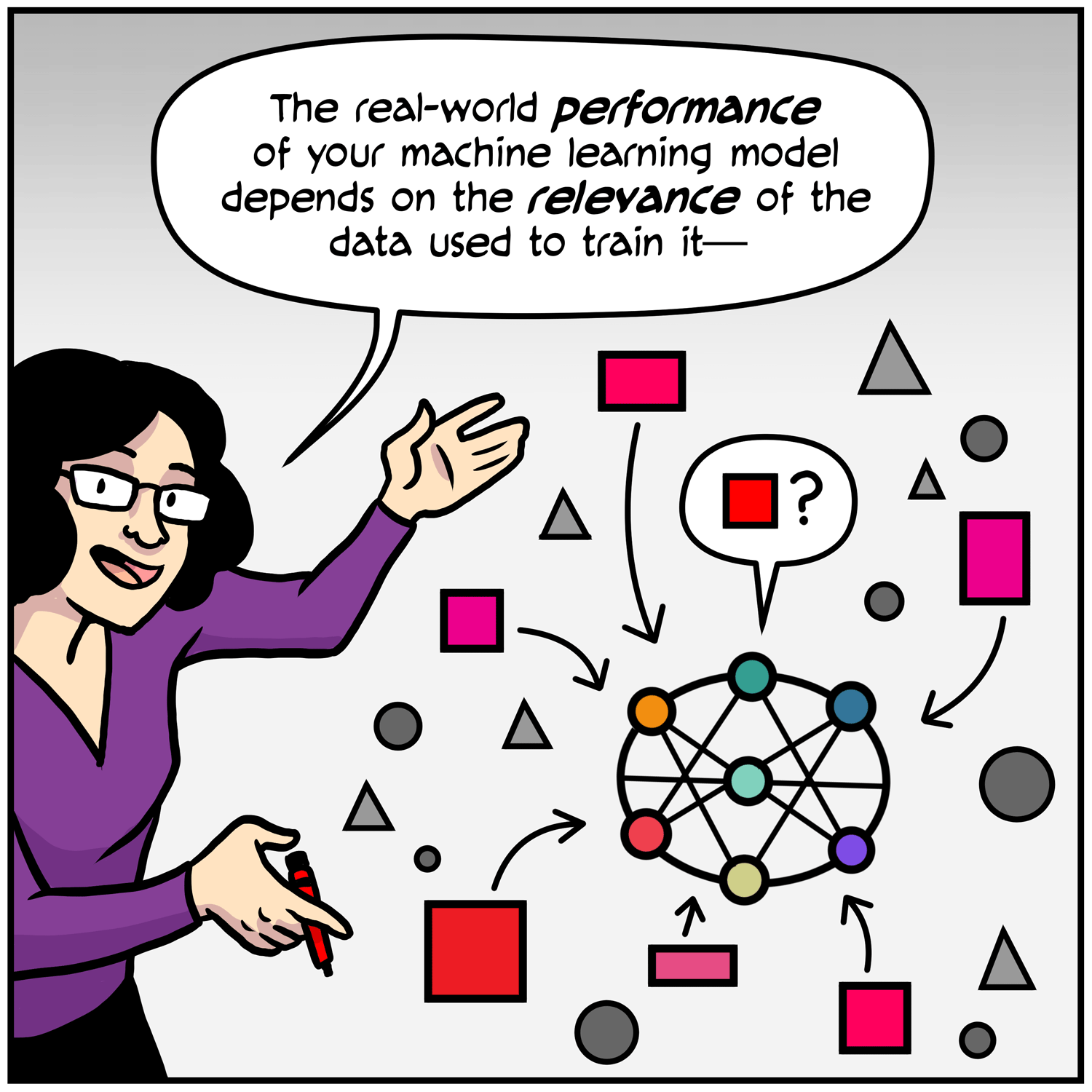
Martha reveals her whiteboard diagram, showing a central brain-like network querying a variety of shapes around it. Martha says “The real-world performance of your machine learning model depends on the relevance of the data used to train it.”
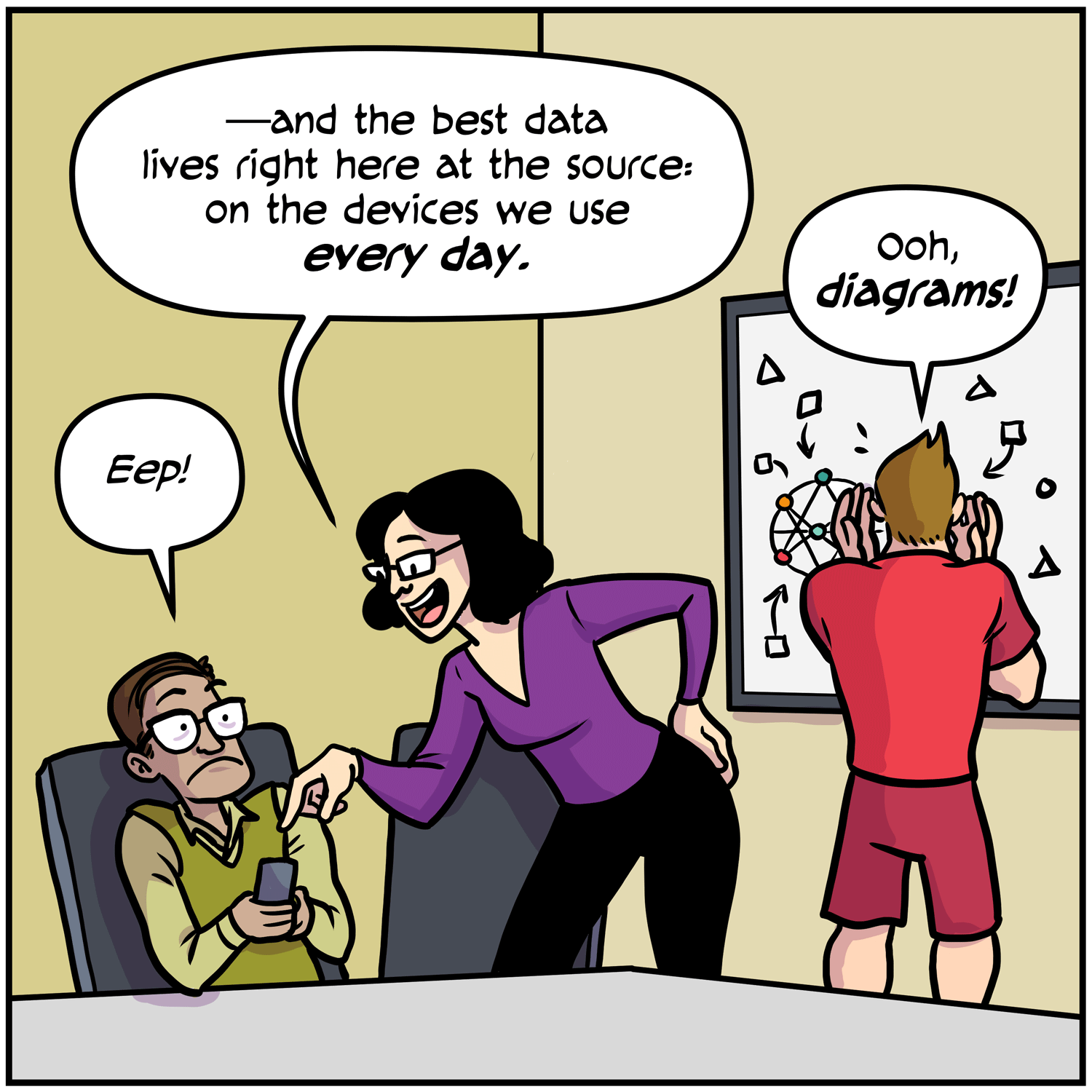
Martha leans over to gesture at Mateo’s phone, saying “And the best data lives right here at the source on the devices we use every day.” Mateo recoils and says “Eep!” In the background, Brad claps his hands in front of the whiteboard and squeals “Ooh, diagrams!”
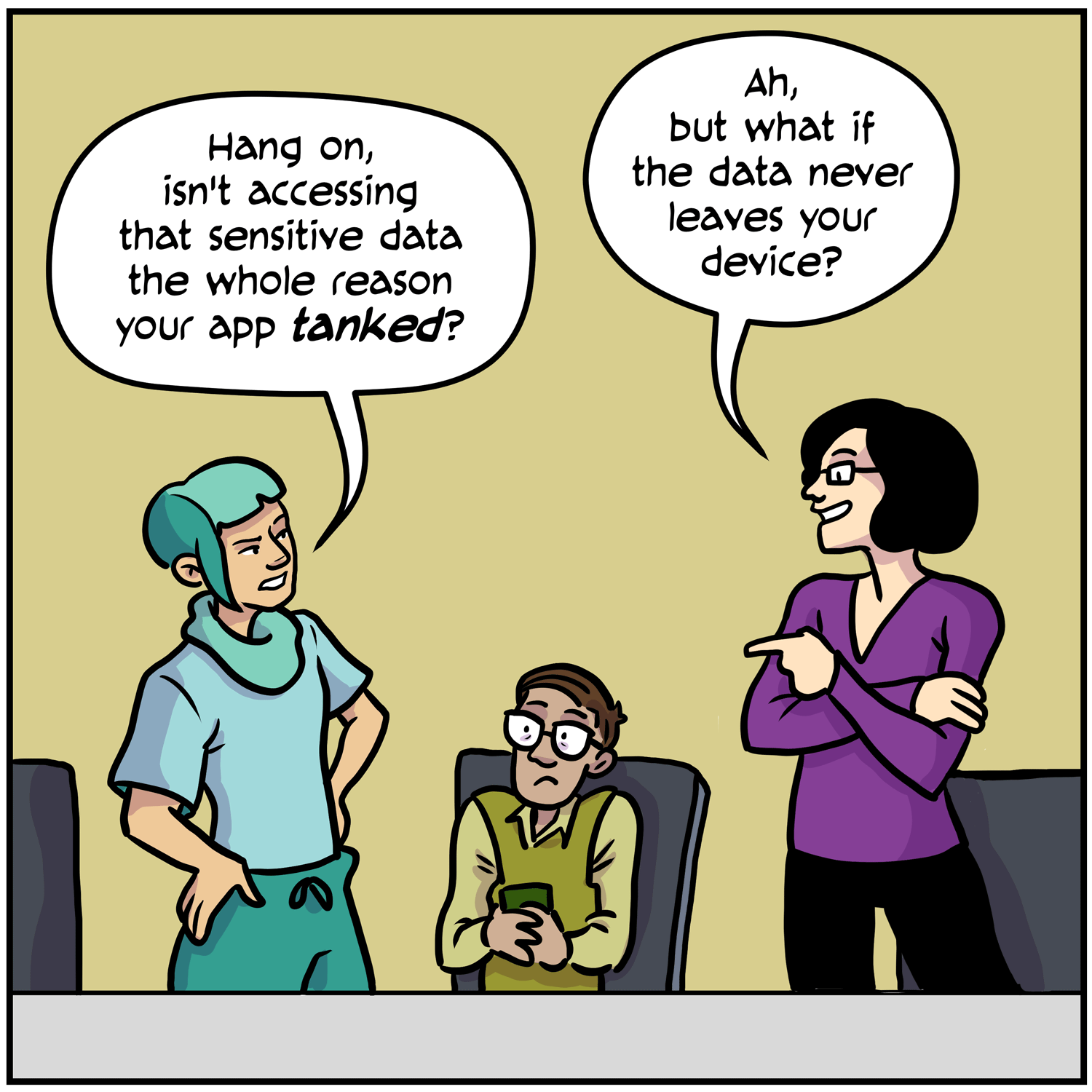
Kai looks skeptically at Martha and says “Hang on, isn’t accessing that sensitive data the whole reason your app tanked?” Mateo looks nervous between them. Martha looks sly and says “Ah, but what if the data never leaves your device?”
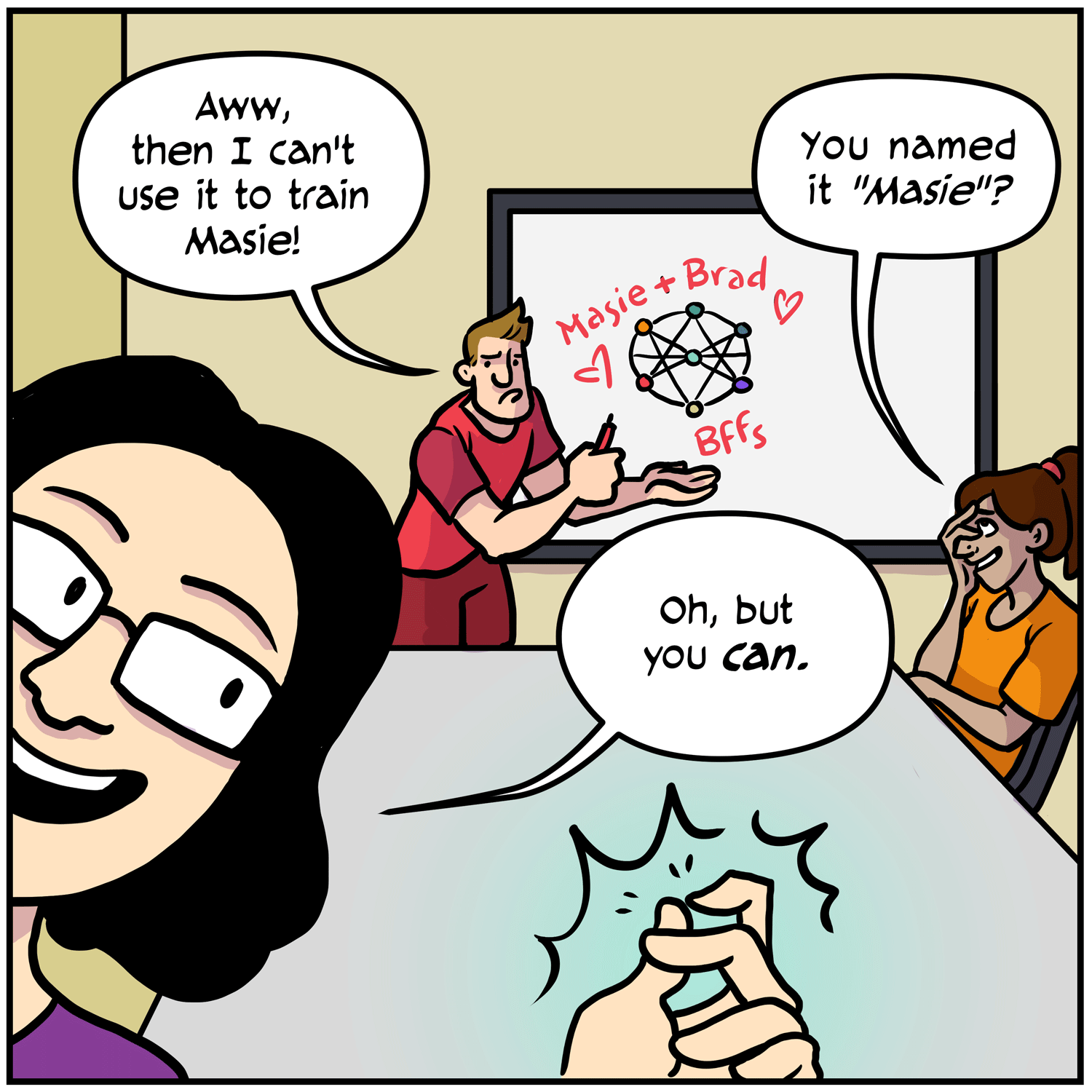
Brad pouts, gesturing to the white-board, where he has annotated the diagram with a bunch of red hearts and the words “Masie + Brad, BFFs.” He says “Aww, then I can’t use it to train Masie.” Martha grins and snaps her fingers in the foreground saying “Oh, but you can.” Aliyah looks at Brad incredulously and mutters “You named it ‘Masie’?”
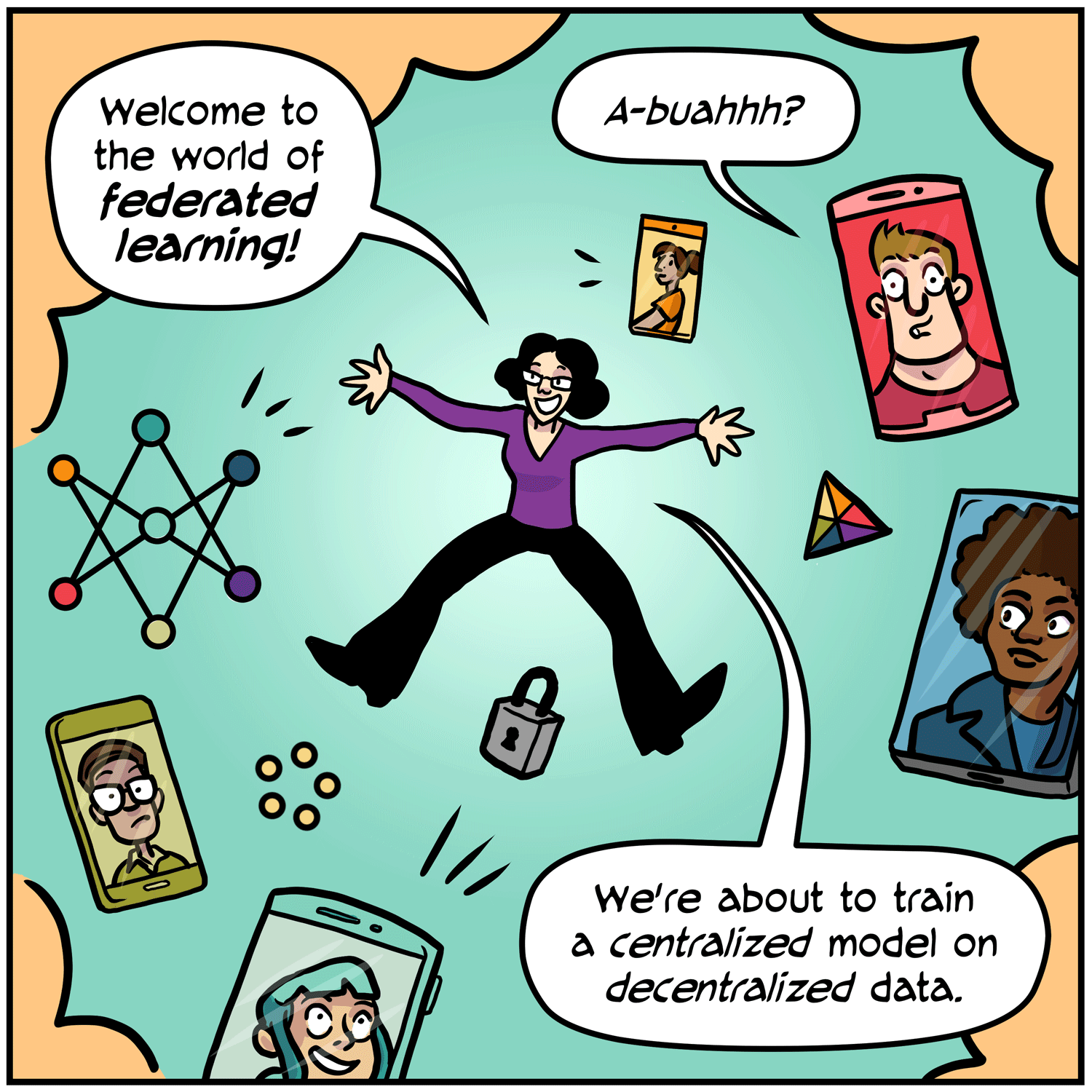
Martha pops into view among a field of smartphones matched to each of the interns, who all sit inside their screens as tiny versions of themselves. Martha is surrounded by icons depicting neural networks, security features, and training models. Martha shouts “Welcome to the world of federated learning! We’re about to train a centralized model on decentralized data.” Brad looks awed.
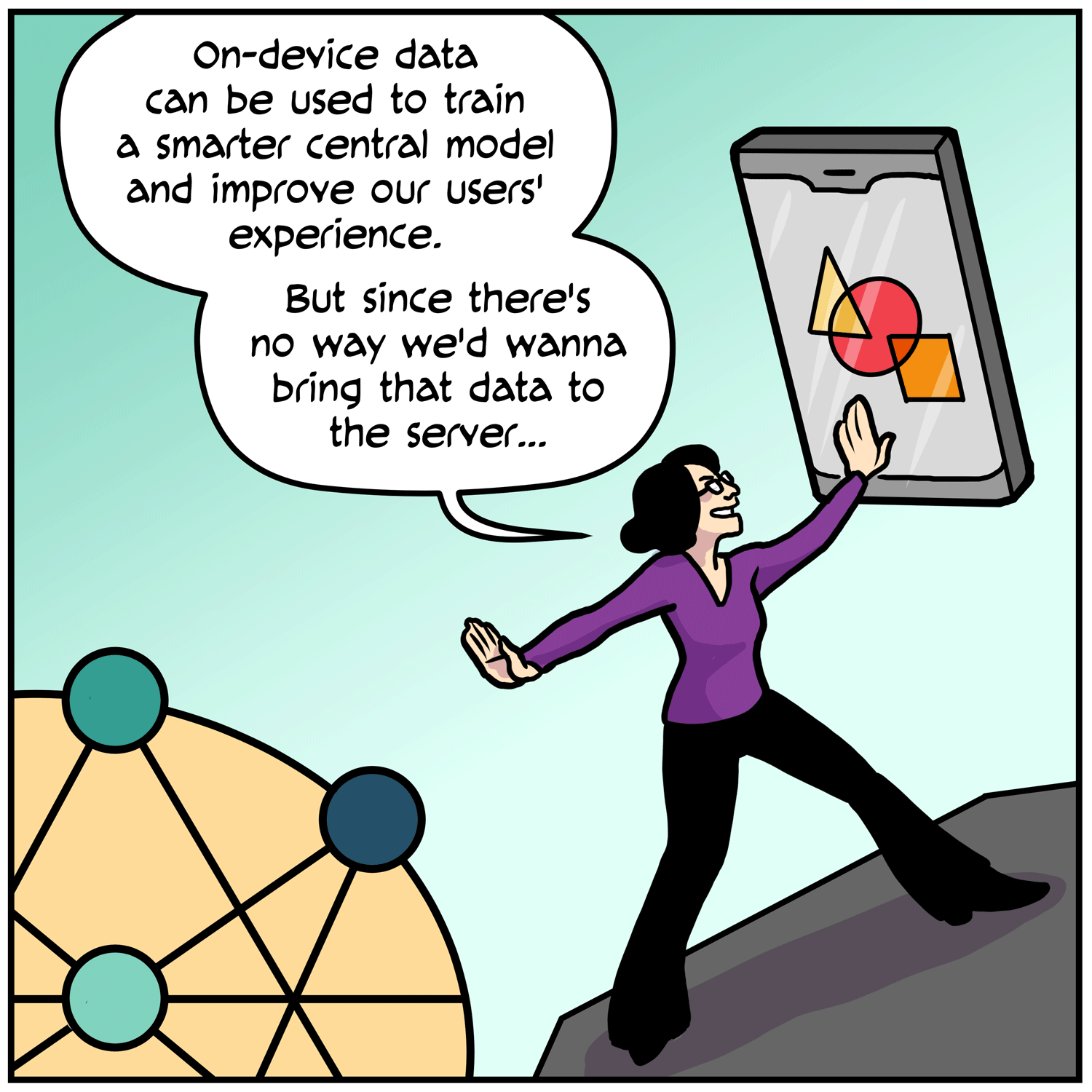
Martha stands between a central neural network model and a floating smartphone, holding out her hands to stop them from interacting. She says “On-device data can be used to train a smarter central model and improve our users’ experience. But since there’s no way we’d wanna bring that data to the server…”
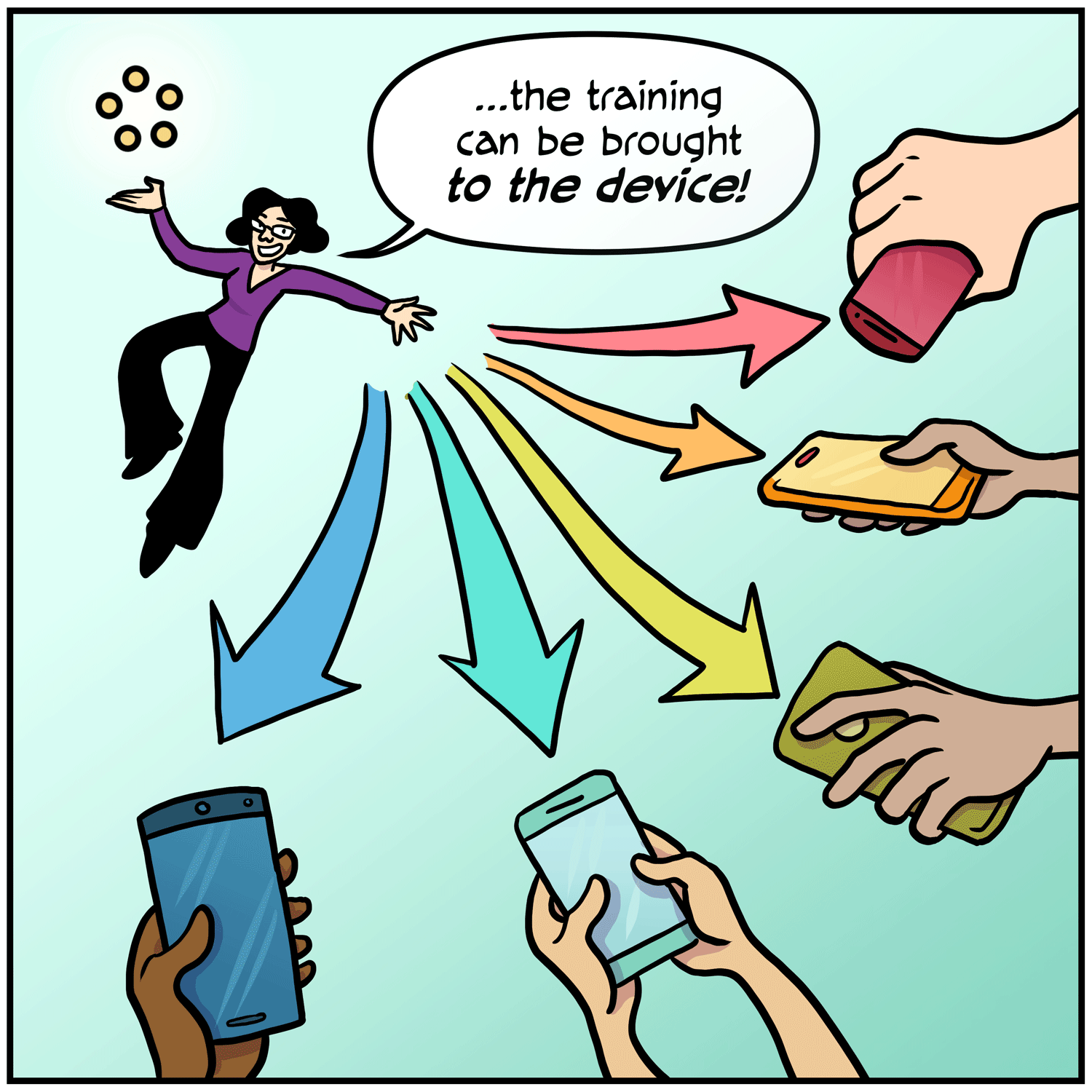
A small neural network training model floats above Martha’s hand. She’s ready to toss it to the five interns’ mobile phones. Martha says “..the training can be brought to the device!”
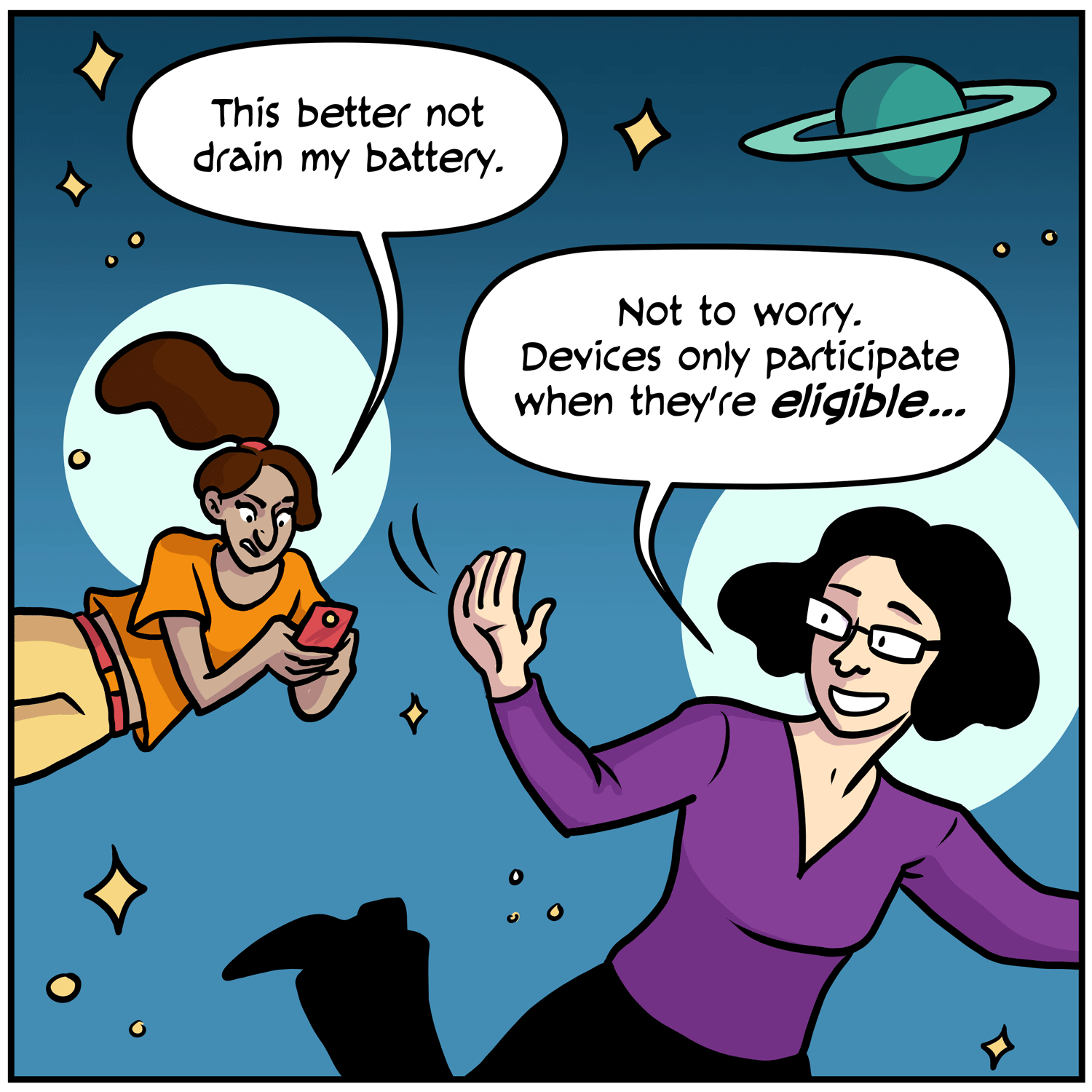
Devi and Martha float through space. A planet drifts by. Devi is busy typing on her phone. She says “This better not drain my battery.” Martha says “Not to worry. Devices only participate when they’re eligible.”
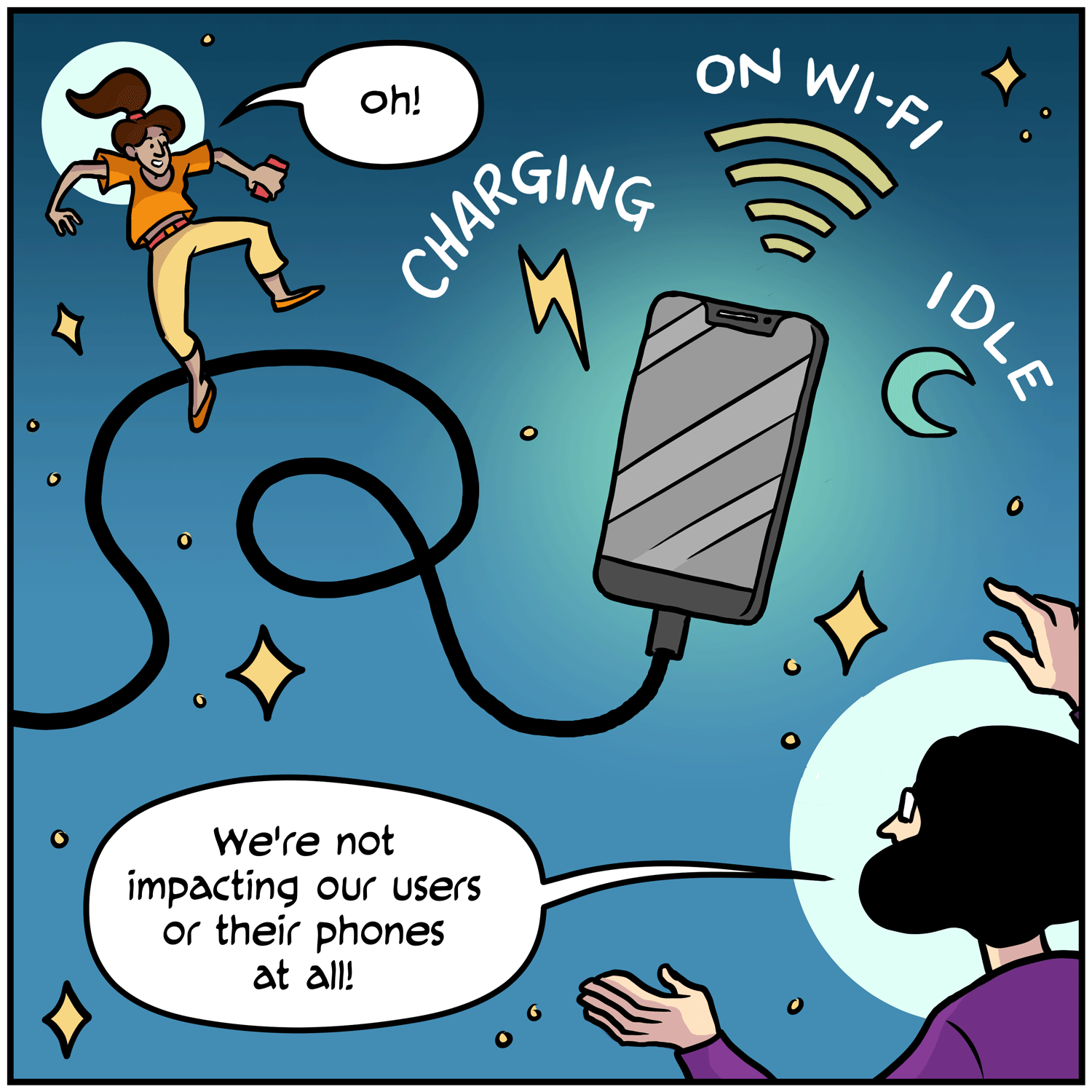
Devi and Martha float past a large smartphone, plugged in and displaying a Do Not Disturb icon on its screen. Devi looks startled and says “Whoa!” The smartphone is surrounded by labels that say “charging,” “on wi-fi,” and “idle,” the basic requirements for eligibility. Martha says “We’re not impacting our users or their phones at all!”
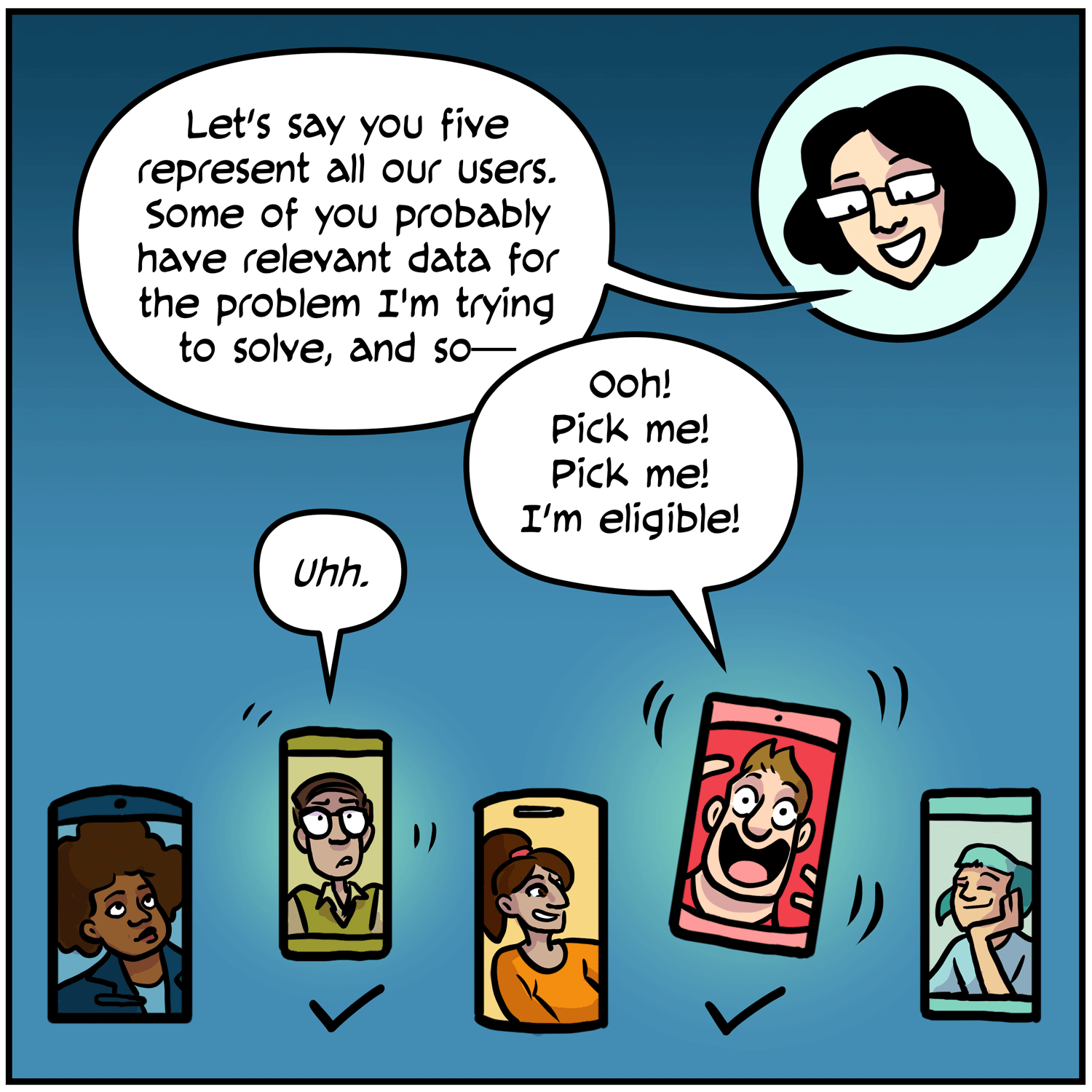
All five interns appear in their phones at the bottom of the panel. Mateo and Brad’s phones float slightly higher in the lineup with tiny check marks below them. Martha floats further above them and says “Let’s say you five represent all our users. Some of you probably have relevant data for the problem I’m trying to solve, and so—” Brad interrupts her, practically hopping out of his phone saying “Ooh! Pick me! Pick me! I’m eligible.” Mateo looks anxious and says “Uhh.”
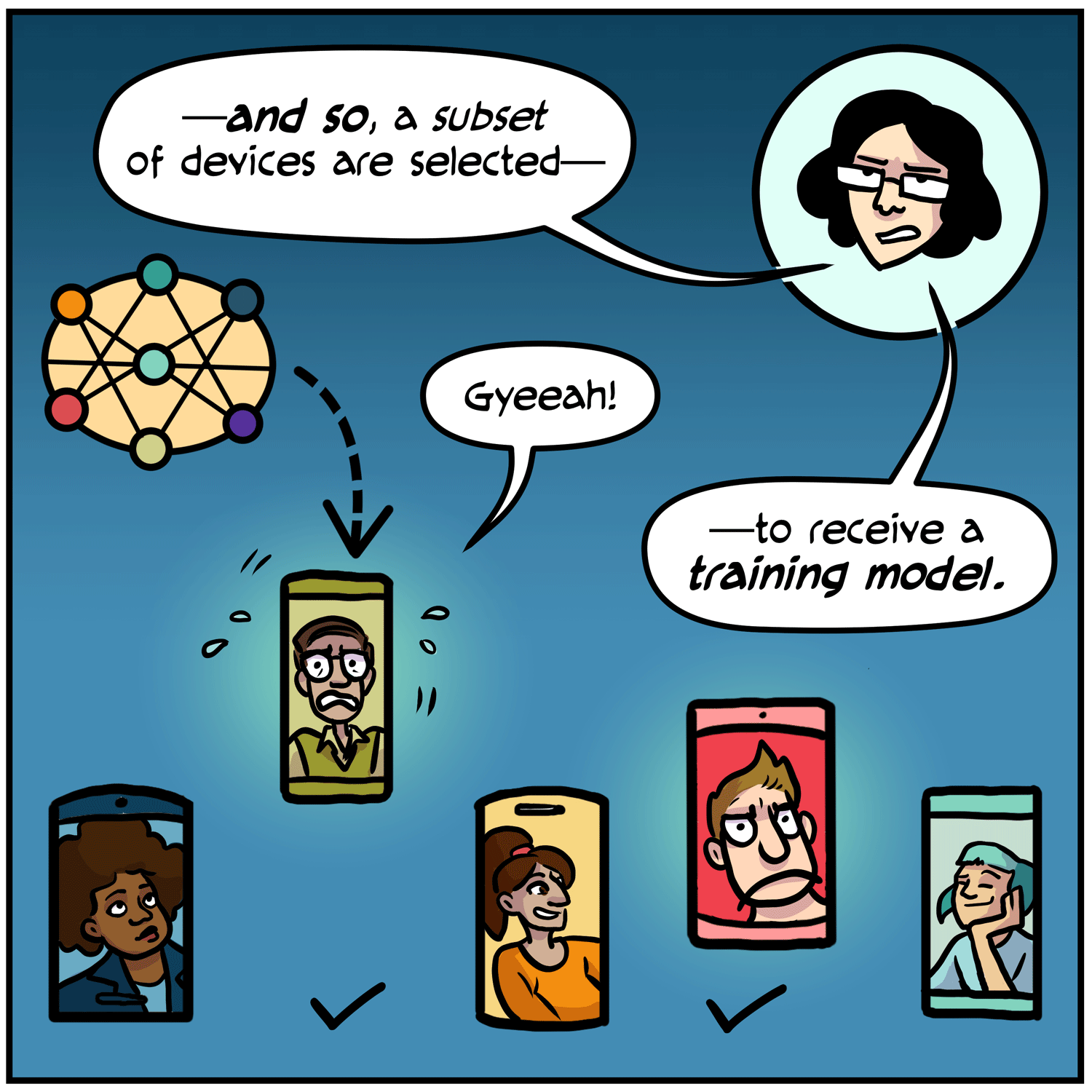
Martha rolls her eyes and forges ahead, ignoring Brad. “–and so, a subset of devices are selected to receive a training model.” The central neural network model points at Mateo’s phone. He lets out a nervous yelp.
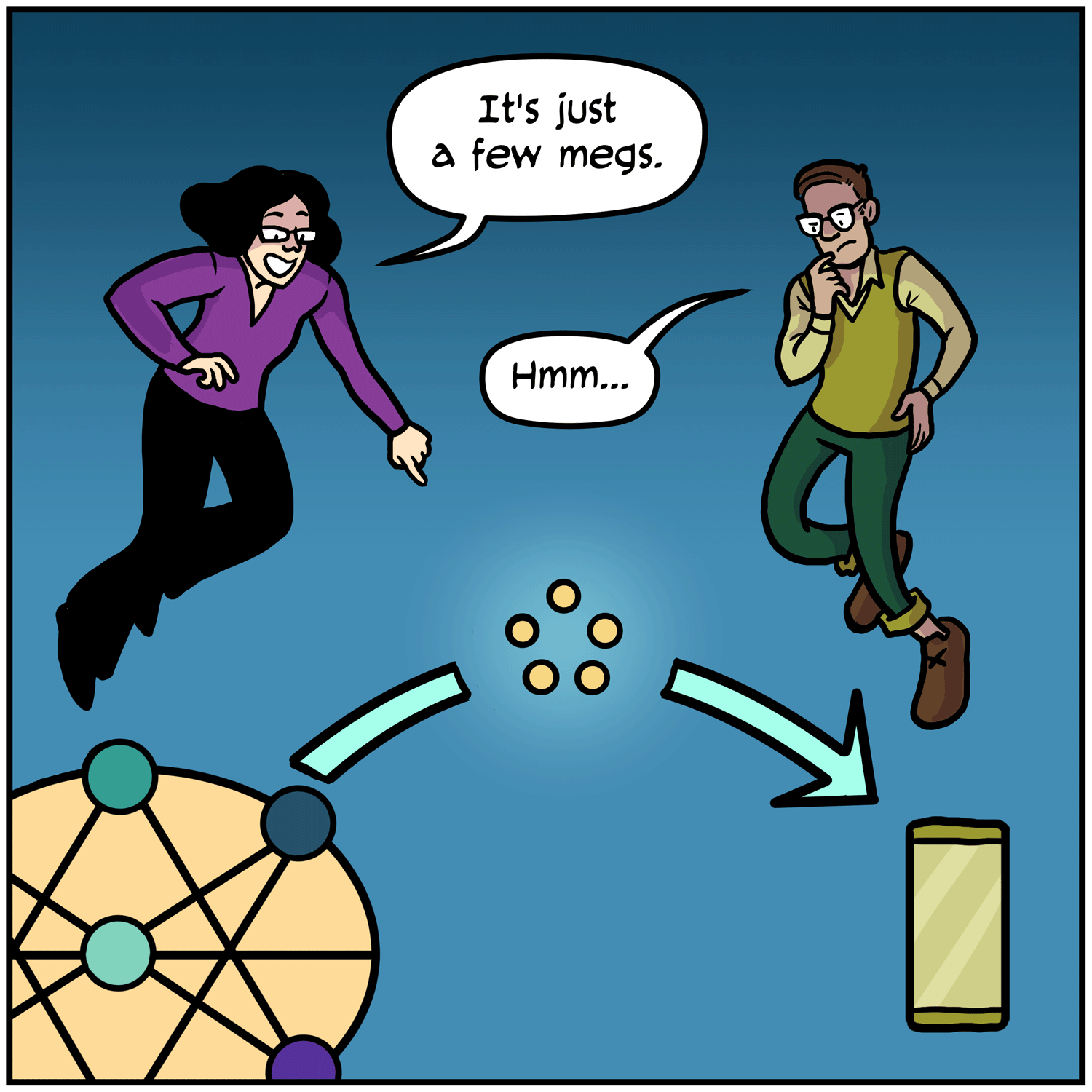
Martha and Mateo float above the following diagram: The central neural network model sends a small yellow training model over to Mateo’s phone. Martha reassures him by saying “It’s just a few megs.” Mateo doesn’t look convinced and says “Hmm.”
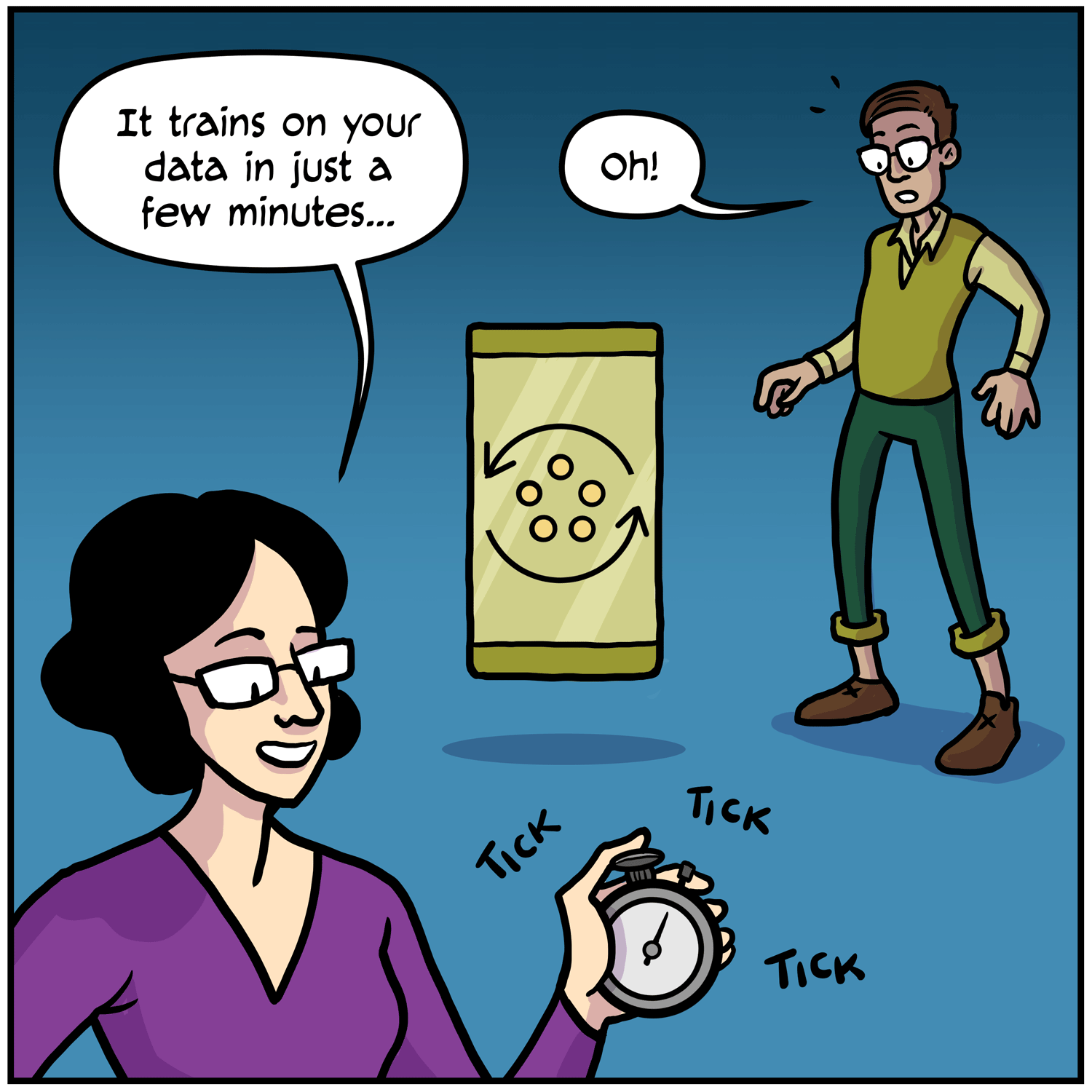
The small neural network training model appears inside of Mateo’s phone. Martha holds up a stopwatch and says “It trains on your data in just a few minutes...” Mateo looks surprised and says “Oh!”
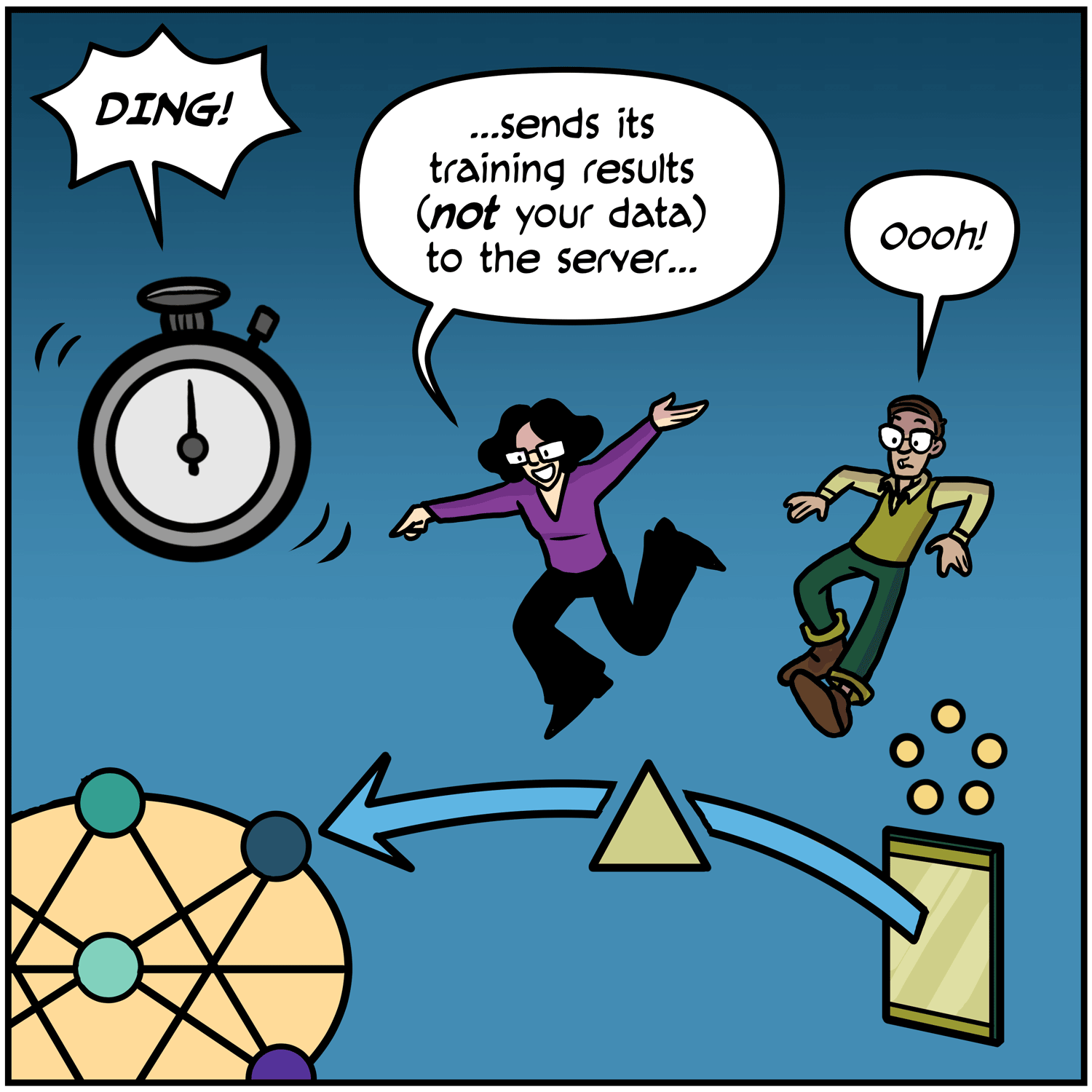
The stopwatch goes ding! Martha continues: “...sends its training results (not your data) to the server…” The training model now floats above Mateo’s phone, as a delta symbol gets sent back toward the central neural network. Mateo looks more intrigued, saying “Oooh!”
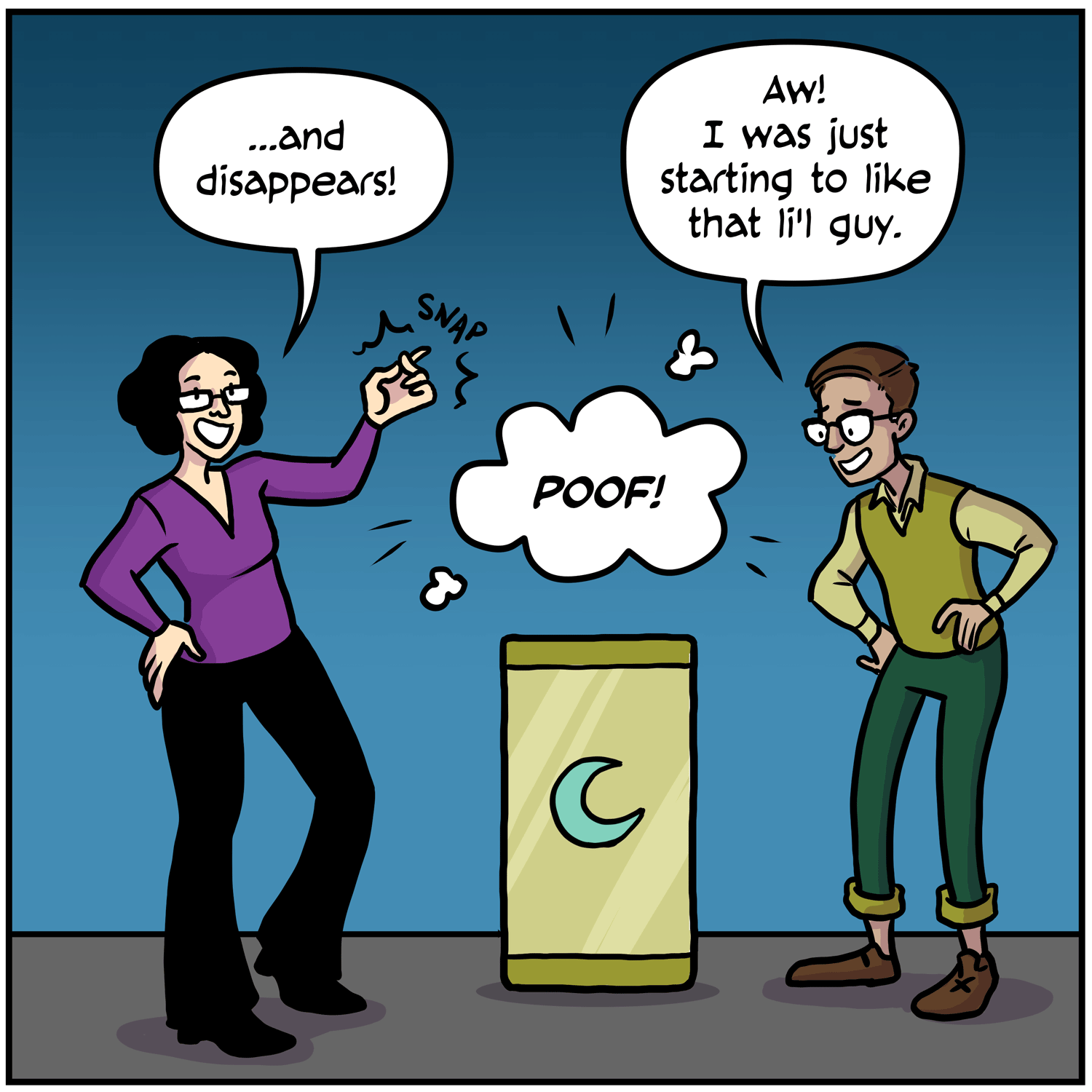
Martha snaps her fingers, saying “…and disappears!” The model vanishes from above Mateo’s phone with a poof. Mateo smiles and looks rueful, saying “Aw! I was just starting to like that little guy.”
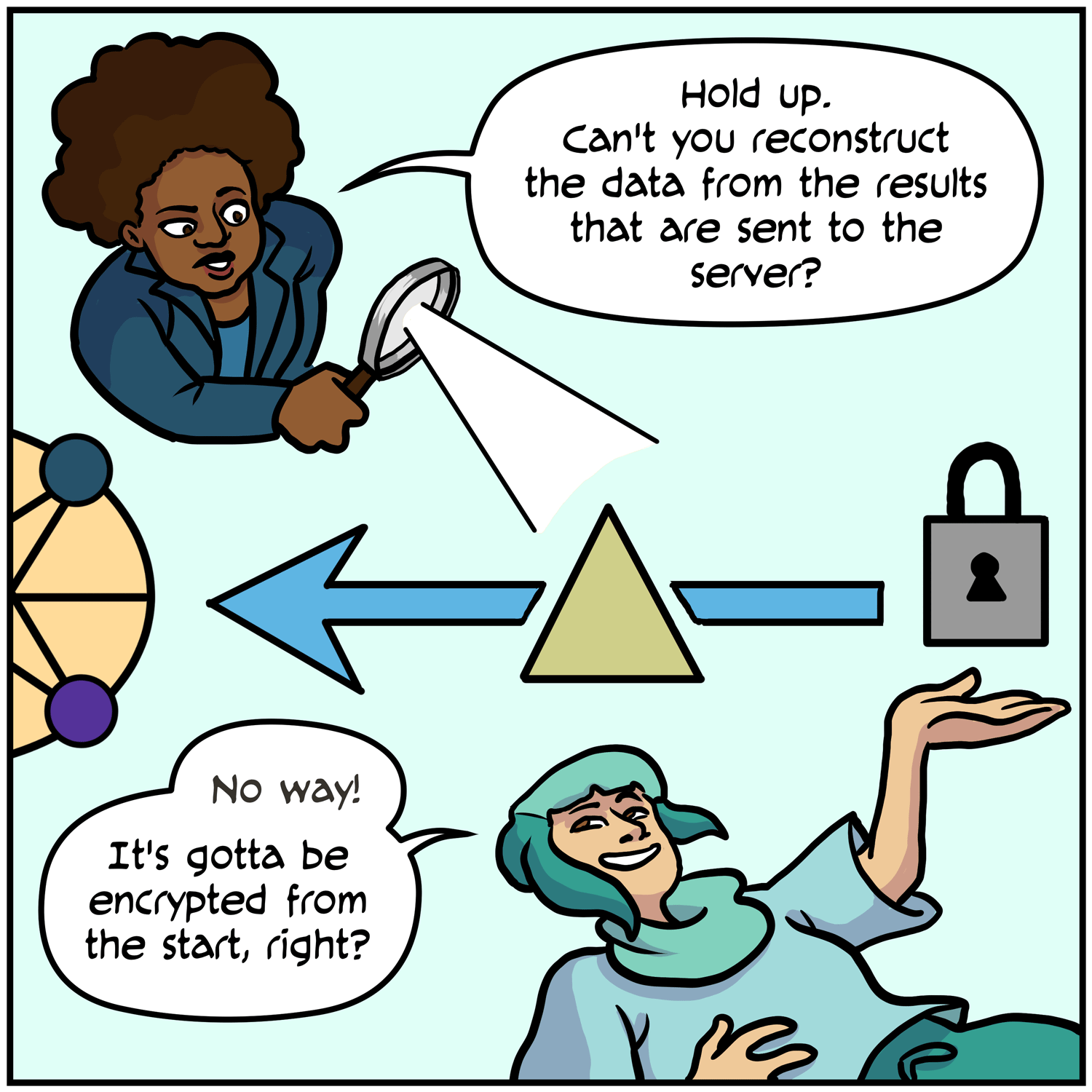
Aliyah and Kai investigate the passage of the delta symbol toward the central neural network. Aliyah holds up a magnifying glass and says “Hold up. Can’t you reconstruct the data from the results that are sent to the server?” Kai floats below and gestures to the right-hand side of the panel, where there’s a lock. “No way!” Kai says, “It’s gotta be encrypted from the start, right?”
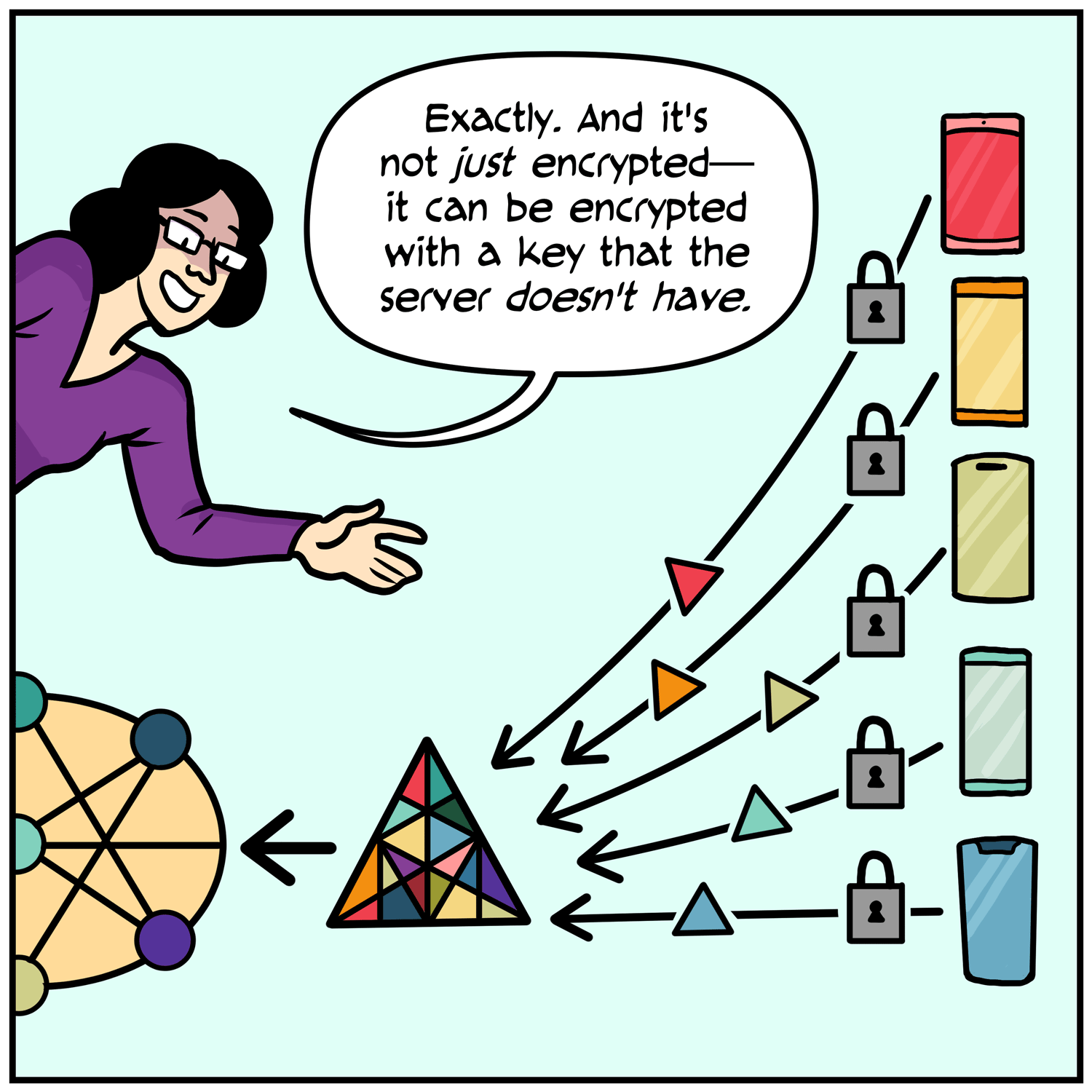
Martha leans in from off-panel, saying “Exactly. And it’s not just encrypted, it can be encrypted with a key that the server doesn’t have.” Below Martha, a line of phones all send delta symbols back toward the central model. Before they reach it, the deltas combine into a larger delta symbol, rendering their individual characteristics anonymous.
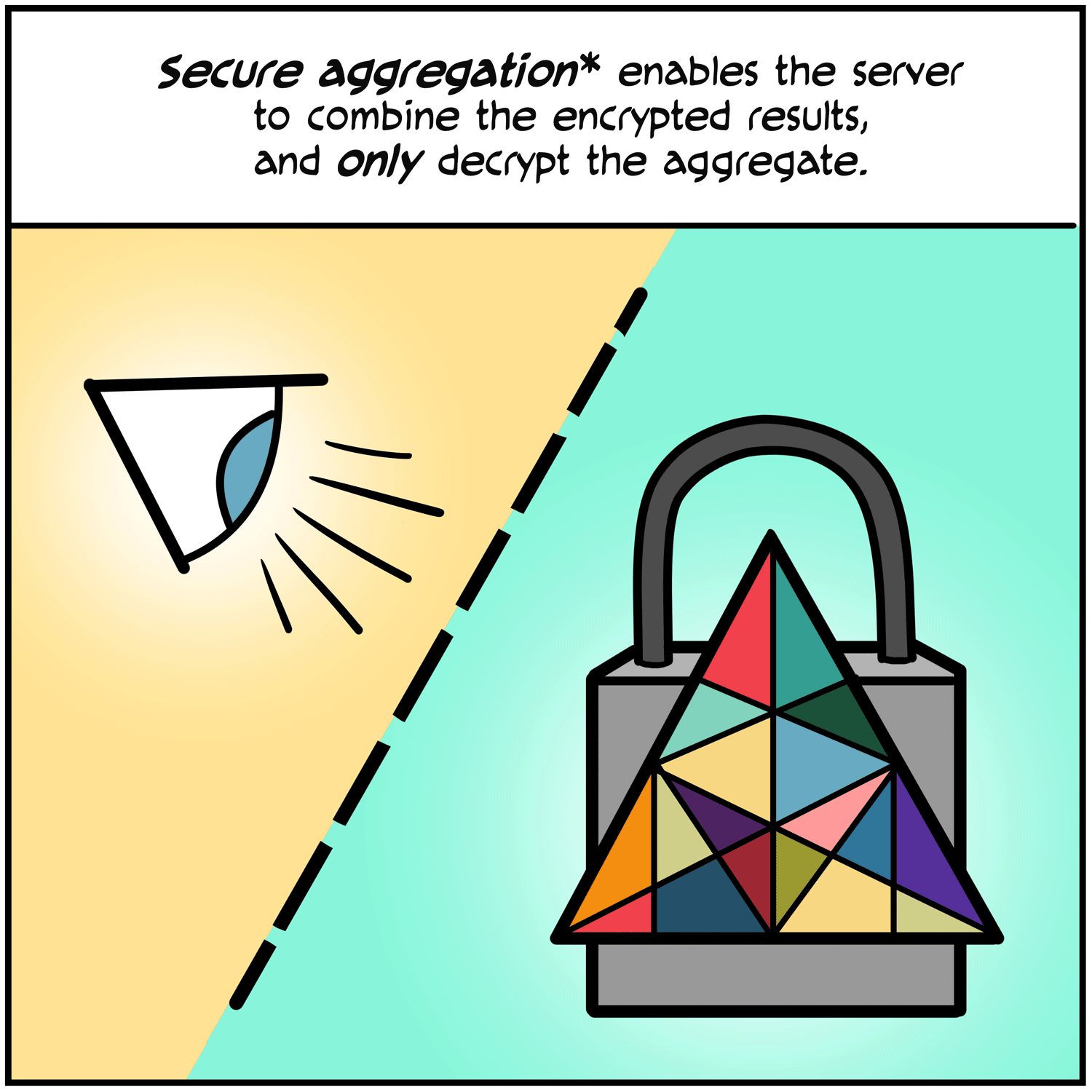
Martha narrates from off-panel, saying “Secure aggregation enables the server to combine the encrypted results, and only decrypt the aggregate.”
Secure aggregation is an interactive cryptographic protocol for computing sums of masked vectors, like model weights. It works by coordinating the exchange of random masks among pairs of participating clients, such that the masks cancel out when a sufficient number of inputs are received. To read more about secure aggregation, see Practical Secure Aggregation for Privacy-Preserving Machine Learning.
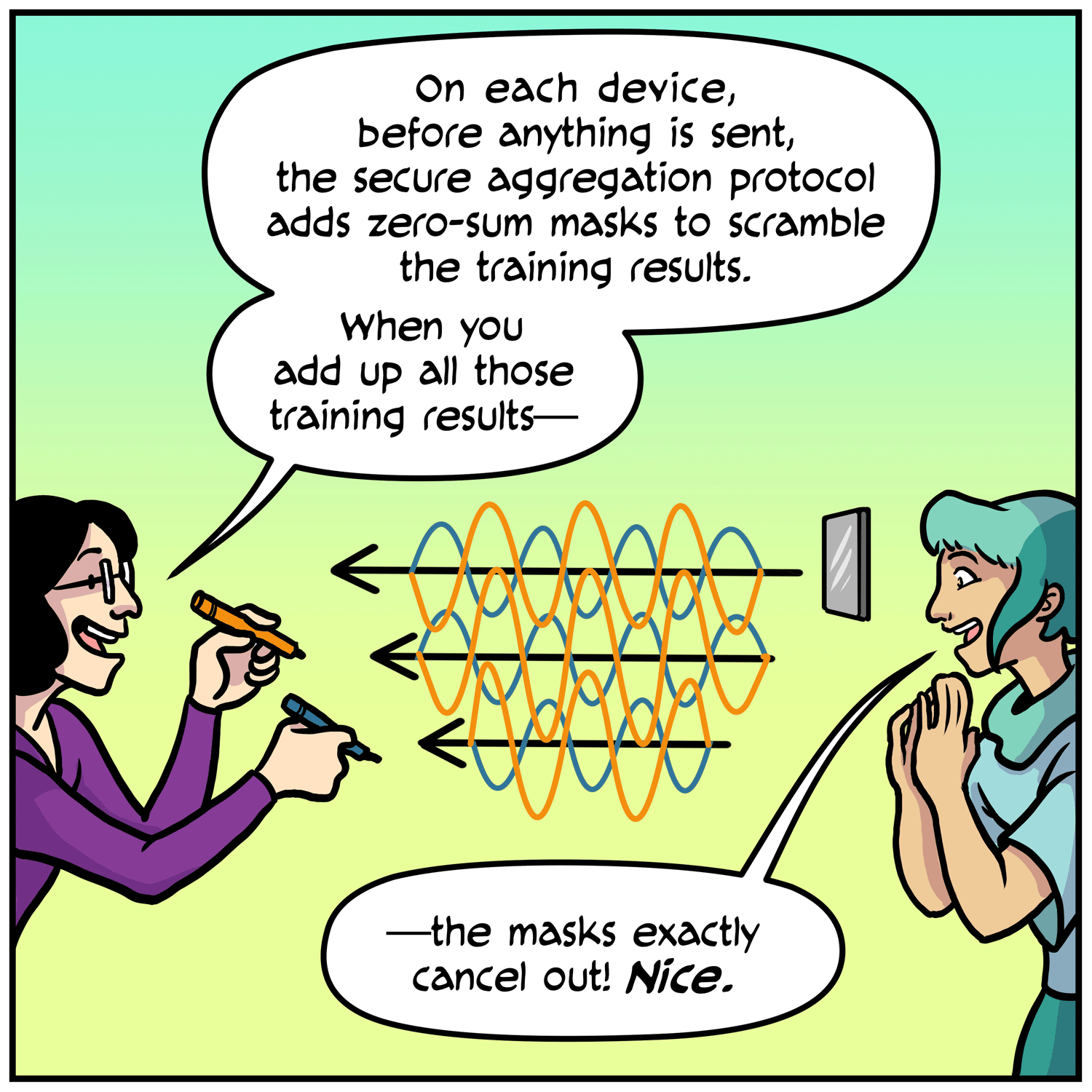
Martha draws wavy lines in two colors across incoming information from a phone, demonstrating zero-sum masking. She says “On each device, before anything is sent, the secure aggregation protocol adds zero-sum masks to scramble the training results. When you add up all those training results” Kai jumps in excitedly and says “the masks exactly cancel out. Nice!”
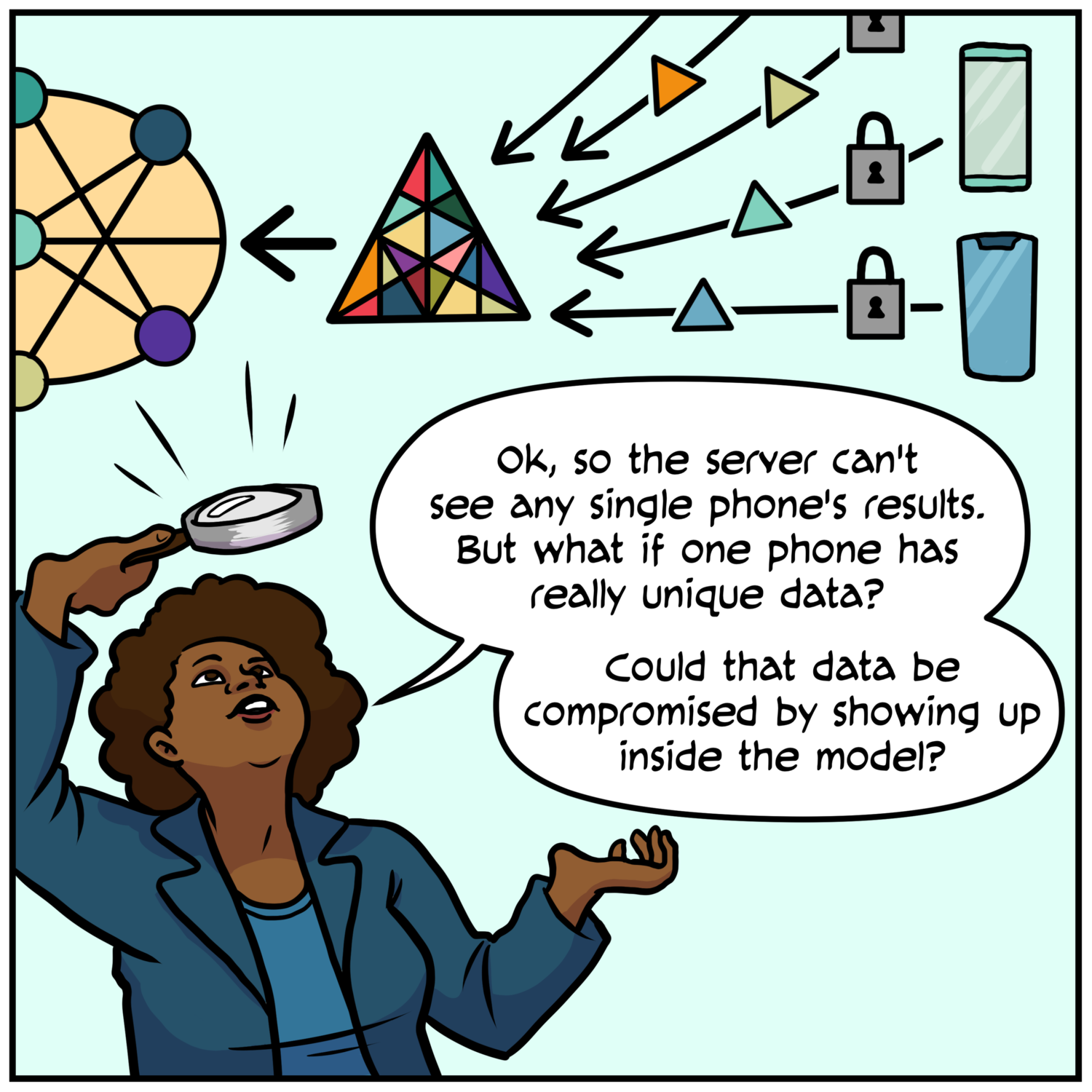
Aliyah points her magnifying glass towards the centralized neural network and says “OK, so the server can’t see any single phone’s result. But what if one phone has really unique data? Could that data be compromised by showing up inside the model?”
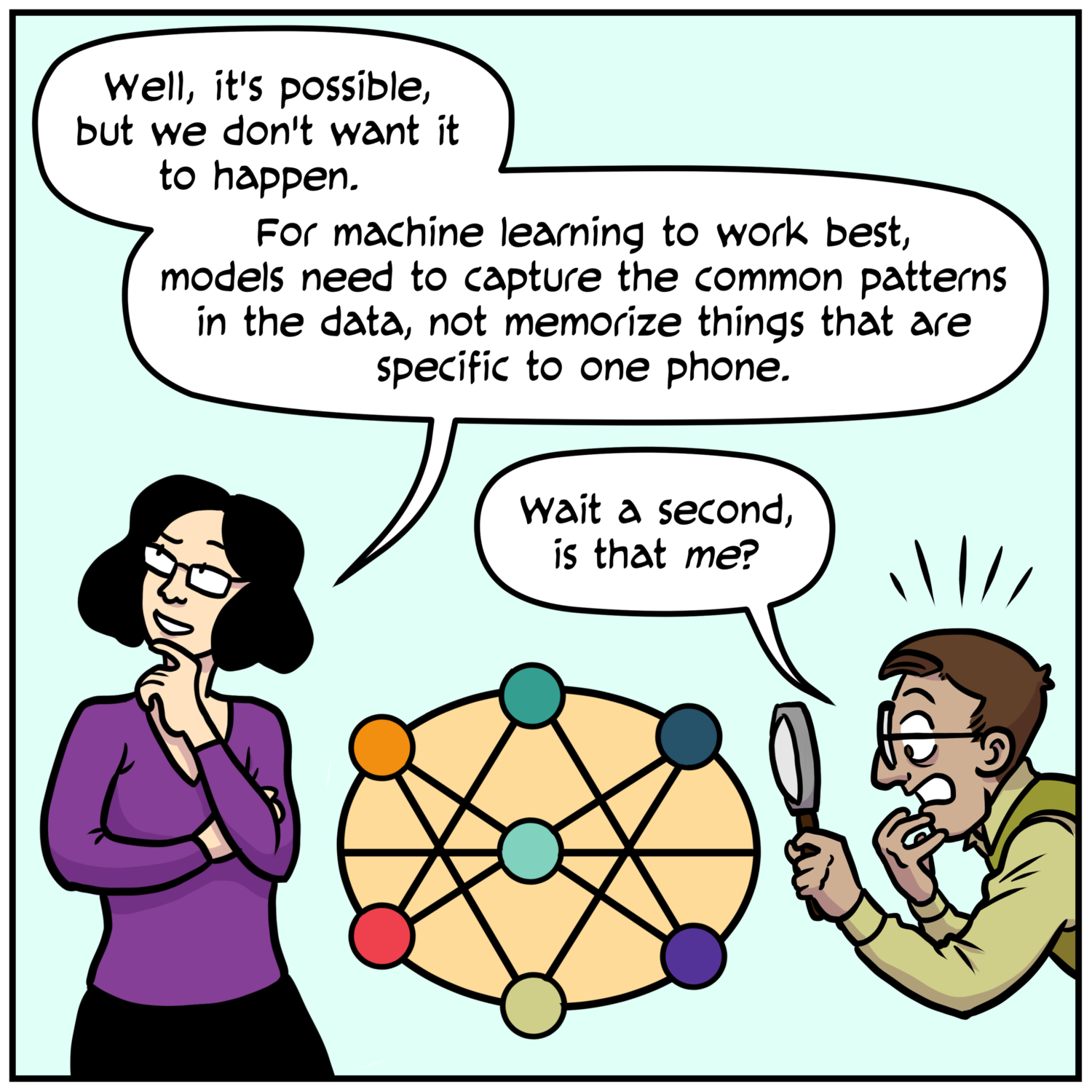
Martha and Mateo stand on opposite sides of the neural network. Martha considers Aliyah’s question thoughtfully, and says “Well, it’s possible, but we don’t want it to happen. For machine learning to work best, models need to capture common patterns in the data, not memorize things that are specific to one phone.” Mateo looks frightened as he inspects the neural network through a magnifying glass, saying “Wait a second, is that me?”
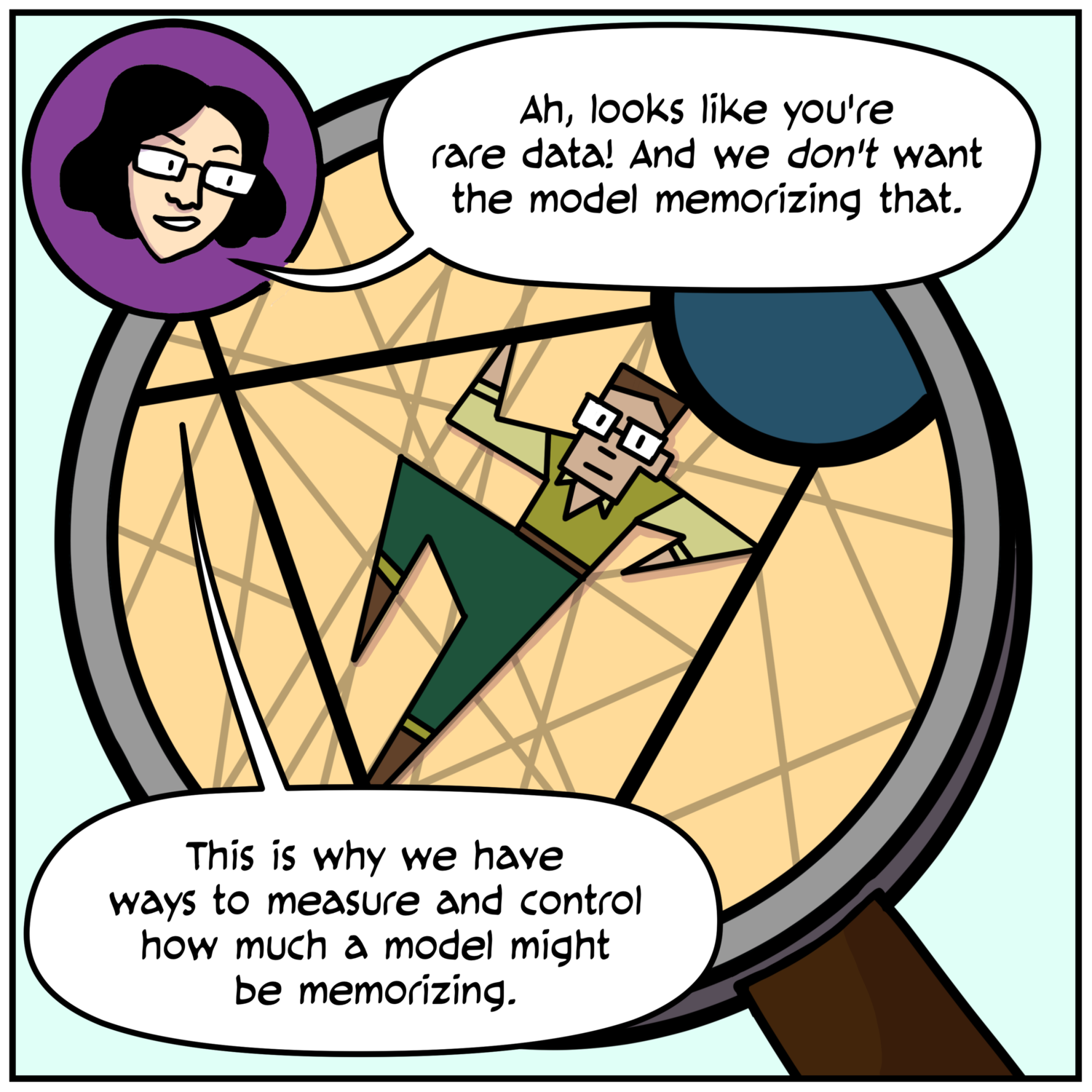
A close-up view of the neural network is shown under a magnifying glass, with a blocky but recognizable Mateo-shaped outline within it, among many background lines. Martha floats to the side of the magnifying glass, looking concerned. She says “Ah, looks like you’re the rare data! And we don’t want the model memorizing that. This is why we have ways to measure and control how much a model might be memorizing.”
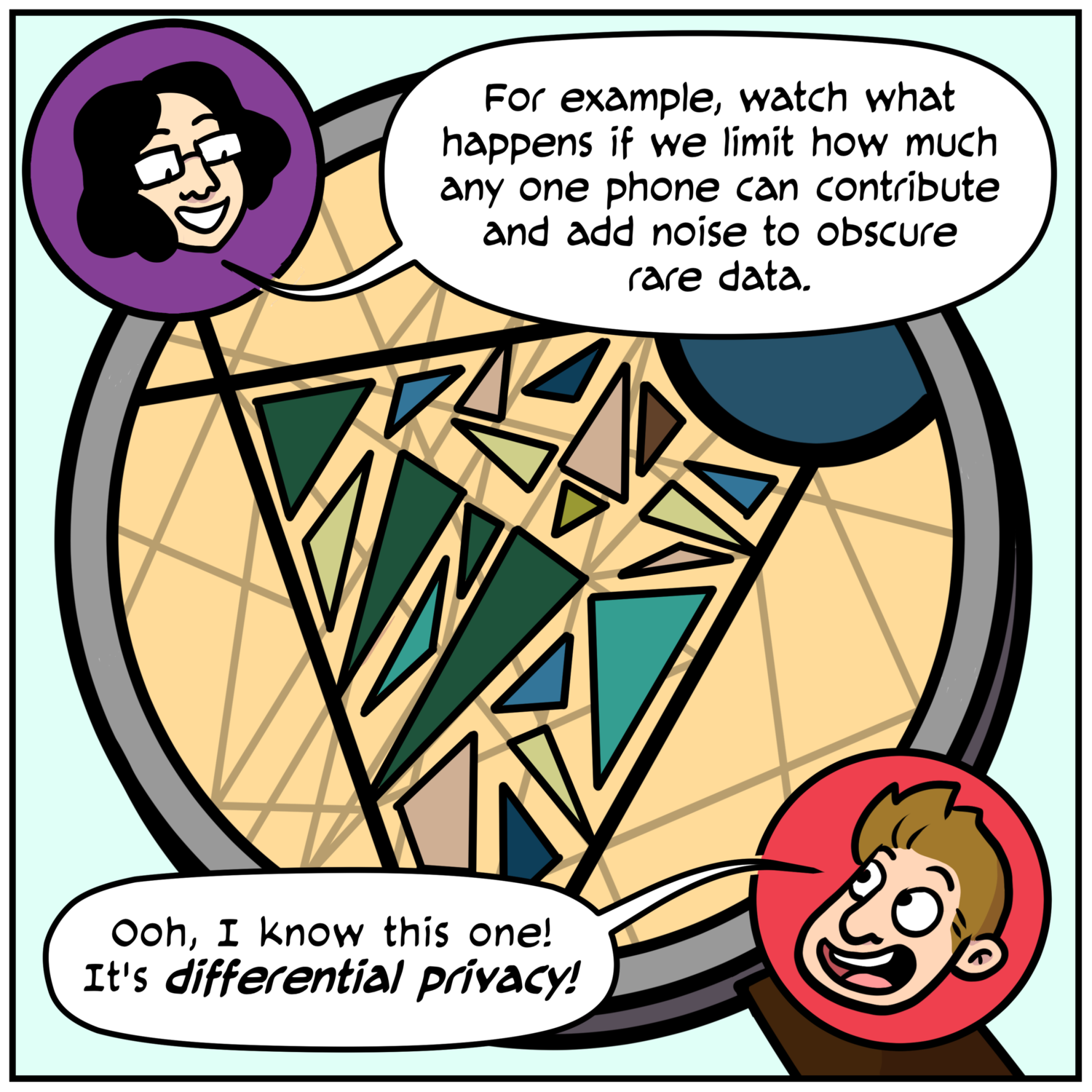
The close-up under the magnifying glass transforms into abstract shapes that are no longer recognizably Mateo. Martha and Brad float on either side of the magnifying glass. Martha happily says, “For example, watch what happens if we limit how much any one phone can contribute and add noise to obscure rare data.” Brad gleefully chimes in “Ooh, I know this one! It’s differential privacy!”
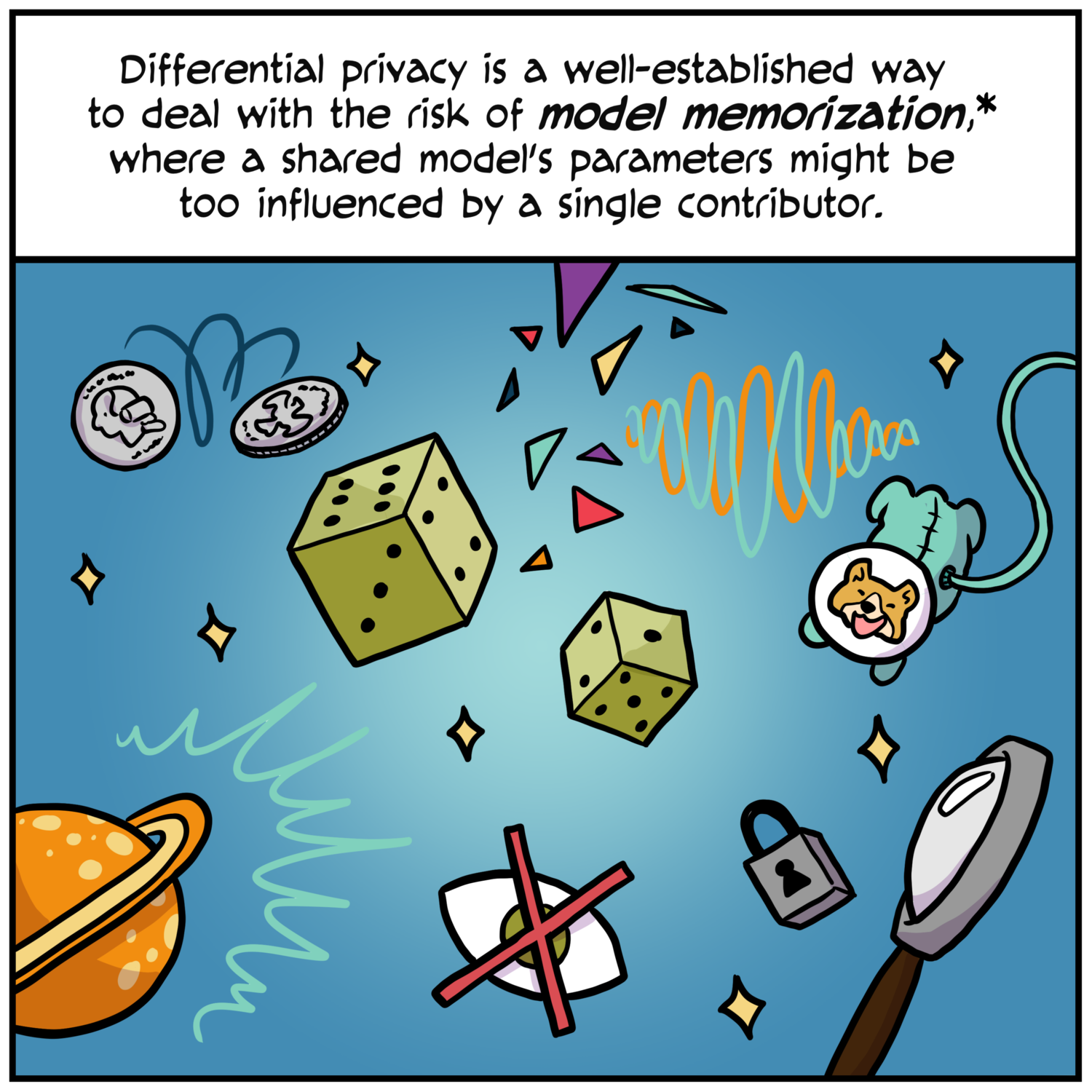
An off-panel narrator says “Differential privacy is a well-established way to deal with the risk of model memorization,* where a shared model’s parameters might be too influenced by a single contributor.”
Understanding and mitigating the risks of model memorization is an active area of research. Techniques to measure memorization are explored, e.g. in the 2018 paper The Secret Sharer: Measuring Unintended Neural Network Memorization & Extracting Secrets. Memorization risk can be mitigated by pre-filtering rare or sensitive information before training. More sophisticated mitigation techniques include differentially private model training as explored, for example, in the 2018 paper Learning Differentially Private Recurrent Language Models, which shows how to learn model weights that are not too dependent on any one device’s data. For more information on differential privacy, the canonical textbook “The Algorithmic Foundations of Differential Privacy” by Cynthia Dwork and Aaron Roth is available from NOW publishers and online.
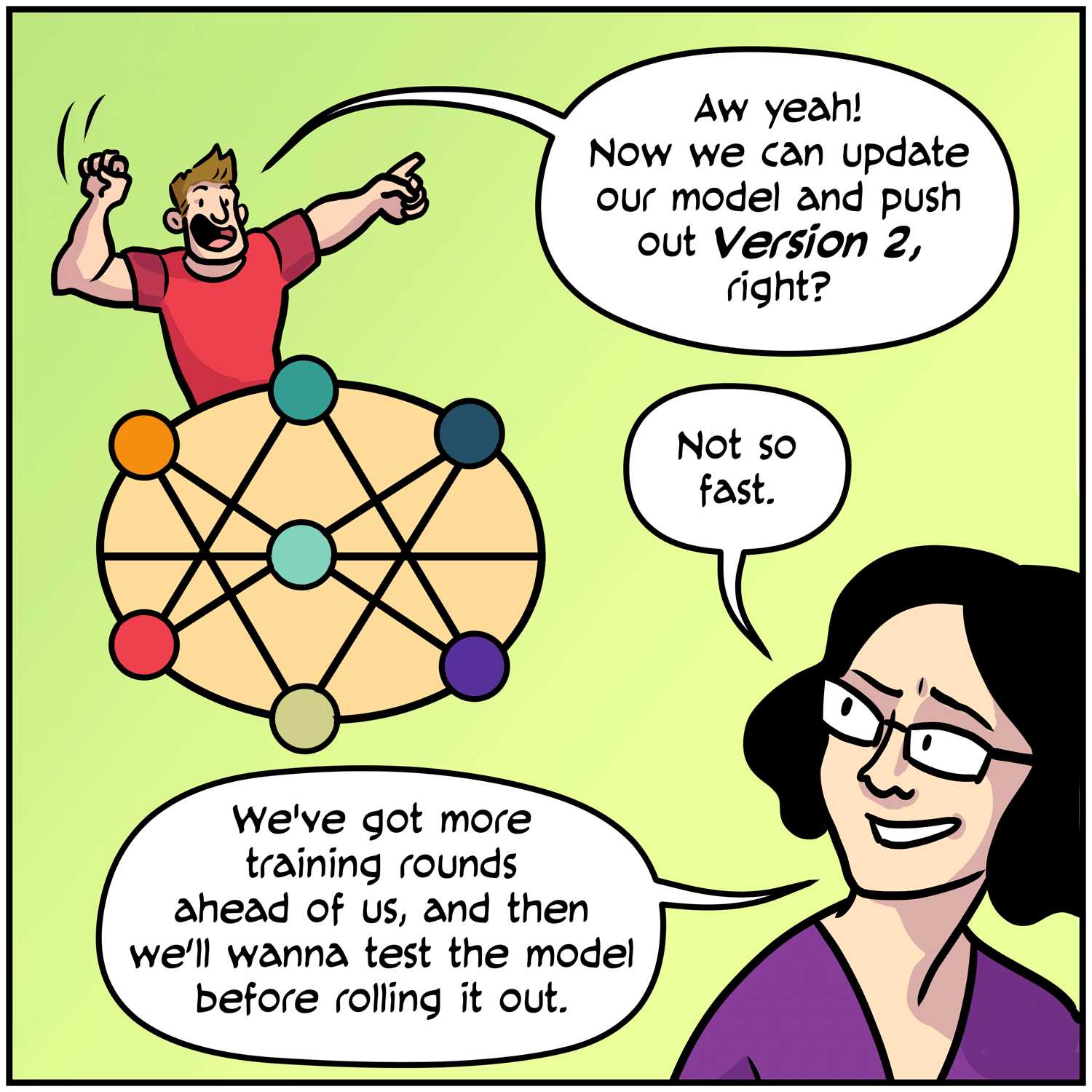
Brad jumps up from behind the large central model, shouting “Aw, yeah! Now we can update our model and push out Version 2, right?” Martha says “Not so fast. We’ve got more training rounds ahead of us, and then we’ll wanna test the model before rolling it out.”
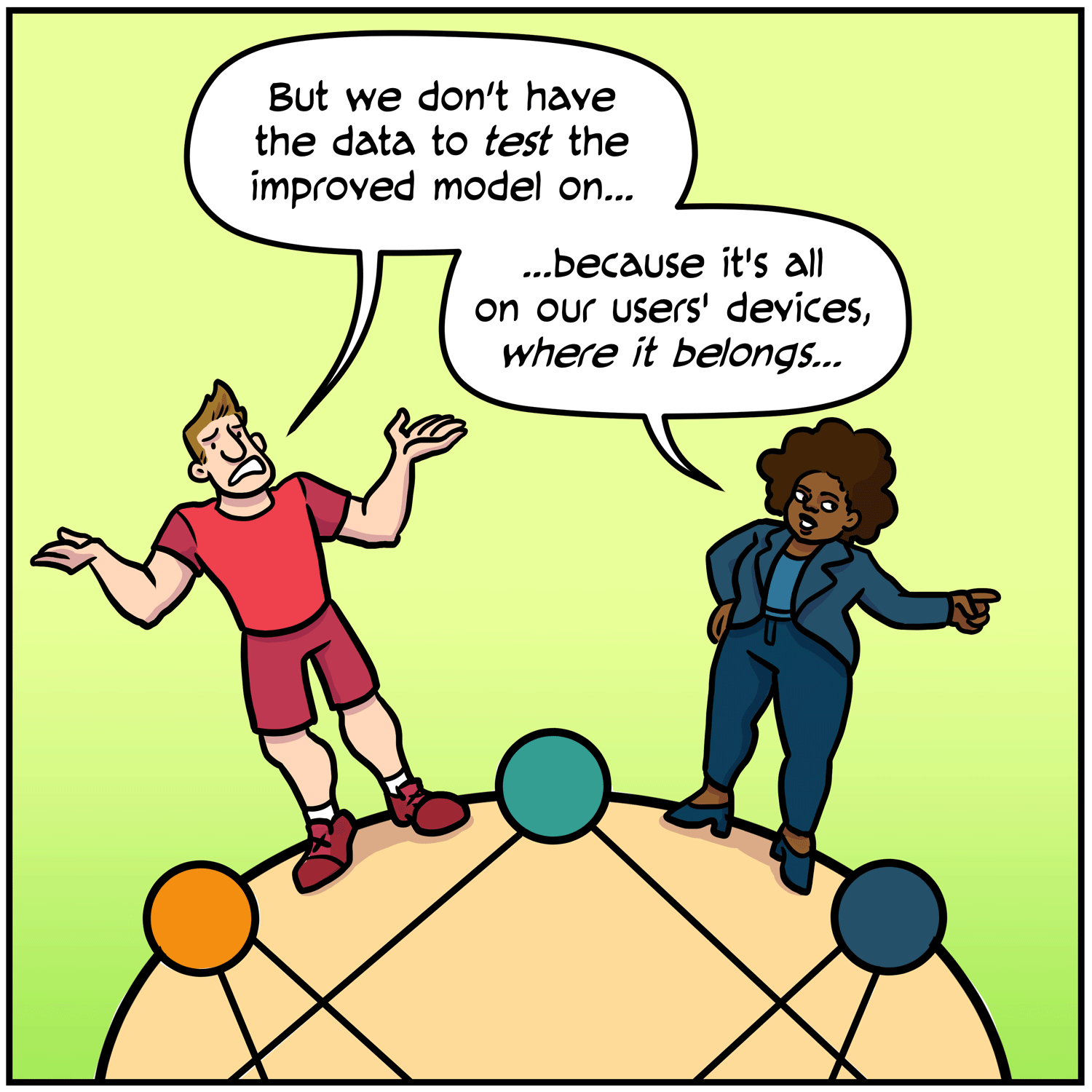
Brad and Aliyah stand on top of the centralized model. Brad says “But we don’t have the data to test the improved model…” Aliyah continues “…because it’s all on the users’ devices, where it belongs…”
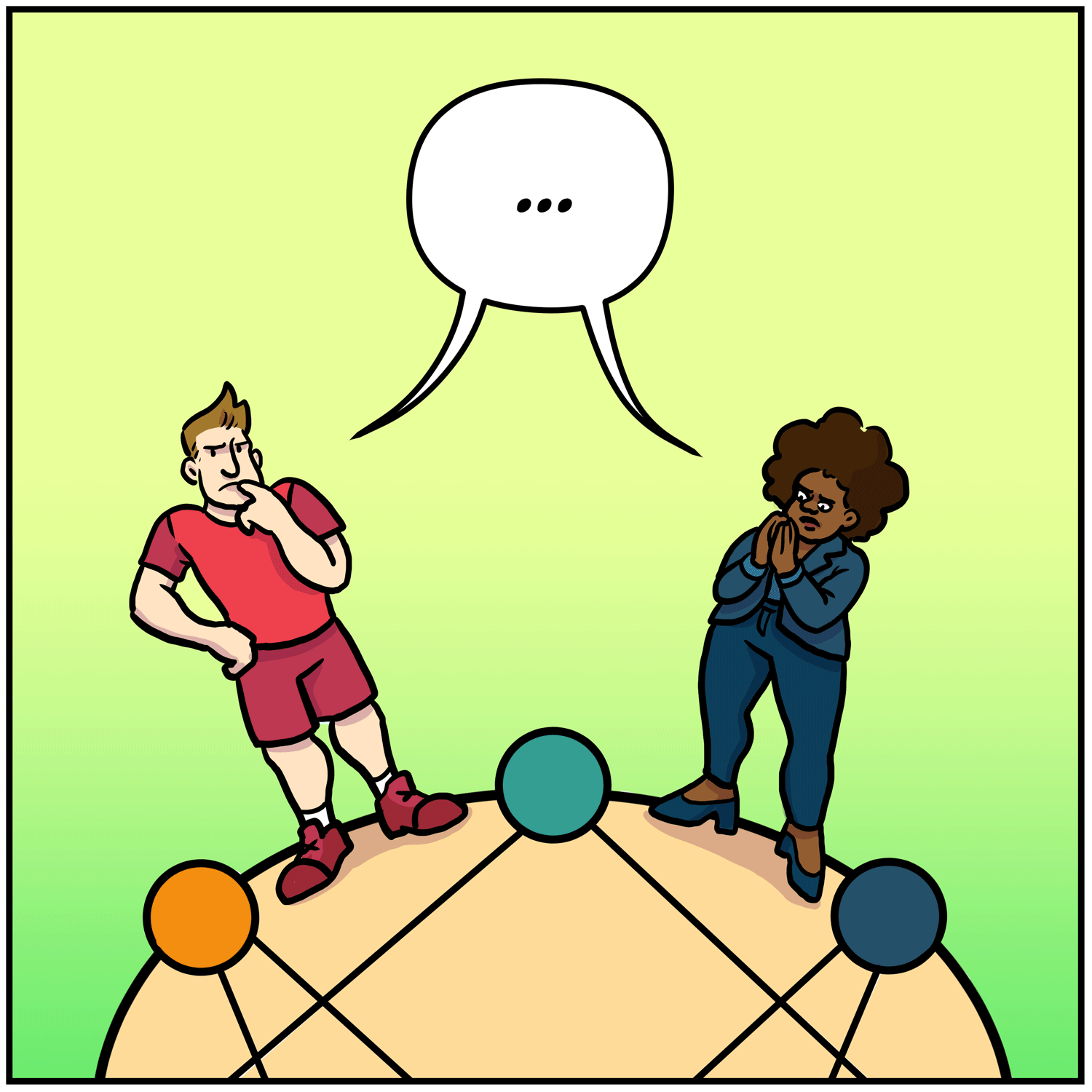
Brad and Aliyah think for a moment.
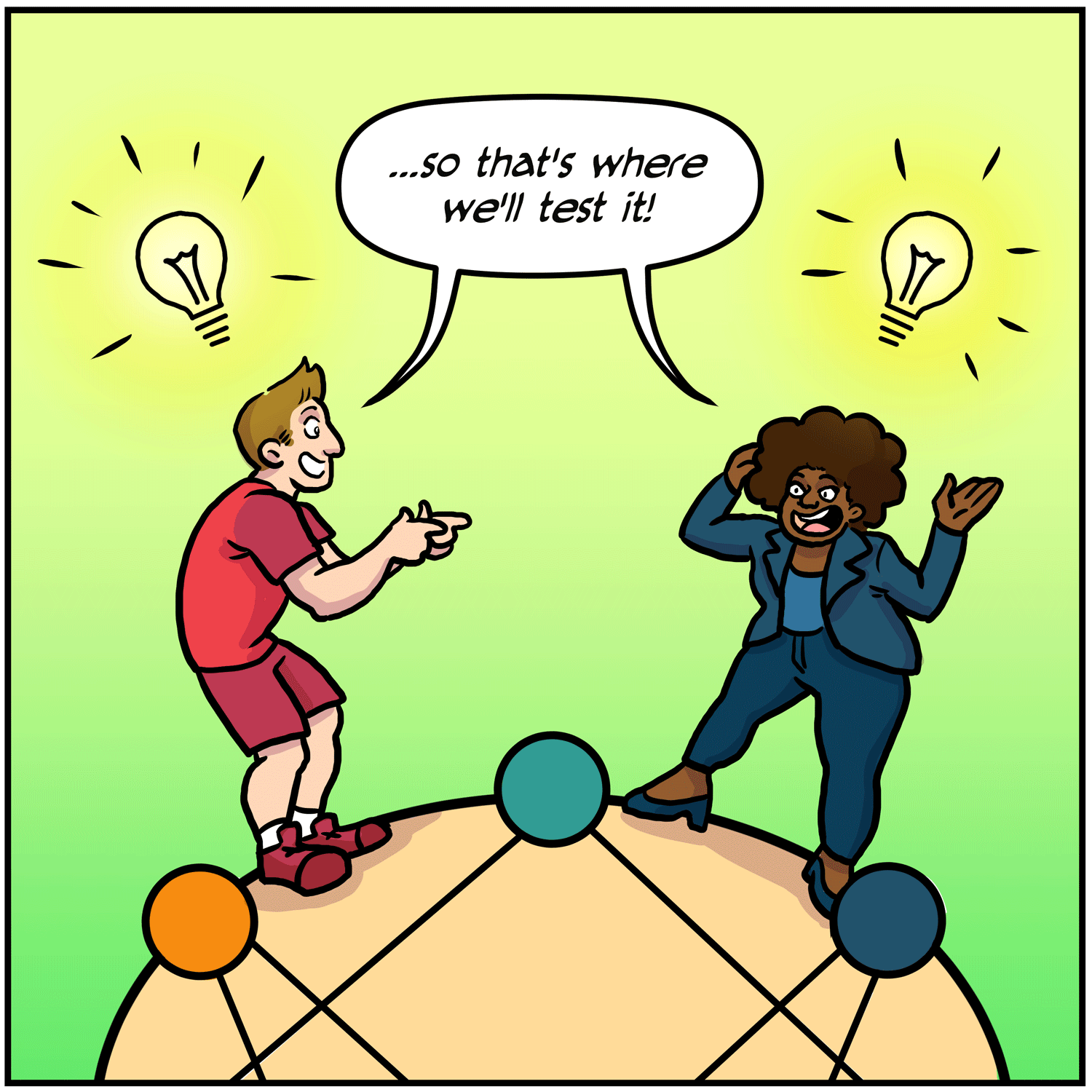
Light bulbs appear over Brad and Aliyah’s heads. They look at each other and shout “so that’s where we’ll test it!”
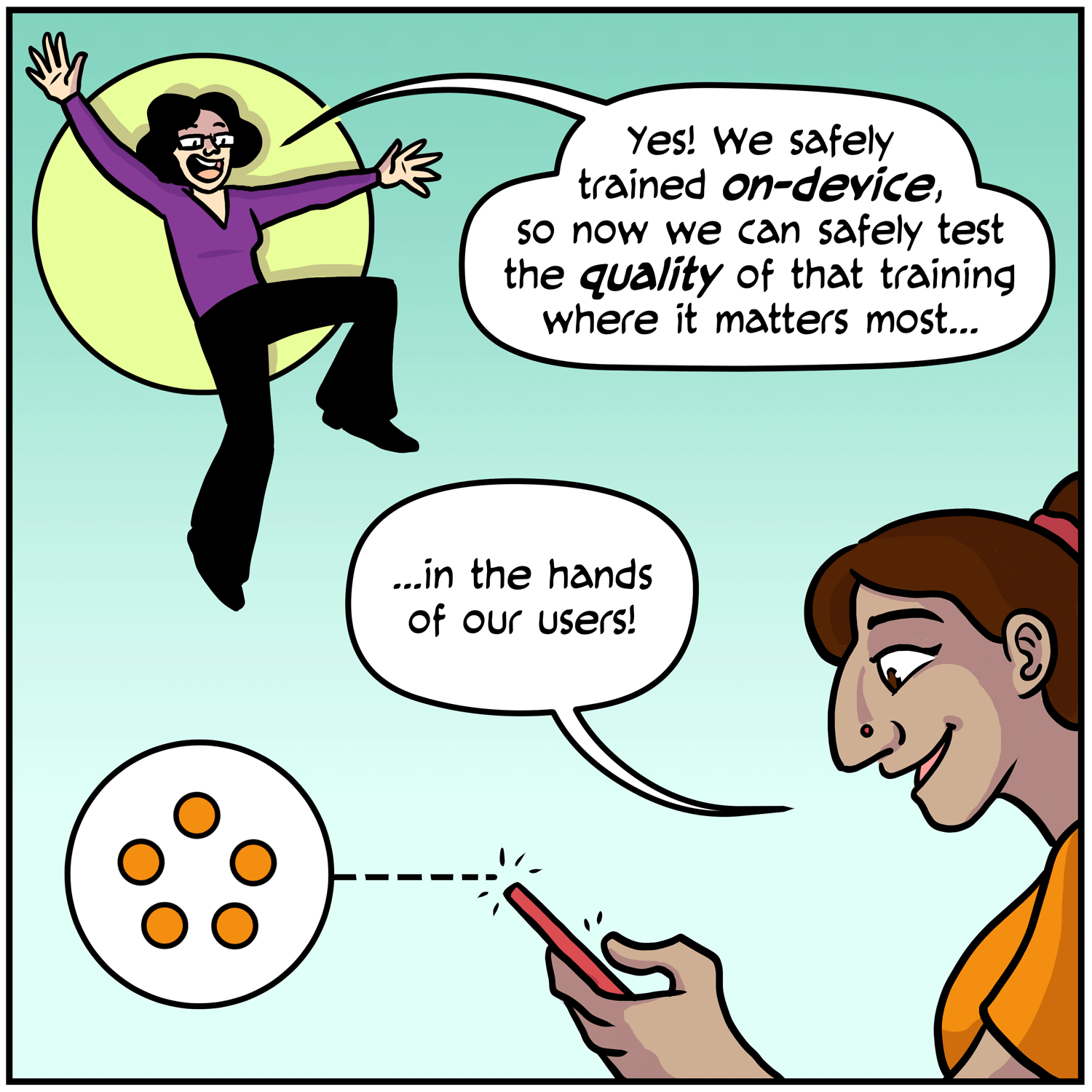
Martha appears and says “Yes! We safely trained on-device, so now we can safely test the quality of that training where it matters most… Devi holds her phone, which is receiving a copy of the small training model. She says “In the hands of our users!”
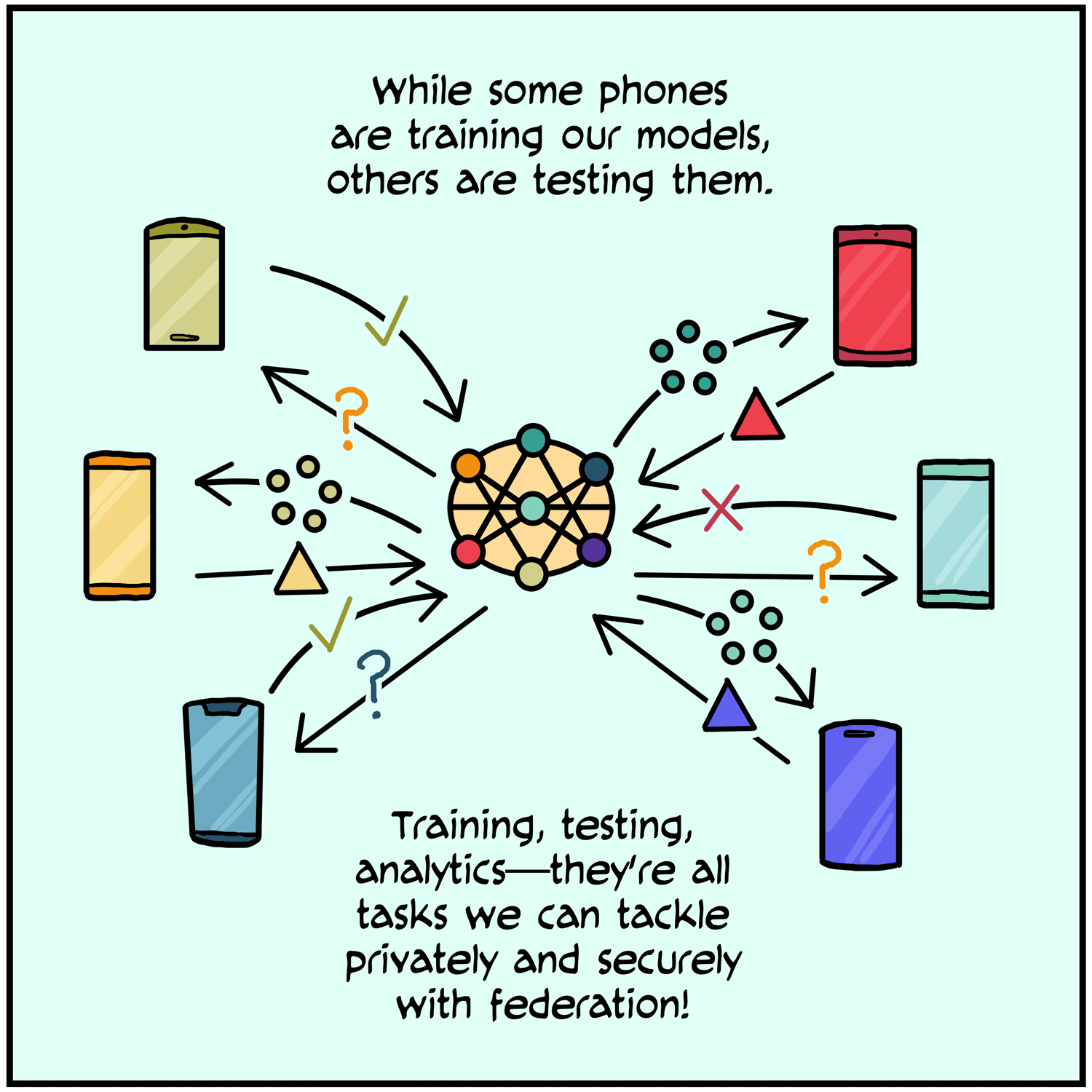
The central neural network model sits in a circle of phones, querying some of them, sending models to others. It’s a busy hive of training activity. Martha narrates “While some phones are training our models, others are testing them. Training, testing, analytics – they’re all tasks we can tackle privately and securely with federation!”
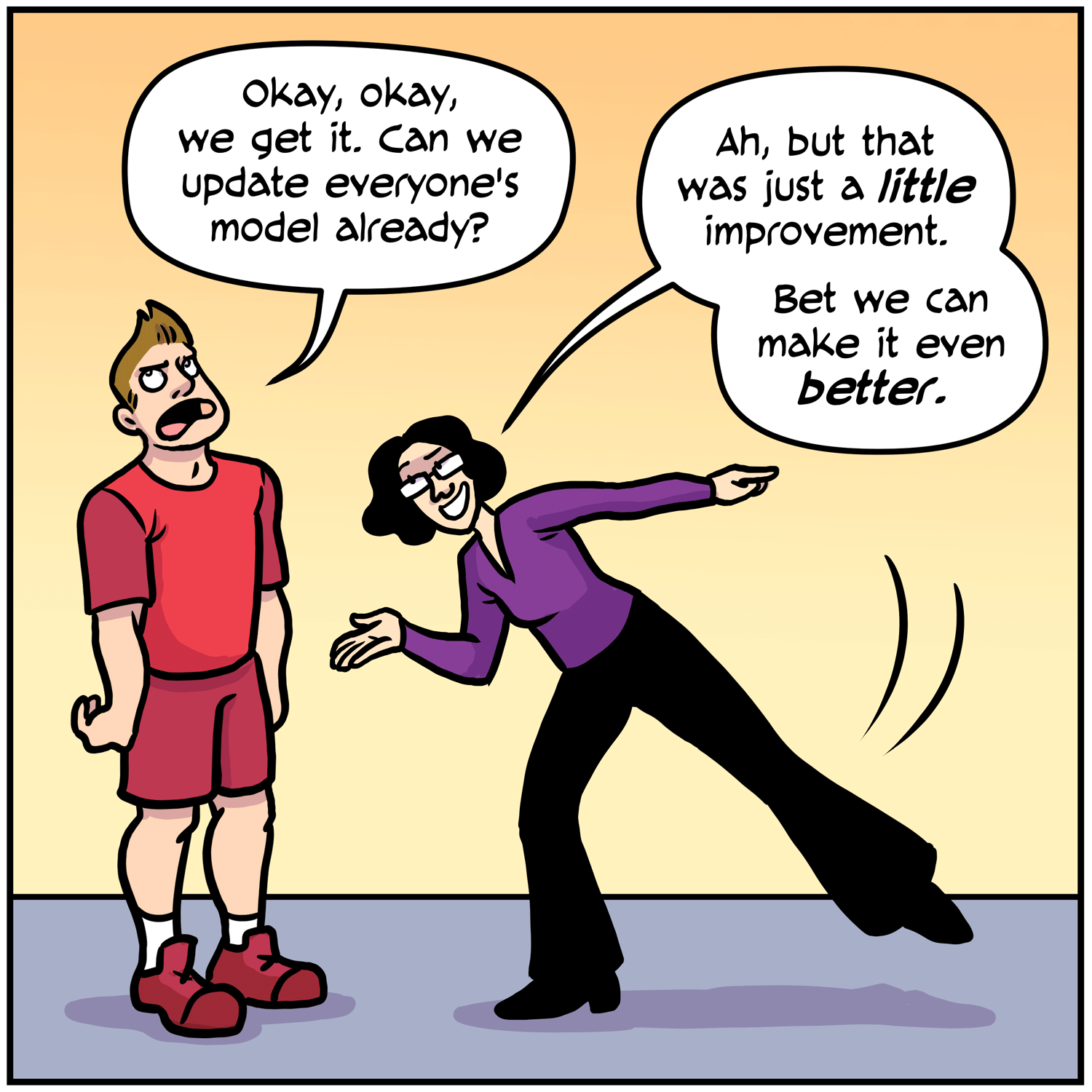
Back in the conference room, Brad becomes impatient. “Okay, okay, we get it,” he groans. “Can we update everyone’s model already?” Martha leans over and grins at him. “Ah,” she says, “but that was just a little improvement. Bet we can make it even better.”
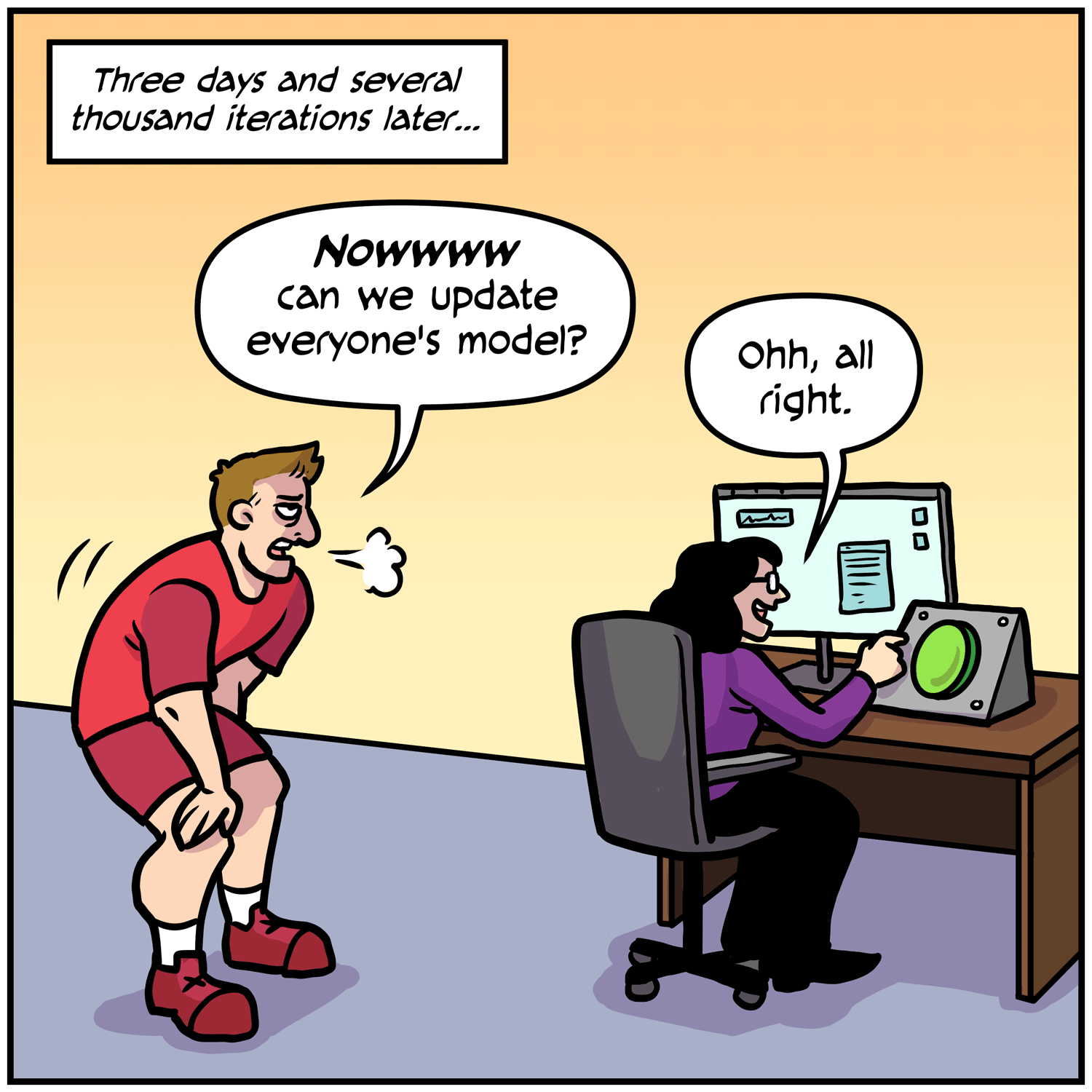
A caption reads “Three days and several thousand iterations later…” Brad is doubled over, exhausted. “Nowwww can we update everyone’s model?” he asks. Martha grins at him from her computer, lifting her hand to press a large green button. “Ohh, all right,” she says.
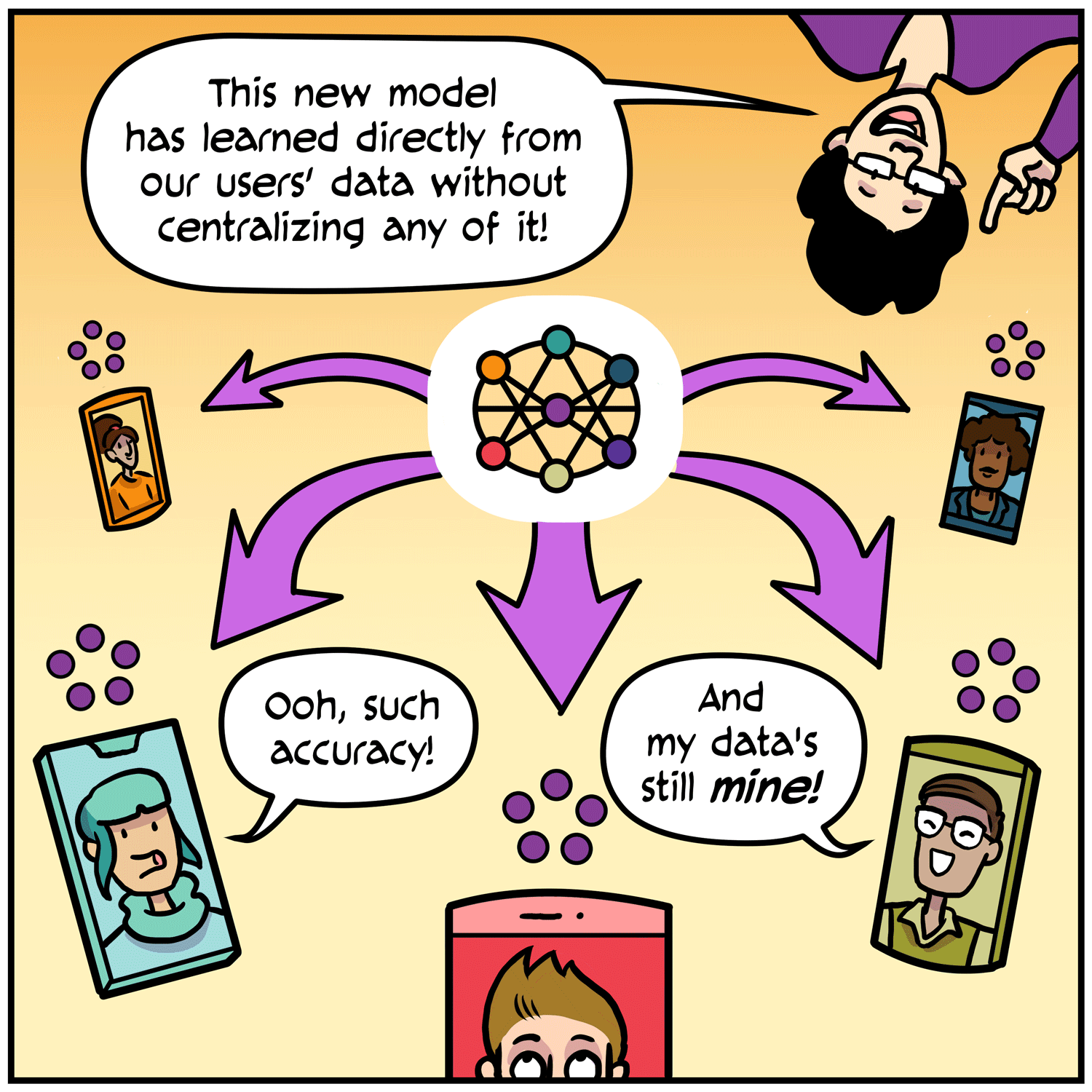
Back in diagram-world, a central model floats in the middle of the panel, with five arrows pointing out to the five interns’ phones. A small neural model, now purple instead of yellow, floats above each phone. The interns all appear within their phone screens. Martha says “This new model has learned directly from our user’s data without centralizing any of it!” Kai coos “Ooh, such accuracy.” Mateo cheers “And my data’s still mine!”
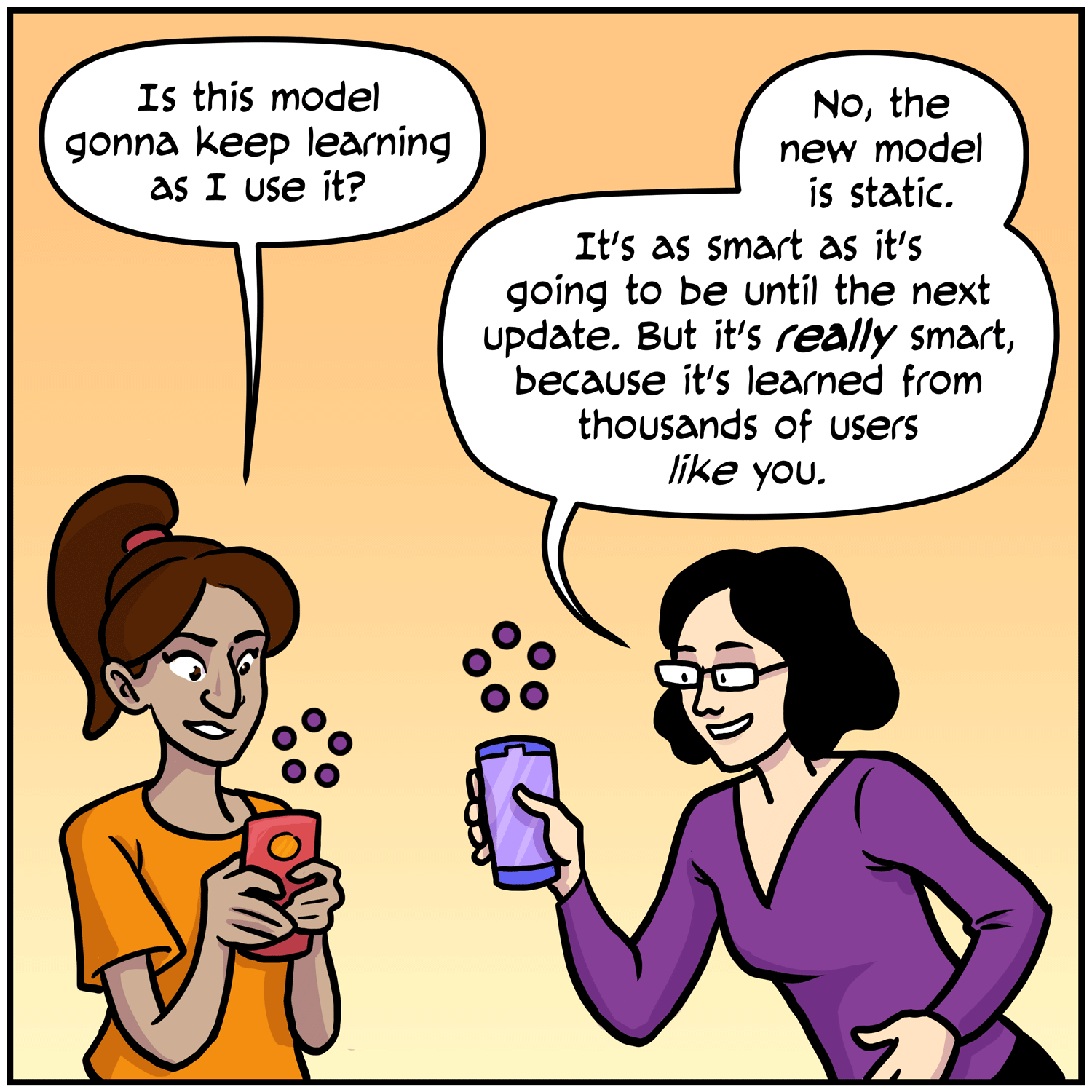
Devi looks at her phone and asks “Is this model going to keep learning as I use it?” “No,” says Martha, “the new model is static. It’s as smart as it’s going to be until the next update. But it’s really smart, because it’s learned from thousands of users like you.”
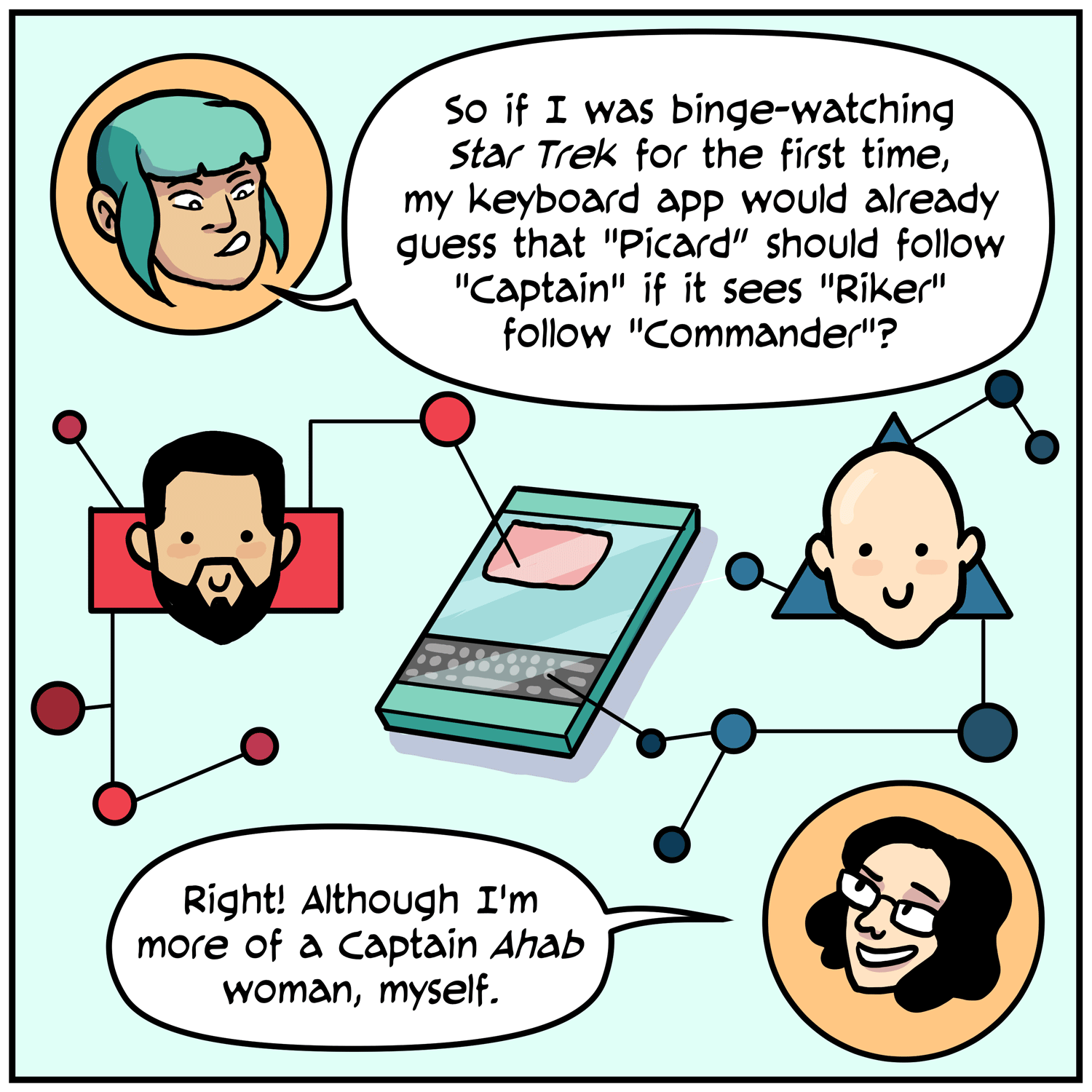
A smartphone appears with little networks of association on either side of it. To the left is a tiny icon of Commander Riker from Star Trek, to the right is Captain Picard. Kai says “So if I was binge-watching Star Trek for the first-time, my keyboard app would already guess that “Picard”: should follow “Captain” every time “Riker” follows “Commander”?” Martha says “Right! Although I’m more of a Captain Ahab woman, myself.”
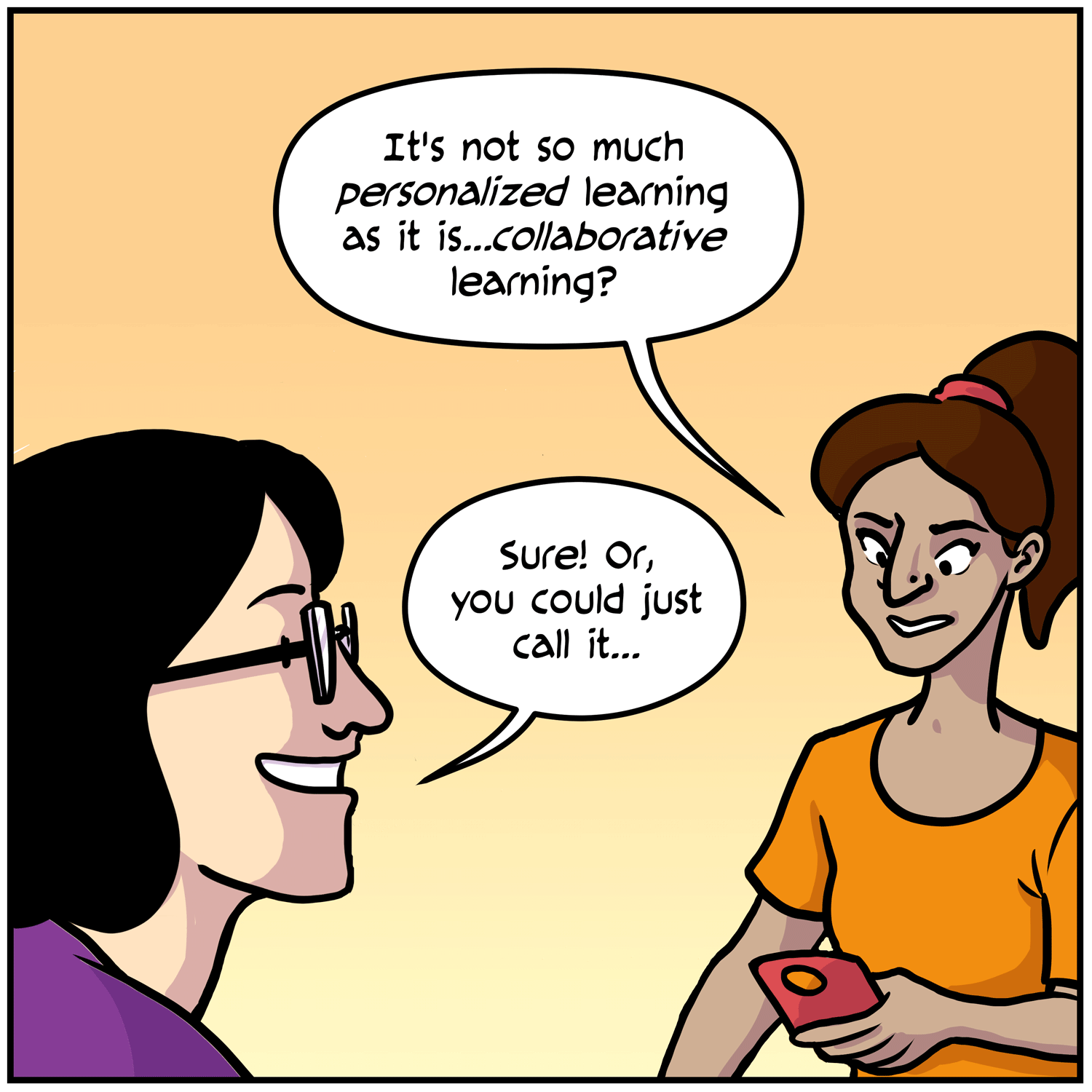
Devi looks thoughtful and says “It’s not so much personalized learning as it is...collaborative learning? Martha smiles and says “Sure! Or, you could just call it…”
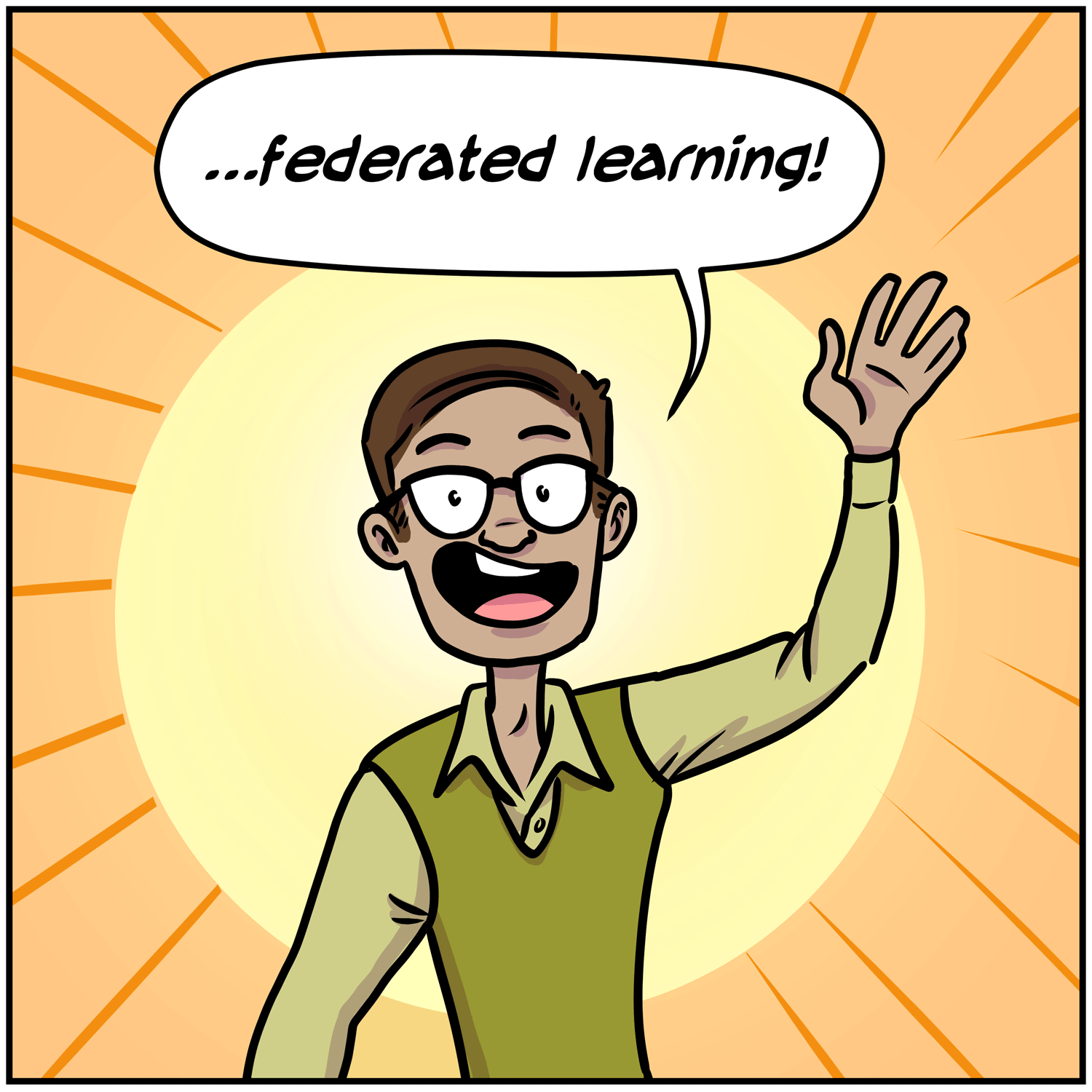
Mateo triumphantly shouts “Federated learning!”
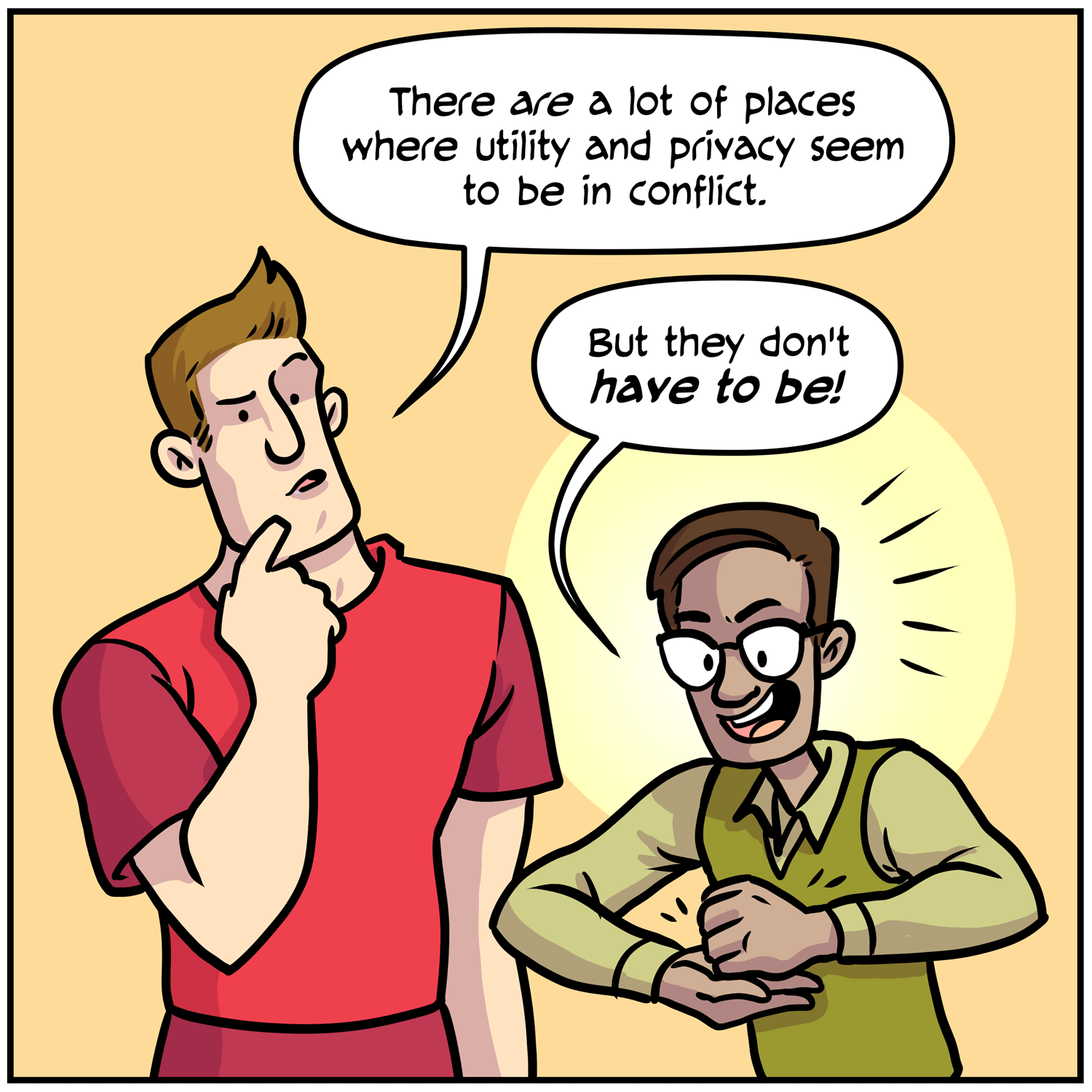
Brad looks thoughtful and says “There are a lot of places where utility and privacy seem to be in conflict.” Mateo looks determined in the foreground, saying “But they don’t have to be!”
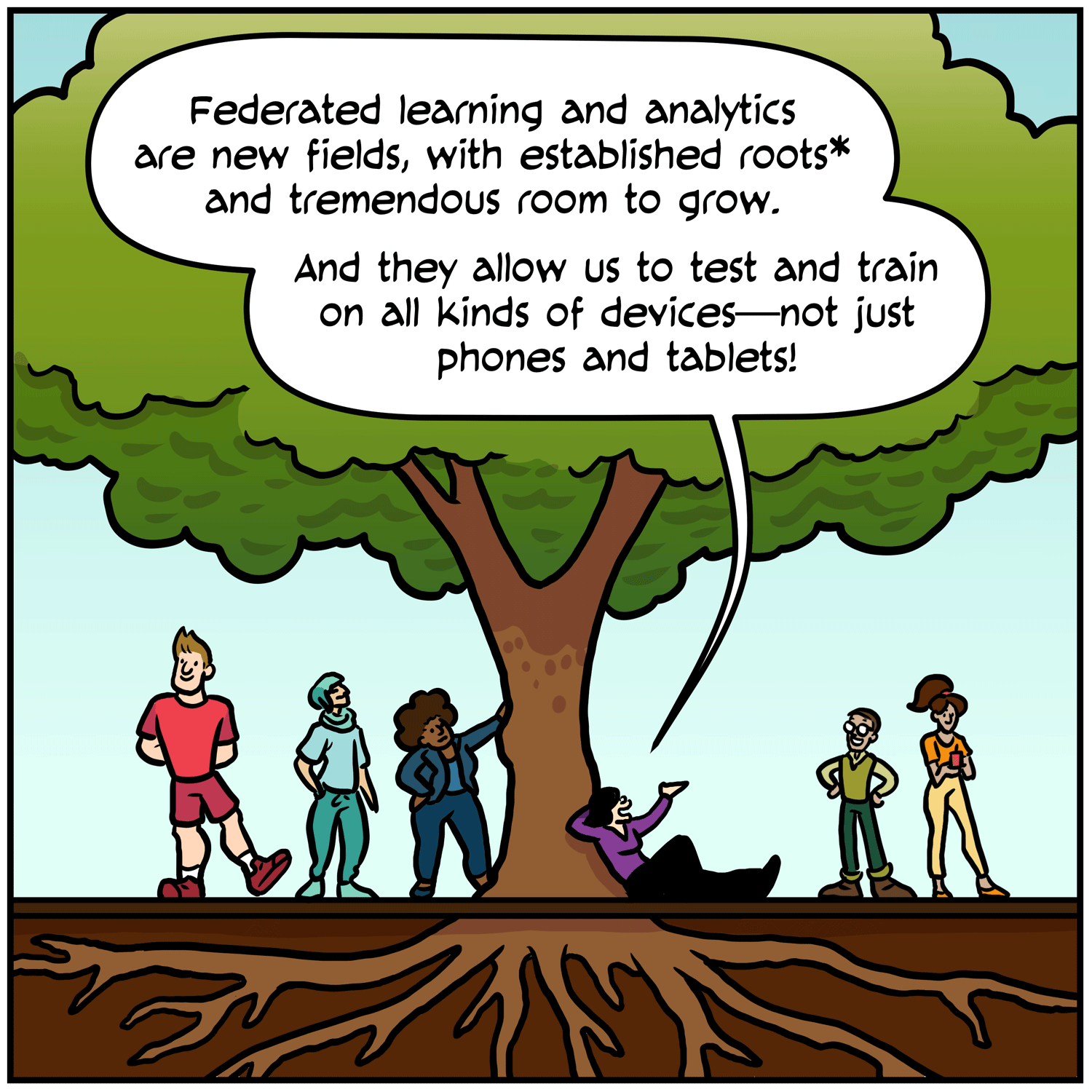
Martha and the interns appear under a large tree, with equally large roots exposed beneath the ground. Martha leans against the trunk while the interns all look up at the branches and enjoy the shade. Martha says “Federated learning and analytics are new fields, with established roots* and tremendous room to grow. And they allow us to test and train on all kinds of devices–not just phones and tablets!”
Federated learning and analytics come from a rich heritage of distributed optimization, machine learning and privacy research. They are inspired by many systems and tools, including MapReduce for distributed computation, TensorFlow for machine learning and RAPPOR for privacy-preserving analytics. The federated learning approach for training deep networks was first articulated in a 2016 paper published by Google AI researchers: Communication-Efficient Learning of Deep Networks from Decentralized Data.
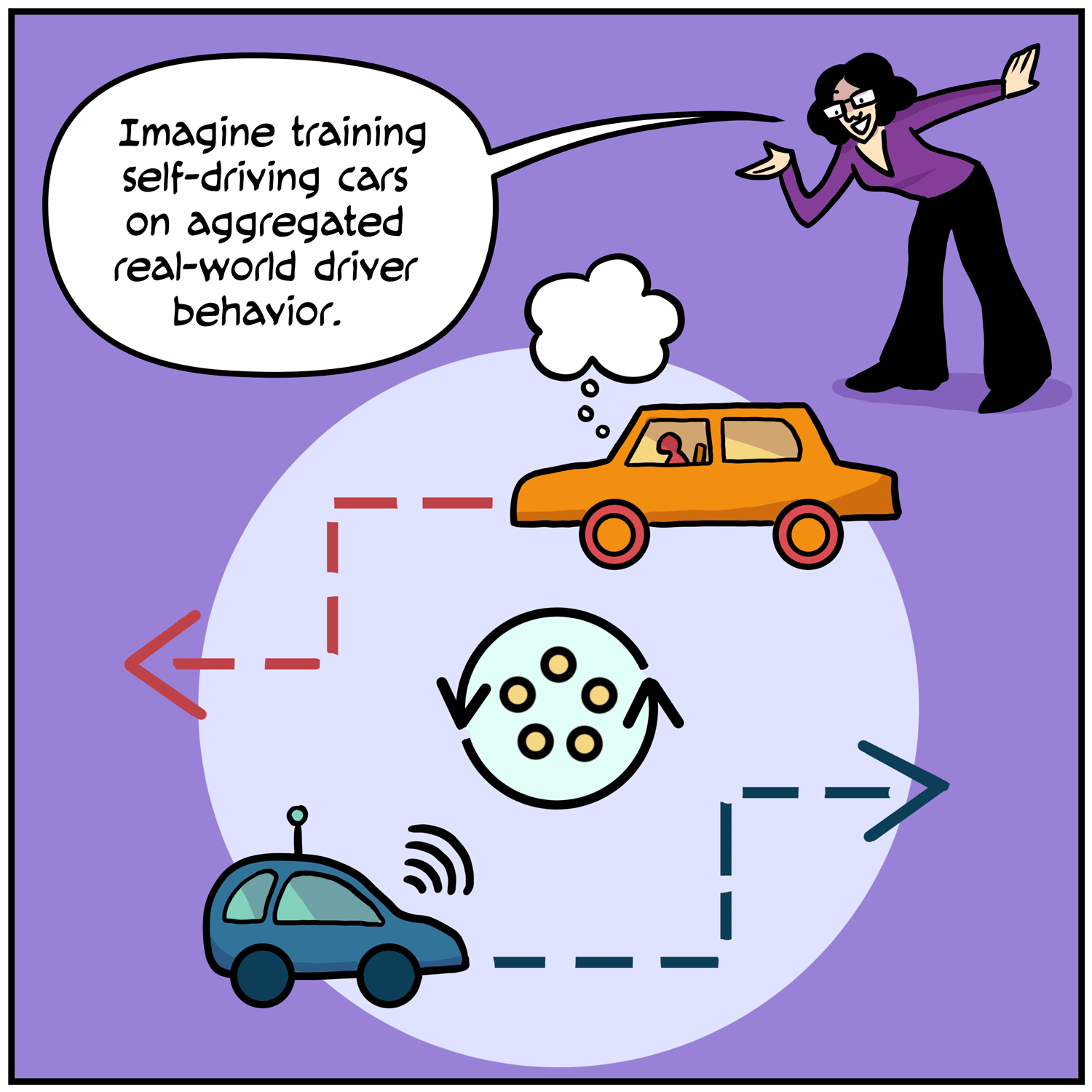
Martha leans over two iconographic cars, one driven by a person and one driven by AI. They circle a small training model. Martha says “Imagine training self-driving cars on aggregated real-world driver behavior.”
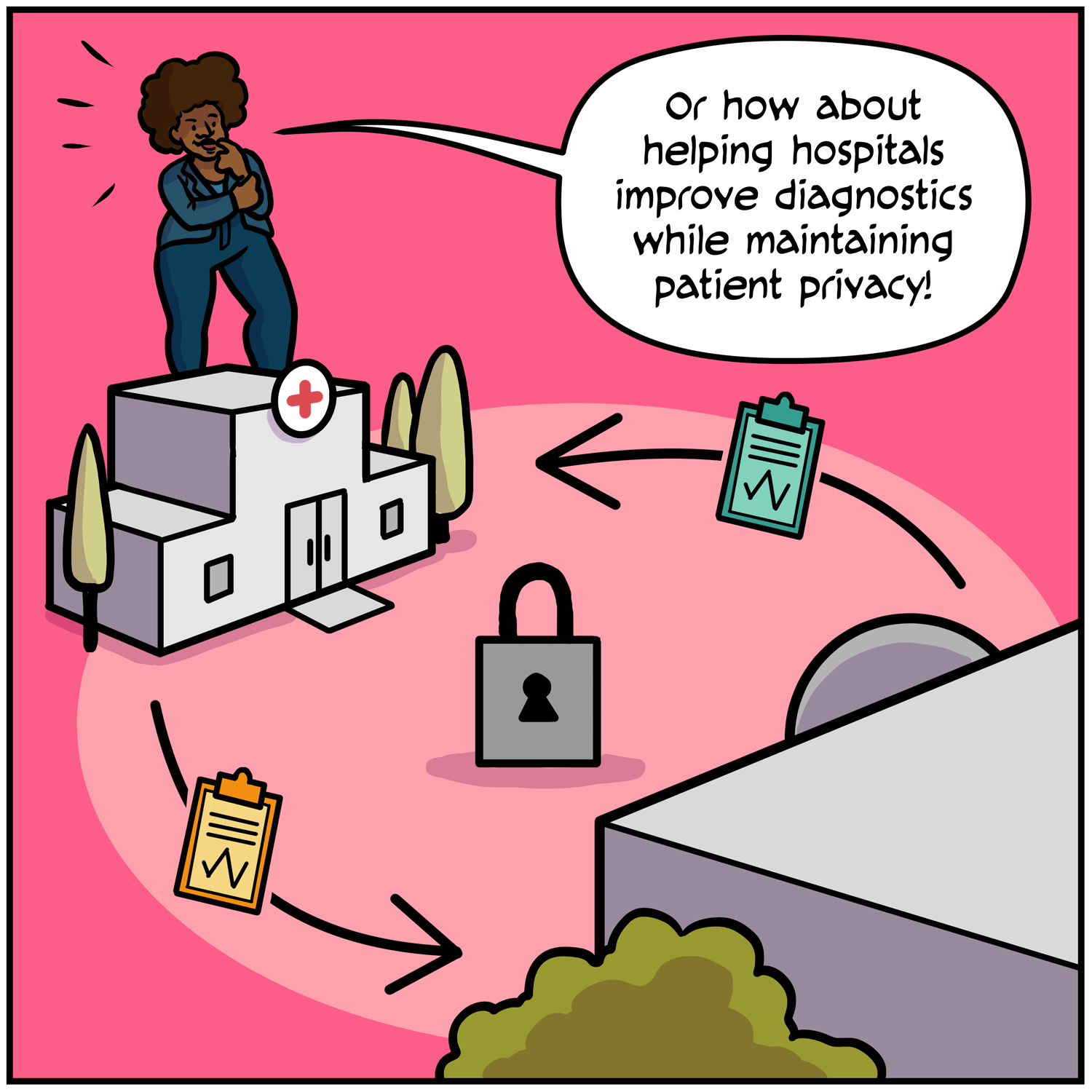
Aliyah strokes her chin while looking at two hospital buildings. A training model sits between them. Arrows show clipboards of information circulating between the buildings. Aliyah says “Or how about helping hospitals improve diagnostics while maintaining patient privacy!”
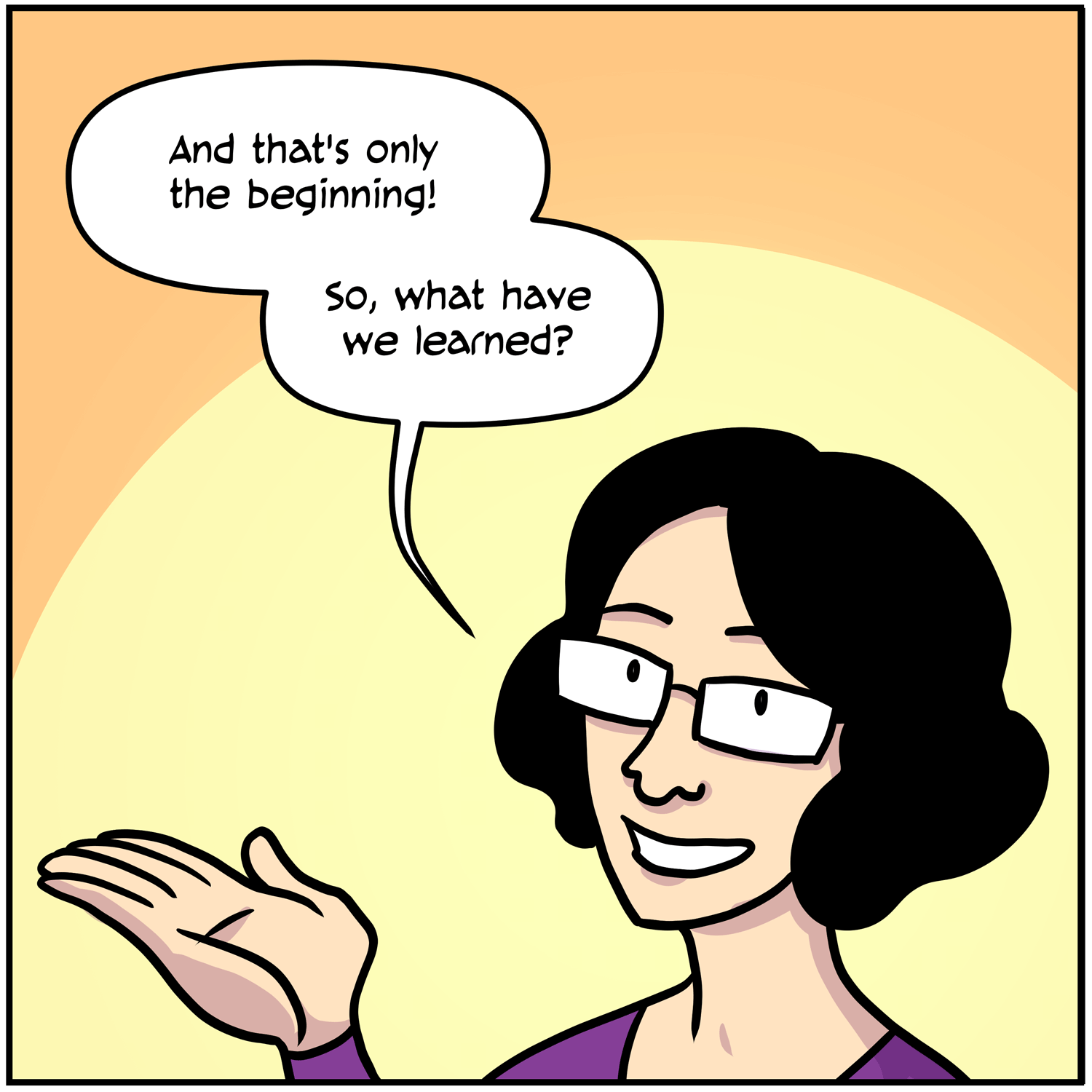
Martha beams and says “And that’s only the beginning! So, what have we learned?”
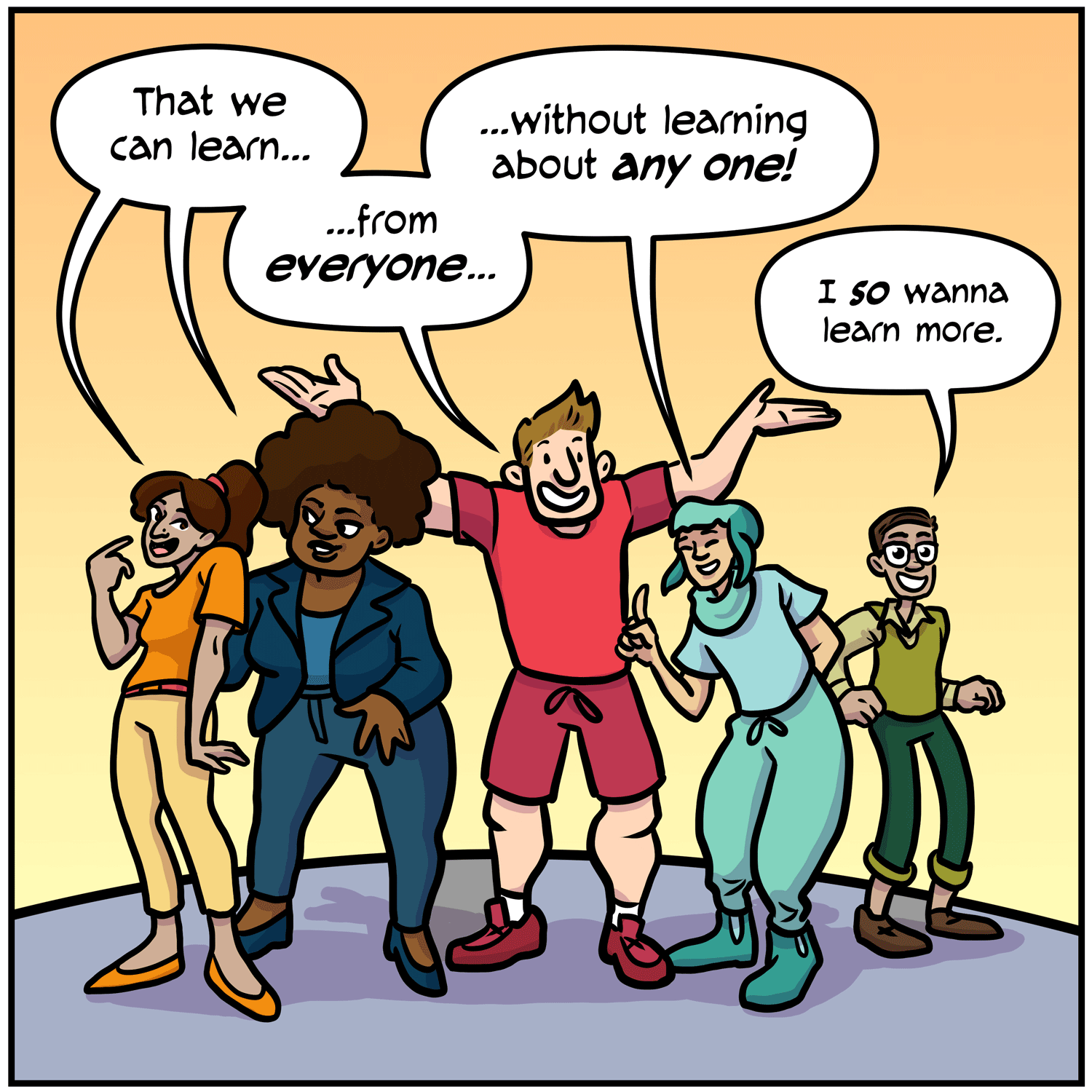
The interns stand together and all chime in to say: “That we can learn from everyone without learning about any one!” Mateo looks wide-eyed with excitement and says “I so wanna learn more!”
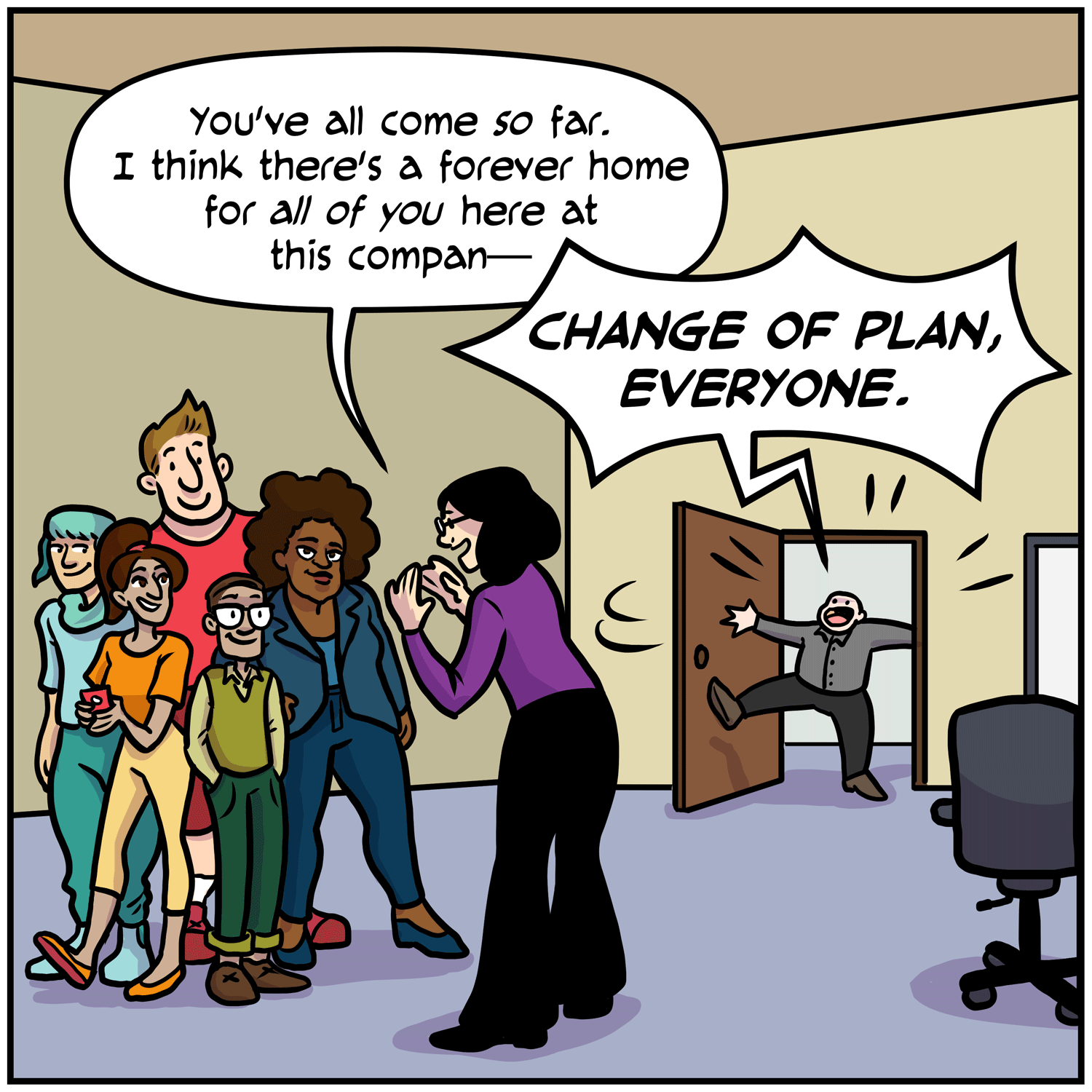
Martha regards the interns proudly and says “You’ve all come so far. I think there’s a forever home for all of you here at this compan-” The Boss bursts through the door to the conference room and shouts “Change of plan, everyone!”
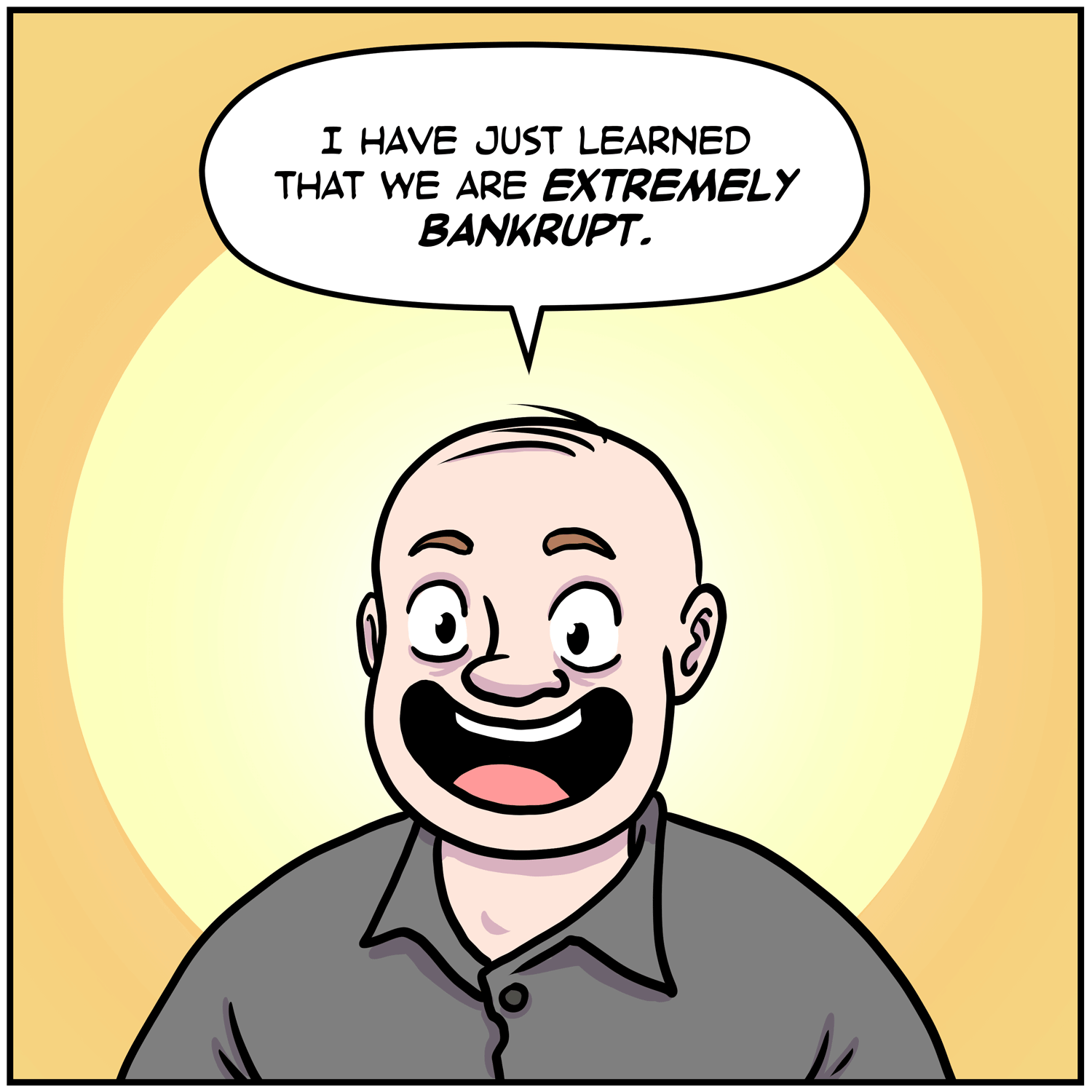
Closeup on the Boss, beaming like a maniac. He says “I have just learned that we are extremely bankrupt.”
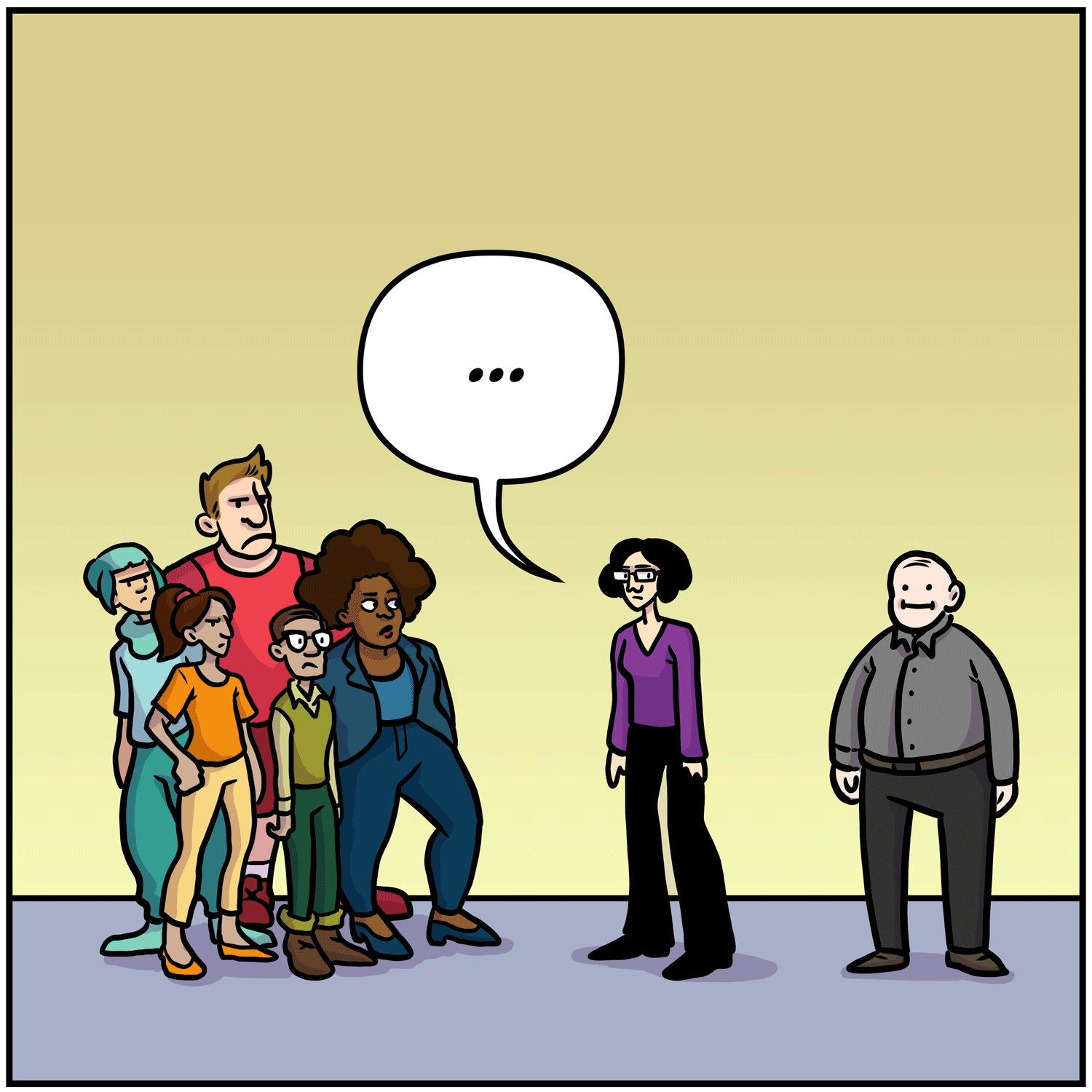
The interns and Martha all stare at the Boss. He looks at them blankly.
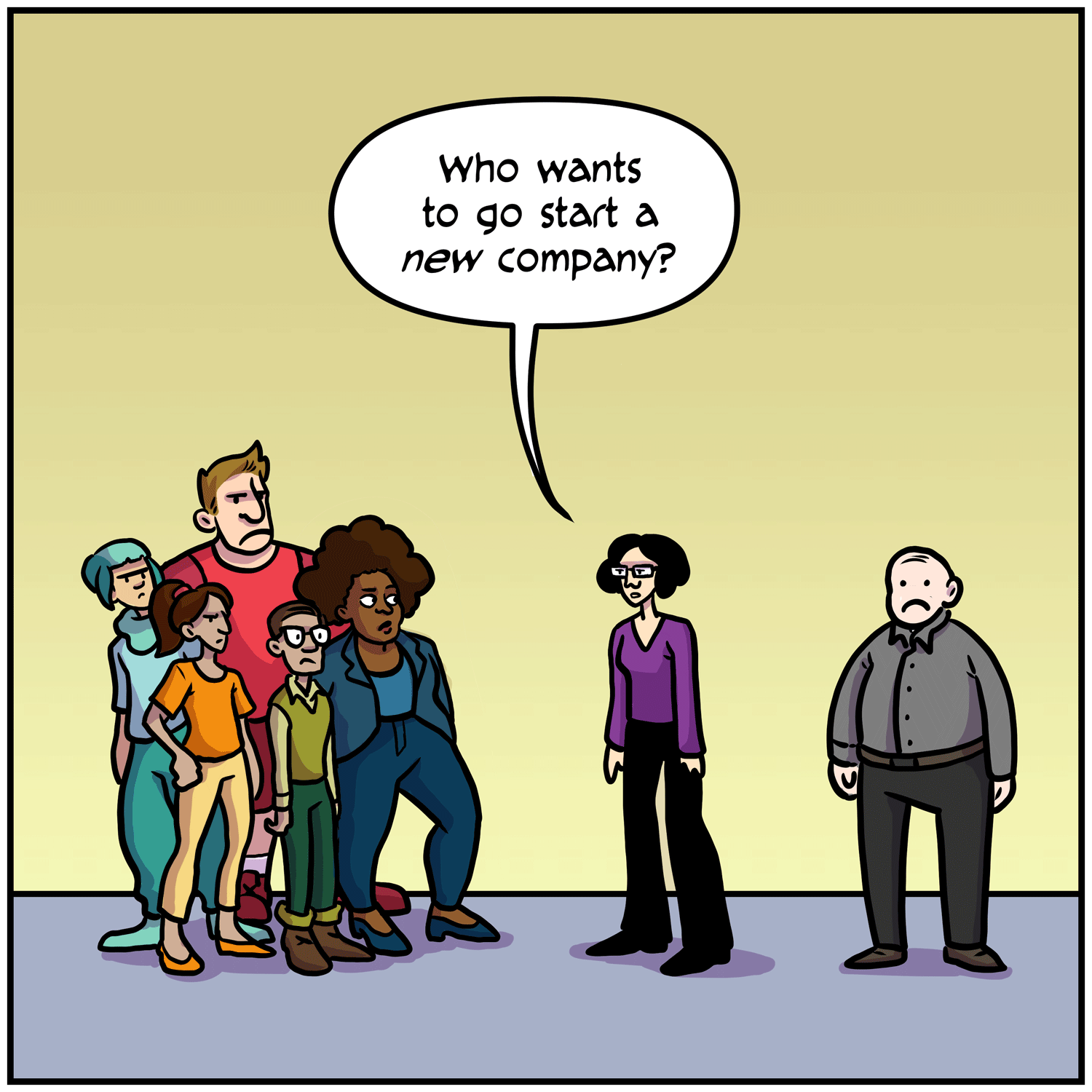
Martha turns to the interns and says “Who wants to go start a new company?”
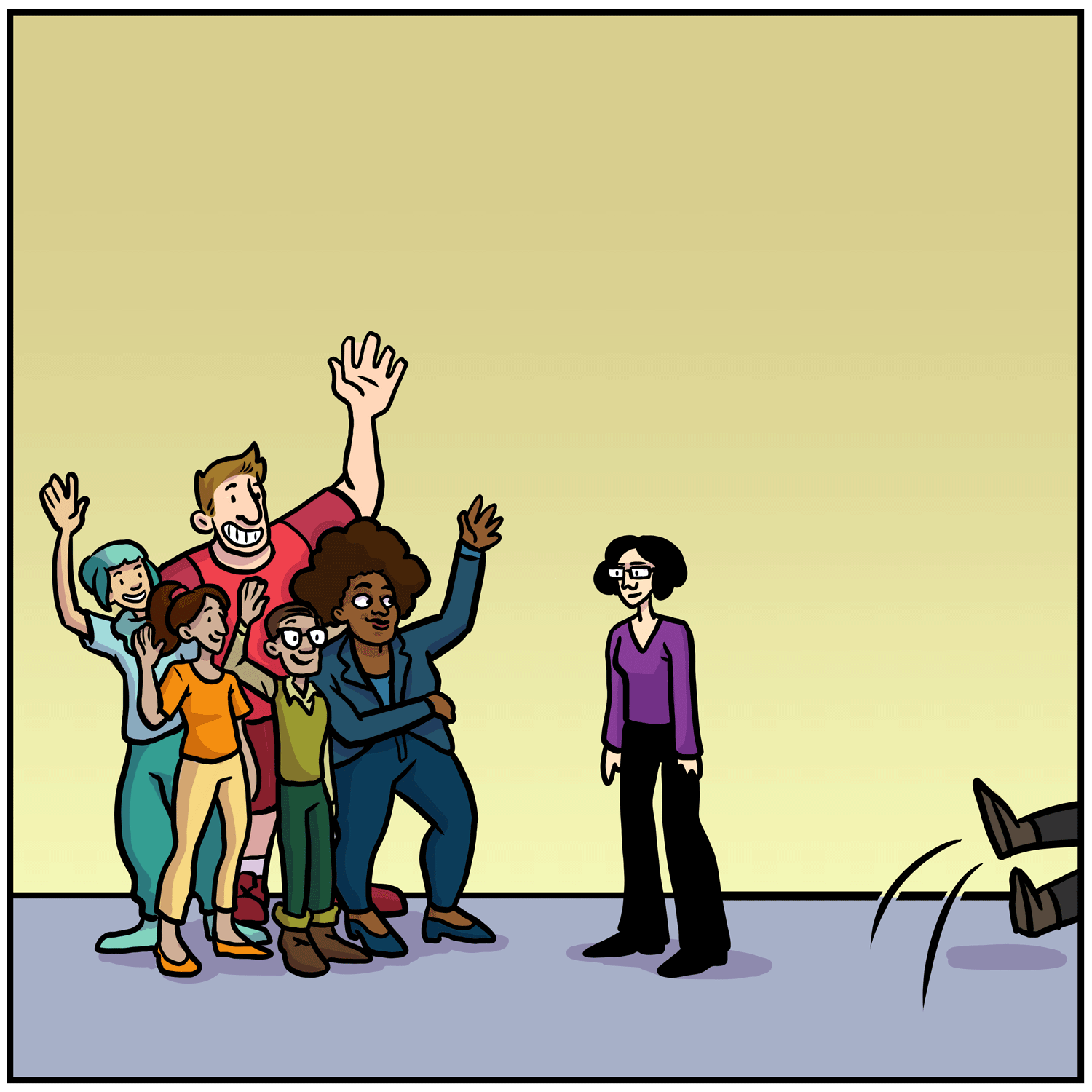
The interns immediately raise their hands and grin. Martha looks pleased. The Boss keels over.
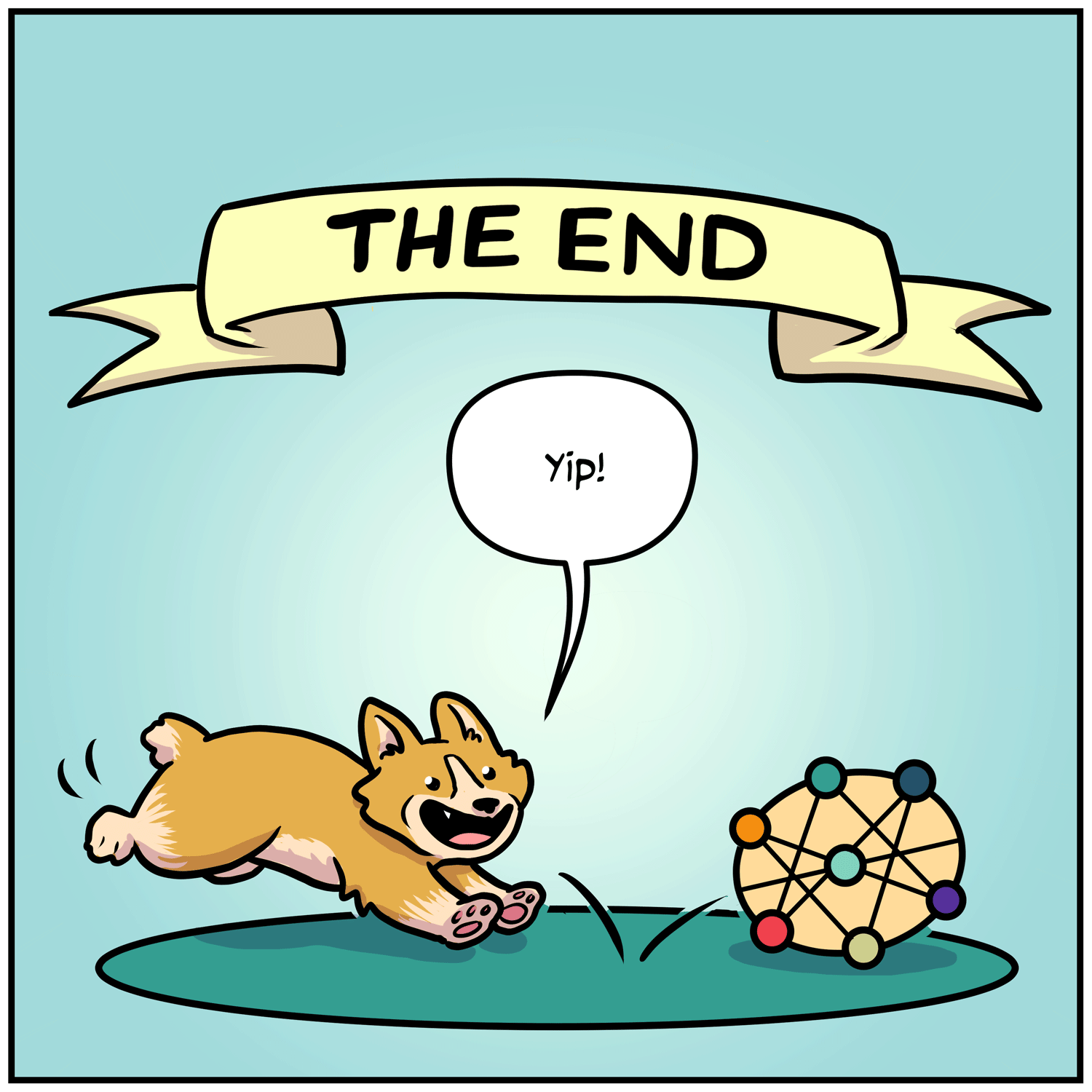
The End! A corgi chases a machine learning model across the panel, saying “Yip!”
Story by Lucy Bellwood and Scott McCloud. Art by Lucy Bellwood. This comic is licensed under the Creative Commons Attribution-Noncommercial-NoDerivative Works 3.0 license.
Translation is permitted.
About
This site is brought to you by the federated learning and analytics team at Google Research. We are a team of researchers and engineers that develop foundational technologies that enable strong privacy guarantees for AI and analytics systems. We are inventors in this space, with work spanning research and deployment of federated learning, analytics, private aggregation, differential privacy, and more. We aim to invent and deploy technologies for a private-by-default ecosystem that empowers users with transparent and verifiable privacy claims.
We believe that strong privacy guarantees are essential to the future of tech, and are committed to driving innovation in this space. We maintain a collection of open source projects under Parfait.
Questions, feedback, ideas? We’d love to hear from you: federated-feedback@google.com
Learn more
Introduction to Federated Learning and Federated Analytics
- Federated Learning: Collaborative Machine Learning without Centralized Training Data
- Federated Analytics: Collaborative Data Science without Data Collection
- How Federated Learning Protects Privacy: Interactive Introduction to Federated Learning
- Building Privacy-Preserving Systems for Machine Learning and Data Science on Decentralized Data
- (Google I/O'19) Federated Learning: Machine Learning on Decentralized Data
Use Cases in Google Products
- Federated Learning with Formal Differential Privacy Guarantee in Gboard
- Predicting Text Selections within Federated Learning
- Federated Learning in Android Private Compute Core
- Google Uses Federated Learning to Improve Speech Models
- How Messages Improves Suggestions with Federated Technology
TensorFlow Federated
Research
Best Practices, Challenges and Open Problems
- Advances and Open Problems in Federated Learning
- Field Guide to Federated Optimization
- Motley: Benchmarking Heterogeneity and Personalization in Federated Learning
YouTube Playlists of Workshop for Federated Learning and Analytics
- 2022 Workshop for Federated Learning and Analytics
- 2021 Workshop for Federated Learning and Analytics
- 2020 Workshop for Federated Learning and Analytics
Federated Differential Privacy Research
- Federated Heavy Hitters Discovery with Differential Privacy
- Practical and Private (Deep) Learning without Sampling or Shuffling
- Improved Differential Privacy for SGD via Optimal Private Linear Operators on Adaptive Streams
- Towards Sparse Federated Analytics: Location Heatmaps under Distributed Differential Privacy with Secure Aggregation
- The Distributed Discrete Gaussian Mechanism for Federated Learning with Secure Aggregation
- The Fundamental Price of Secure Aggregation in Differentially Private Federated Learning
Federated Learning Research in Gboard
- Federated Learning of Out-Of-Vocabulary Words
- Applied Federated Learning: Improving Google Keyboard Query Suggestions
- Federated Learning for Mobile Keyboard Prediction
Fundamental Research in Federated Learning
- Communication-Efficient Learning of Deep Networks from Decentralized Data
- Adaptive Federated Optimization
- Federated Select: A Primitive for Communication and Memory-Efficient Federated Learning
- Mixed Federated Learning: Joint Decentralized and Centralized Learning
Secure Aggregation
- Practical Secure Aggregation for Privacy Preserving Machine Learning
- Secure Single-Server Aggregation with (Poly)Logarithmic Overhead
Federated Learning System Design